Introduction
Departamento Moreno in the province of Santiago del Estero, Argentina (Figure 1), has as its main activity extensive cattle breeding, being the natural vegetation (Chaco Forest and savannahs) the basis of its diet (Boletta et al., 2006). The vegetation is a mosaic of wooded areas and land, cleared and sown to improved pasture. Excessive felling of the forest, overgrazing and changes in the fire regimen degraded the forest and changed it into either area covered by dense and thorny shrubs or secondary forests showing high density of individuals (Boletta et al., 2006).
According to Kunst et al. (2012, 2013) and Anriquez et al. (2005), this kind of upper stratum cover and structure significantly reduced herbaceous forage on offer and dramatically limited accessibility to animals and thus livestock activity. Livestock production in Santiago del Estero was intensified following removal of shrubs and tree strata less than 3 m tall from the Chaco forest by roller chopping and sowing megathermic grasses, such as Megathyrsus maximus (Jacq.) B.K. Simon & S.W.L. Jacobs (syn. Panicum maximum Jacq.) (Figure 2).
Determining the aboveground net primary productivity (ANPP) of the green vegetation in cattle raising activities is fundamental to take decisions, specifically concerning areas assignment and grazing lands and livestock management. In this sense, the spectral information supplied by remote sensing may provide a rapid and inexpensive means of estimating forage biomass and quality variables (Baldassini, 2018; Baldassini & Paruelo, 2020).
Productivity of vegetation derived from vegetation index
A widely-used indicator for deriving vegetation productivity from remotely sensed imagery is the Normalized Difference Vegetation Index (NDVI) (Rouse et al., 1973). The significant relationships existing between the NDVI and the fraction of the photosynthetically active radiation absorbed make it possible to determine the aboveground net primary productivity (ANPP) from data derived from NDVI time series (Baldassini, 2018; Zhang et al., 2018; Baldassini & Paruelo, 2020). The ANPP is the temporal integration of the positive increase of the biomass of vegetation photosynthetically active, expressed as the amount of dry matter produced by vegetation per unit area, it is one of the most integrative descriptors of ecosystem functioning (Myneni & Williams, 1994; Dardel et al., 2014; Baldassini, 2018; Zhang et al., 2018; Baldassini & Paruelo, 2020; Zhang et al., 2020). It was created from the Monteith’s empirical model (Monteith, 1972), based upon radiation use efficiency which is a useful tool to quantify seasonal biomass production without limitations of water, temperature and fertility.
Growing season and seasonal rainfall
Photosynthetically active vegetation delimits the growing season (Field et al., 1995) and is closely linked to the amount and distribution of seasonal rainfall (Robinson et al., 2013; Dardel et al., 2014; Zhang et al., 2018; Zhang et al., 2020; Le Houérou et al., 1988) and to the moisture available in soil (Noy-Meir, 1973), particularly in arid and semi-arid zones (Le Le Houérou et al., 1988). Le Houérou et al. (1988) reviewed pasture production studies from numerous semi-arid ecosystems to estimate the efficiency with which rainfall is converted into plant production. The productivity of grasslands is linearly related to annual precipitation in both wet and dry periods (Le Houérou, 1984; Lauenroth & Sala, 1992), and varies among different ecosystems (Le Houérou, 1984; Lauenroth & Sala, 1992). In water-limited regions, like the study area, seasonal rainfall generally explained ANPP better than total rainfall (Bai et al., 2008; Hsu et al., 2012; Robinson et al., 2013; Liu et al., 2020).
Rain Use Efficiency (RUE), defined as the ratio between ANPP and rainfall, is increasingly used to diagnose land degradation (Huxman et al., 2004; Dardel et al., 2014; Gamoun, 2016; Zhang et al., 2020; Liu et al., 2020). It has also been increasingly used to analyze the variability of vegetation production in arid and semi-arid biomes where rainfall is a major limiting factor for plant growth (Huxman et al., 2004; Bai et al., 2008; Vermeire et al., 2009). RUE is a useful ecological parameter to determine ecosystem adaptation to climate change and characterizes the efficiency of converting CO2 into dry biomass by using water (Le Houérou, 1984).
The importance of knowing and understanding the response in aboveground net primary productivity (ANPP) of M. maximus pastoral systems and its relationship with precipitation is the main determinant of forage availability and hence of stocking density. Available information, at site level, facilitates decision making on management guidelines prior to climatic adversities, such as the planning and integration of strategic forage reserves or adjustments needed to optimize grazing pressures.
From the above, the objectives of this work are: a) to delimit the growing seasons (seasonality), b) to quantify the above-ground net primary productivity and rainfall use efficiency and their responses to seasonal rainfall anomalies, and c) to relate rainfall use efficiency with seasonal rainfall and determine its trend in the analyzed period.
Materials and methods
Description of Departamento Moreno
Departamento Moreno (Figure 1, left) is located in the east center of the province of Santiago del Estero, Argentina, between 26º 53' and 27º 48' parallels, South latitude and 61º 50' and 63º 25' meridians, West longitude. The soil belongs to the order of the mollisols, represented by the group of the Haplustolls, with predominance of entic haplustolls that have been generated from original loess, under semi-arid hyperthermic climate conditions, and a plain gently undulating landscape (Boletta, 2001). According to Boletta et al. (2006), the climate is warm with an average temperature of 28 °C (centigrade) in the hottest month (January) and 16.3 °C in the coldest (July). The absolute maximum temperature exceeds 47°C and the absolute minimum is -10 °C. The rainy season extends from December with 103.3 mm average to March with 96.6 mm average. The average annual precipitation is between 500 and 750 mm. The soil water balance shows water deficit in several months, particularly in early Spring.
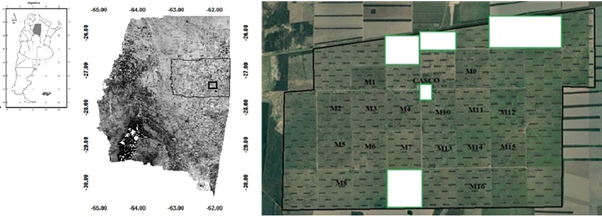
Source: https://eos.com/landviewer & Google Earth pro.
Figure 1 Left: Province of Santiago del Estero in Argentina, province of Santiago del Estero (center), Departamento Moreno (polygon in black fine line) and El 14 establishment (polygon thick black line). Right: El 14 and sixteen sample points in Megathyrsus maximus (Jacq.) pastoral systems.
The predominant natural vegetation in the province of Santiago del Estero is the semi-arid, xerophytic and open Chaco Forest (Boletta et al., 2006). According to SAyDS (2005), the climax community or upper stratum is formed by the forest of Schinopsis quebracho colorado and Aspidosperma quebracho blanco; the middle stratum by of species of the Zizyphus, Cercidium, Celtis, Caesalpinia and Prosopis genuses, among others; the shrub stratum by species belonging to the Acacia, Mimosa, Geoffroea, Prosopis, Atamisquea genuses, among others; and the herbaceous stratum is formed by grasses of the Setaria, Gouinia, Digitaria, Eragrostis, Gymnopogon, Panicum, Heteropogon, Trichloris, Chloris, and dicotyledons such as Ruellia, Justicia, Holocheilus, Trixis, Hyptis genuses, among others.
Description of the study area
The study area is El 14 establishment (Lat -27.6683/Long -62.2853), the owner company is RUAS Agropecuaria SA, located in Quimili, Departamento Moreno, province of Santiago del Estero (Figure 1, right). El 14 has an area of 7500 ha, with paddocks of 200 ha. The soil is loamy, with high content of organic matter ranging between 3% and 4%, soils are deep and well drained, without phreatic, and the phosphorus content is higher than 50 ppm, rich in potassium and nitrogen. The main activity is extensive livestock breeding, with 5000 adult wombs, services in December, January and February, and deliveries in September, October and November. All the wombs and bulls are of San Ignacio breed, created at the Catholic University of Córdoba (data provided by the managers).
MODIS NDVI Time Series
The time series of the Normalized Difference Vegetation Index (NDVI) (Rouse et al., 1973) was derived from the Temporary Vegetation Analysis System (Embrapa, 2021). The system provides temporary NDVI profiles (derived from MOD13Q1 (Terra satellite) and MYD13Q1 (Aqua satellite) images belonging to the LP-DAAC/EOS-NASA project, with Google Maps interface. MODIS NDVI, produced on 16-day intervals and at spatial resolution 250 m x 250 m, derived from atmospherically-corrected reflectance in the red, near-infrared, and blue wavebands.
Sixteen sampling points (M1 to M16) were located, twelve samples were taken in central paddocks and four in peripheral paddocks (Figure 1 right). Each sampling polygon covers four pixels of NDVI MODIS250, covering 21.6 pure hectares of M. maximus pastoral systems throughout the period analyzed. The Lat/Long coordinates of the sixteen sampling points are shown in Table 1 and Figure 1 (right).
Sixteen time series of original MODIS NDVI were derived, one for each sampling point, each time series ranged from 08.12.2000 to 05.25.2021 consisting of a total of 912 NDVI values for each sampling point. According to Celleri et al. (2018) and Gao et al. (2020) land surface monitoring over long timescales is essential to identify ecosystem responses to climate variability. Remote sensing allows gathering information over large geographic areas with high revisit frequency and low cost, providing a valuable tool for long-term observation of land surface processes.
Table 1 Lat/Long coordinates of the sixteen sampling points
M1: Lat-27.6515/Long-62.3059 |
M2: Lat-27.6626/Long-62.3198 |
M3: Lat-27.6635/Long-62.3069 |
M4: Lat-27.6627/Long-62.2926 |
M5: Lat-62.3201/Long-62.2924 |
M6: Lat-27.6754/Long-62.3066 |
M7: Lat-27.6757/Long-62.2929 |
M8: Lat-27.6907/Long-62.3195 |
M9: Lat-27.6489/Long-62.2652 |
M10: Lat-27.6641/Long-62.2791 |
M11: Lat-27.6622/Long-62.2654 |
M12: Lat-27.6624/Long-62.2513 |
M13: Lat-27.6767/Long-62.2796 |
M14: Lat-27.6765/Long-62.2654 |
M15: Lat-27.6758/Long-62.2505 |
M16: Lat-27.6902/Long-62.2655 |
Growing seasons of pastoral systems
The growing seasons of pastoral systems were delimited by transforming the original NDVIMODIS time series into NDVIRATIO time series; the method was developed by White et al. (1997) and was performed using Formula 1.
The NDVIRATIO oscillates in the range of zero to one. In Formula 1, NDVI is the 16 day NDVIMODIS, NDVIMAX is the maximum 16 day NDVIMODIS and NDVIMIN is the minimum 16 day NDVIMODIS. The beginning and end of each biannual growing season was determined by the 0.5 threshold NDVIRATIO (White et al., 1997). According to White et al. (1997) the transformation is attractive because it is consistent. Thus, a single NDVIRATIO threshold may be used, obviating the need to establish absolute NDVI thresholds or landcover-specific thresholds (White et al., 1997). According to Myneni and Williams (1994), the NDVIRATIO 0.50 threshold minimizes background effects on fAPAR/NDVIRATIO. However, while Gao et al. (2020) report that remote sensing thresholds are currently still a challenge, the NDVIRATIO 0.5 threshold used in this study reduces uncertainty in delimiting growing seasons, and thus increases accuracy in quantifying seasonal ANPP of Chaco Semi-arid vegetation at local and regional level.
Aboveground net primary productivity
Aboveground net primary productivity (ANPP) of the pastoral systems was determined by the radiation use efficiency model proposed by Monteith (1972). The model establishes that the ANPP of a plant cover is proportional to the incident photosynthetically active radiation (PAR), that is, the fraction of that radiation that is intercepted by green tissues (fAPAR), and to the conversion efficiency (ε). According to Fensholt (2004), due to the strong relationship between NDVI and fAPAR, the Monteith model can be written as follows (Formula 2):
In Formula 2, the efficient use of the energy ε = 0.94 g·dm·Mj-1·m-2 is the average of the four stages of the growing season (Spring, Summer, late Summer and Autumn) of M. maximus var. Gatton pastures (Baldassini & Paruelo, 2020). PAR (Mj/m2.t) is the incident photosynthetically active radiation. SINDVIRATIO is the NDVIRATIO growing season integrated, defined by the area under the curve delimited by start and end of the season, was determined mean Formula 3 (Zhang et al., 2018; Zhang et al., 2020).
In Formula 3, i is NDVIRATIO monthly of the growing season j.
The fraction of photosynthetically active radiation (fAPAR) absorbed by the pastoral systems was determined by Formula 4 (Myneni & Williams, 1994; Seaquist et al., 2003). The parameters α = 0.504 and β = 0.01 are the constants that arise from the linear relationship between NDVIRATIO Maximum (0.999) and NDVIRATIO Minimum (0.50) of the 2000-2021 time series (Myneni & Williams, 1994; Seaquist et al., 2003; Fensholt, 2004).
The average seasonal incident radiation in the area under study (average of 22 years) was derived from the Prediction of Worldwide Energy Resource (POWER) Project (NASA, 2021). Incident photosynthetically active radiation (PAR) in foliage is considered to be a constant fraction of 48% of the incident radiation at the atmosphere limit (Seaquist et al., 2003; Fensholt, 2004). The ANPP of pastoral systems was quantified and expressed in kg·dm·ha-1.
Time series of rainfall data
Time series of average monthly rainfall corresponding to period 2000-2021 were derived from the weather station at “Sociedad Rural del Noreste Santiagueño” (SRNS, Coor: lat -27.65; long -62.40). This weather station is 12 km away from the study area. Average monthly rainfall produced in the months corresponding to growing seasons were integrated to generate the seasonal rainfall (SR) for each of the 21 growing seasons.
The anomalies of the seasonal rainfall (Figure 3) were determined as follows the Formula 5.
Where Xi is the integrated seasonal rainfall (SR) of each of the 21 growing seasons included in the period 2000-2021, and Xh is the historical integrated seasonal rainfall (1918-2021).
Rain use efficiency of pastoral systems
Rain use efficiency (RUE) could be a critical indicator for evaluating the response of primary productivity to variability of rainfall in arid and semi-arid ecosystems (Le Houérou et al., 1988; Sala et al., 1988). The RUE can be calculated directly as the ratio of ANPP to the corresponding integrated seasonal rainfall by the Formula 6 (Bai et al., 2008; Hu et al., 2010; Zhang et al., 2020).
Where RUE is the rainfall use efficiency (kg·dm·ha−1·mm−1), ANPP is the aboveground net primary productivity of growing seasons (kg·dm·ha-1·season−1), and RS is the integrated seasonal rainfall (mm) in the pastoral systems
Statistical analysis
The mean of the aboveground net primary productivity of growing seasons (kg·dm·ha-1·season−1) (expectations) were compared, using the t-test for independent samples (Di Rienzo et al., 2019). The classification variables (distributions) were two periods: One with negative rainfall anomalies (AN), which including the growing seasons: 2000-2001, 2003-2004, 2005-2006, 2007-2008, 2008-2009, 2011-2012, 2012-2013, 2014-2015, 2017-2018, and another with positive rainfall anomalies (AP) which including growing seasons: 2002-2003, 2004-2005, 2006-2007, 2009-2010, 2010-2011, 2013-2014, 2015-2016, 2016-2017, 2018-2019, 2019-2020, 2020-2021 (Figure 3).
Analysis trend of seasonal RUE was performed using a linear best-fit regression line fitted to RUE of growing season of the period 2000-2021. The slope of the resulting best-fit line was tested using a t-test for a parameter to see if it was significantly different (p < 0.01) from a zero slope (Di Rienzo et al., 2019).
The RUE (kg·dm·ha−1·mm−1) (dependent variable y) by means of the linear or nonlinear regression method (α = 0.05) were related to integrated season rainfall (SR) (independent variable x). The models were based on two goodness-of-fit tests: 1) the best adjustment of the adjusted coefficient of determination (R2 Aj) and by the lack of fit test which is an estimation regardless (2 of the model or pure error (Di Rienzo et al., 2019).
The analyses were performed with the Infostat package (Di Rienzo et al., 2019). Only the relationships found statistically significant (p < 0.05) will be displayed.
Results and discussion
Growing season of pastoral systems
The NDVI MODIS time series and the 0.5 NDVIRATIO threshold make it possible to delimit the start and end of the growing stations for the pastoral systems with high efficiency. The growing seasons start in November and end May. Figure 4 show the NDVIRATIO growing season integrated (SINDVIRATIO) (from November to May) corresponding to period 2000-2021.
ANPP-Seasonal of pastoral systems
Significant differences were found between ANPP-Season of growing season with anomalies rainfall positive and anomalies rainfall negative (Table 2).
The growing seasons included in group A with seasonal positive rainfall anomalies or positive legacies (Sala et al., 2012) had an ANPP-seasonal mean of 18 729.29 kg·dm·ha-1, minimum of 6 584 kg·dm·ha-1 and maximum of 24 431 kg·dm·ha-1.
The growing seasons included in group B with seasonal negative rainfall anomalies or negative legacies (Sala et al., 2012) had an ANPP-seasonal mean of 16 233.78 kg·dm·ha-1, minimum of 5 238 kg·dm·ha-1 and maximum of 23 319 kg·dm·ha-1.
According to Celleri et al. (2018) in arid and semi-arid regions, characterized by water scarcity, vegetation is highly sensitive to the quantity, timing and frequency of rainfall events. However, the productivity of the pastoral systems groups recovers itself rapidly in the respective post-drought seasons, like growing seasons 2009-2010, 2013-2014, 2016-2017, 2018-2019 and 2020-2021 (Figure 3). On this respect, Sala & Lauenroth (1982) communicated that drought lowers the ANPP; however, this can recover itself rapidly after high intensity precipitations. Hoover et al. (2014) observed a total recovery of the ecosystemic functions of the pastures one year after the drought due to the rapid response of the predominating C4 species. According to Hsu et al. (2012) little changes in the average precipitation might cause great changes in the ANPP of some pastures in arid and semiarid zones.
Table 2 T-test for sample independents of ANPP-Season of growing season of pastoral systems corresponding to period 2000-2021. AN = seasonal negative rainfall anomalies, AP = positive rainfall anomalies, T = T-test statistic and p-valor = probability.
Classific | Variable | Grup A | Grup B | n(A) | n(B) | Mean(A) | Mean(B) | T | p-value |
---|---|---|---|---|---|---|---|---|---|
Anomalies | ANPP | (AP) | (AN) | 176 | 160 | 18 729.29 | 16 233.78 | -6.3 | < 0.0001 |
The results show the high sensitivity of the ANPP-Season of pastoral systems to the increase or decrease in seasonal rainfall. They represent the response of production per unit increase in resource input. The M. maximus pastoral systems make it apparent their high capacity of resilience before such extreme climatic events such as droughts.
The results are consistent and coincide with those obtained in previous work carried in the semi-arid Chaco region. On this matter, Baldassini (2018), Tiedemann (2018) and Baldassini & Paruelo (2020) determined the ANPP of pastoral systems of M. maximus in the Semiarid Chaco mean time series of NDVI MODIS and the Monteith model. Baldassini (2018) found significant differences in the average annual ANPP of pure pastoral systems of M. maximus in the Semiarid Chaco of Salta throughout a precipitation gradient. The ANPP with rainfall ≤ 650 mm was 5 649.21 kg·dm·ha-1 while with rainfall ≥ 700 mm was 6 038.71 kg·dm·ha-1. Later, Baldassini & Paruelo (2020) determined, in the Semiarid Chaco of Salta, yields ranging from 4 300 kg·dm·ha-1 and 11 700 kg·dm·ha-1. In turn, Tiedemann (2018) determined that ANPP seasonal mean of M. maximus pastoral systems in Departamento Moreno during the period 2008-2016. The author determined that ANPP seasonal was affected by positive and negative anomalies of seasonal rainfall. The ANPP of growing seasons affected by negative rainfall anomalies oscillated between 1 790.2 kg·dm·ha-1 and 6 440.25 kg·dm·ha-1, while the ANPP of growing seasons with positive rainfall anomalies oscillated between 9 245.23 kg·dm·ha-1 and 11 562.31 kg·dm·ha-1.
Rain use efficiency, seasonal trend and seasonal rainfall relationship.
The RUE means of the period 2000-2021 was 29.45 kg·dm·ha-1·mm-1, the maximum 56 kg·dm·ha-1·mm-1 and the minimum 17 kg·dm·ha-1·mm-1 (Figure 5).
Seasonal slope of the RUE (-0.88) was significantly different from zero (T = 14.16; p < 0.000 1) (Figure 5), evidencing a marked negative trend in the period and a high sensitivity of pastoral systems to variation in seasonal rainfall anomalies.
Significant and nonlinear relationships were found between rain use efficiency (RUE) of pastoral systems and seasonal rainfall (SR) (R² = 0.61; R²Aj = 0.56; F = 13.83; p < 0.000 1) (Figure 6, Formula 7). The model did not show a lack of fit.
According to Gamoun (2016) the RUE is also highly dependent on soil and vegetation type and environmental conditions and, therefore, may not necessarily respond linearly with rainfall. Further, Hsu et al. (2012) state that, in many cases, ANPP during relatively wet years determines the nonlinearity of precipitation-ANPP relationship, and the frequency of these ‘outlier’ years is increasing with climate change.
The growing season of pastoral systems with the maximum RUE had rainfall of 300 mm (Figure 6), evidencing the great adaptation of the Megathyrsus pastoral systems to the negative rainfall anomalies (Huxman et al., 2004). According to Huxman et al. (2004), during the driest years at each site, there is convergence to a common maximum RUE that is typical of arid ecosystems in years when water is most limiting, deserts, grasslands and forests all exhibit the same rate of biomass production per unit rainfall, despite differences in physiognomy and site-level RUE ecosystems.
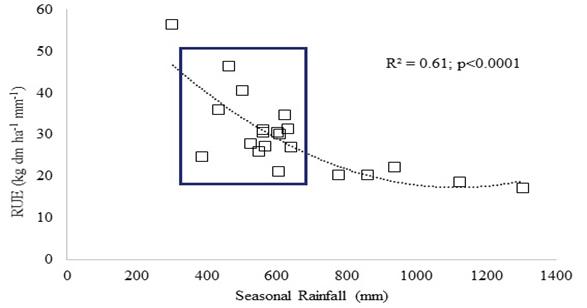
Figure 6 Rain use efficiency of Megathyrsus maximus pastoral systems and seasonal rainfall relationship corresponding to period 2000-2021.
The RUE of fifteen growing stations (Figure 6, blue box) had seasonal rainfall ranging from 400 mm to 650 mm (normal seasonal rainfall for the study area). According to Hsu et al. (2012) and Zhang et al. (2018), ANPP sensitivity to rainfall average is higher in semi-arid ecosystems that get between 300 m and 600 mm year-1 rainfall. In accordance with Huxman et al. (2004), at the sites with lowest annual average rainfall, high efficiency of water use associated with individual plant growth rate is translated to high efficiency of water use at the ecosystem level. In turn, Le Houérou (1984) states that the high level of synchronization between biomass productivity and the positive and negative trends of precipitations is due to the wide adaptation of plants to water-limited environments.
The growing seasons that had rainfall ≥ 700 mm (with positive rainfall anomalies) are the ones that had the lowest RUE, which ranged between 17 and 20 kg·dm·ha-1·mm-1 (Figure 6). Following Paruelo et al. (1999), the model using only the average annual precipitation can explain such a big fraction of the spatial variability of the ANPP, from the desertic prairies to the mesic ones. They showed that RUE increased first, peaked at 475 mm·yr-1, and then declined along a precipitation gradient (200 - 1 200 mm·yr-1). For a given ecosystem, a number of studies such as those carried out by Huxman et al. (2004), Bai et al (2008), Dardel et al., (2014), and Gamoun (2016), have shown that RUE decreases over time with increasing annual precipitation.
The widely accepted view is that RUE should increase with annual rainfall until other environmental factors limit ANPP (Le Houérou, 1984). They are major determinants of soil water availability, consequently, large amounts of precipitation are not utilized for plant growth, and have important effects on the site-level RUE (Noy-Meir, 1973; Le Houérou, 1984; Sala et al., 1988). Moreover, increases in annual rainfall amounts usually reduce RUE due to runoff and drainage, soil evaporation, soil infertility, water-holding capacity, permeability, texture and depth and temperature increase (Paruelo et al., 1999; Bai et al., 2008; Gamoun, 2016; Zhang et al., 2018; Zhang et al., 2020; Liu et al., 2020), leaf area index and vegetation cover (Hu et al., 2010), plant species composition, seral stage, basal cover, and previous year production (Vermeire et al., 2009)
Previous studies carried out in Departamento Moreno make it possible to infer the causes of the ''ineffective precipitation'' (Noy-Meir, 1973) of the growing seasons with seasonal rainfall equal to or greater than 700 mm. Further, this value could be considered the threshold in the inefficient use of water of pastoral systems in the area study.
According to Le Houérou (1984) anthropic activities would be one of the main causes of the inefficient use of water by surface runoff. In the study area anthropic activities like deforestation at clear-cut and management of livestock, would contribute to water loss by runoff in pastoral systems. The elimination of the shrub and tree stratum of the Chaco forest by roller chopping of different intensities and changes in the fire regime influenced the action of wind and surface runoff generating wind and water erosion processes (Anriquez et al. (2005); Boletta et al., 2006; Kunst et al., 2012, 2013). Overgrazing due to inadequate management of livestock densities or to droughts contributed to soil compaction, which prevents the infiltration of precipitation water, modifies its storage capacity, and increases surface runoff (Anriquez et al. (2005); Boletta, 2001, Kunst et al., 2012, 2013). Increases of unproductive water loss by runoff and high bare soil evaporation on grazed sites have been viewed as the most important reasons for low RUE (Le Houérou, 1984; Bai et al., 2008, Hu et al., 2010). Le Houérou (1984) reviewed the fact that the magnitude of evaporation from soil surface in arid and semi-arid rangelands varies from 20% to 70% of the infiltrated rain. Furthermore, the soil environment is harsh due to serious soil erosion, causing considerable losses of N, P and other soil nutrients (Bai et al., 2008; Gamoun, 2016; Zhang et al., 2020). ANPP in arid and semi-arid ecosystems is usually limited or co-limited by nitrogen availability, which is tightly coupled with water availability through biogeochemical feedbacks (Huxman et al., 2004).
Furthermore, according Huxman et al. (2004), Vermeire et al. (2009), Robinson et al. (2013), Liu et al. (2020), Celleri et al. (2018) and Zhang et al. (2018), shifts in seasonal storm in intensity/magnitude, number of rainy, timing and frequency, which may independently affect communities, will make it more important than ever to factor in how abiotic and biotic factors affect the transition from precipitation to plant-available soil moisture. All of these factors would greatly contribute to the loss of large amounts of water by surface runoff in the study area. In this sense, Figure 3 shows extreme seasonal positive rainfall anomalies of 500 mm and 700 mm. Further, in the period analyzed, average rainfall was recorded in the wettest quarter of great intensity/magnitude: December 127 mm ± 79, January 135 mm ± 80 and February 103 mm ± 78.
Conclusions
The NDVIMODIS time series and the 0.5 NDVIRATIO threshold make it possible to delimit the growth season and quantify the seasonal ANPP of pastoral systems with high efficiency in the study area. Information derived from time series is essential for understanding the temporal dynamics and productive capacity of pastoral systems.
The seasonal ANPP of Megathyrsus maximus (Jacq.) B.K. Simon & S.W.L. pastoral systems of the study area shows great sensitivity to seasonal rainfall anomalies. The ANPP seasonal of the pastoral systems recovers itself rapidly of negative rainfall anomalies in the post-seasons with positive rainfall anomalies. The M. maximus pastoral systems make it apparent their high capacity of resilience before such extreme climatic events as droughts.
Most of growing season RUE are related to the normal range of precipitation for the study area evidencing great adaptation of pastoral systems. In turn, growing season with lowest RUE were related to rainfall ≥ 700 mm. Seasonal rainfall of 700 mm could be considered the threshold in the loss of large amounts of water of pastoral systems in the area study. Anthropic activities like deforestation and management of livestock, and shifts in seasonal storm in intensity/magnitude, number of rainy, timing and frequency, they would contribute to the loss of large amounts of water by surface runoff in the study area.
Changes in RUE have been suggested as an integral measure for evaluating pastoral system state, like land degradation, runoff coefficient and fertility in the study area.
The regression models obtained provide novel information at the local level and are useful and efficient tools for predicting the productivity of M. maximus. Jacobs pastoral systems in terms of seasonal rainfall. These make it possible to implement appropriate management strategies (such as reserves) to mitigate extreme climatic adversities especially in arid and semiarid lands like the study area.