Introduction
The new ICD-11 dimensional model for the diagnosis of personality disorder
Personality refers to the characteristic way in which individuals behave; experience life; and perceive and interpret themselves, other people, events, and situations. On the other hand, personality disorder (PD), according to the new ICD-11 model, which is ready to be implemented from the year 2022 (Bach et al., 2022; Mulder, 2021), is a substantial and marked alteration in personal and social functioning (World Health Organization (WHO) 2022a, 2022b).
In the ICD-11, the diagnosis of PD has taken a radical turn concerning the previous nosological model, since it represented a diffuse conceptualization causing problems in the theoretical, practical, and methodological aspects (Bach et al., 2022; Mulder, 2021; Tyrer et al., 2019; Widiger & Oltmanns, 2021), which present in five important deficits. (a) The most frequent diagnoses corresponded to two of the 10 specific diagnostic categories, borderline personality disorder (BPD), antisocial personality disorder (ASPD), and personality disorders not otherwise specified (PD NOS). (b) In addition, the complexity of the nosology of PD meant that any interest was limited to a few specialists, and inexperienced general practitioners or psychologists avoided getting involved (Tyrer et al., 2019; Watts, 2019 ). (c) Likewise, a strict categorical PD model could not explain the relationship between abnormal and normal personalities. (d) Moreover, there is consistent evidence that severity, rather than specification of personality pathology, is the main predictor of individual suffering and dysfunction (Tyrer et al., 2019). (e) Furthermore, the constant overlap (comorbidity) of specific personality types added to the categorical diagnostic criterion caused deficits in the construct and diagnostic validity of the taxonomic model criteria of the previous classification system.
The diagnosis of PD in the ICD-11 is mainly defined according to its severity with the categories «mild»,
«moderate,» or «severe» (Mulder, 2021; WHO, 2022a, 2022b) -located in Chapter 06: Mental, behavioral and neurodevelopmental disorders-, and the category «difficulty» (included in Chapter 24: Factors influencing health status or contact with health services). Optionally, personality can be classified according to five trait domains: (a) negative affectivity, (b) detachment, (c) dissociality, (d) disinhibition, (e) anankastia, and the additional qualifier borderline pattern (previously called BPD). With this new diagnostic model, from a theoretical point of view, the conceptualization of psychopathology, the understanding of semiology (Bach et al., 2022), and the epigenetics of PD (Ramoz, 2022) are improved; and from a methodological point of view, it allows the development of new procedures, instruments, and evaluation and intervention devices with multidisciplinary work that the new times demand (see, e.g., Christensen et al., 2020.
From a practical point of view, according to Bach et al. (2022), the communication of non-stigmatizing clinical information between mental health professionals, health service administrators, researchers, patients, and their families is favored; and the differential diagnosis and assignment of the most relevant mental disorders predicting future treatment needs (such as suicide, assault, and morbidity), and the expected degree of disability leave. This is because the main diagnosis, based on severity, allows the detection and early intervention of personality difficulty or the corresponding referral by the primary care psychologist if the anomaly exceeds the clinical threshold (Bach, 2019; Bach et al. (2022; Bach, Somma, et al., 2021; Tyrer & Mulder, 2022). Diagnosis based on optional qualifiers allows mental health specialists to focus on the type of intervention performed (Bach, 2019; Bach et al. (2022; Bach & Simonsen, 2021).
Current measures of the ICD-11 personality disorder model
Existing measures for the evaluation of PD according to ICD-11, -as also detailed in Mulder & Bach (2022)-, are based on self/hetero-informed instruments and semi-structured interviews as following (see Appendix A for more details):
(1) Psychometric studies of item pool development, based on ICD-11 Clinical Descriptions and Diagnostic Requirements (CDDR), to generate measures include: (a) for the severity of personality dysfunction, the ICD-11 Personality Disorder Severity Scale (PDS-ICD-11; Bach, Brown, et al., 2021) and two domain scales from the ICD-11 Personality Disorder Model (ICD-11 PD Model scales; Clark et al., 2021); (b) for the trait domain qualifiers, five domain scales from the ICD-11 PD Model (Clark et al., 2021) and the Personality Inventory for ICD-11 in its self-report version (PiCD; Oltmanns & Widiger, 2018) and its hetero-informed version (Informant-Personality Inventory for ICD-11 (IPiC); Oltmanns & Widiger, 2021); and finally (c) for the additional borderline pattern qualifier, the Borderline Pattern Scale (BPS; Oltmanns & Widiger, 2019).
(2) Research that consider the assembly of items from other measures to develop scales include the following: (a) for the severity of personality dysfunction, the Standardized Assessment of Severity of Personality Disorder (SASPD; Olajide et al., 2018); (b) for the trait domain qualifiers, the Personality Inventory for DSM-5-Brief Form-Plus (original) (PID-5BF+ (original); Kerber et al., 2022), the Personality Assessment Questionnaire for ICD-11 (PAQ-11; Kim et al., 2021), the Personality Inventory for DSM-5-Brief Form scored for ICD-11 (PID-5-BF for ICD-11; Bach & El Abiddine, 2020), the Five-Factor Inventory for ICD-11 (FFiCD; Oltmanns & Widiger, 2020), the Personality Inventory for DSM-5 scored for ICD-11 (revised) (PID-5 for ICD-11 (revised); Sellbom et al., 2020), the Personality Inventory for DSM-5 scored for ICD-11 (original) (PID-5 for ICD-11 (original); Bach et al., 2017), and the Personality Assessment Schedule for ICD-11 (PAS-ICD-11; Tyrer, 2017); and (c) for the additional borderline pattern qualifier, an 8-item scale based on an algorithm from other PAQ-11 scales (Kim et al., 2021).
(3) Finally, studies that consider the validation of a measure of Criterion A (level of personality functioning) in the Alternative Model of Personality Disorders of the DSM-5-TR (DSM-5 AMPD; American Psychiatric Association, 2022), taking as criterion the severity of personality dysfunction proposed in the ICD-11, include the Self and Interpersonal Functioning Scale (SIFS; Gamache et al., 2021), the Semi- Structured Interview for Personality Functioning DSM-5 (STiP 5.1; Hutsebaut et al., 2021 ; Huprich et al. 2018; Hutsebaut, et al.2016), and the Level of Personality Functioning Questionnaire Screener (LoPF-Q Screener, Goth et al., 2019 ; Zimmermann et al.,2022).
According to Morgado et al. (2017), there are 10 main limitations of test development studies: (a) sample characteristic limitations that include convenience sampling OR small sample size (< 1:10); (b) methodological limitations that include cross- sectional methodology OR self-reporting methodology OR web-based survey; (c) psychometric limitations that include lack of a more robust demonstration of the construct validity/reliability OR inadequate choice of the instruments/variables to be correlated with the variable of the study OR factor analysis errors; (d) qualitative research limitations that include lack of deductive approach to scale development OR lack of a more robust literature review OR subjective analysis OR content validity was not formally assessed OR lack of recruitment and training of a qualified number of interviewers; (e) missing data that includes the absence of enough information about grouped OR added OR rounded OR censored OR truncated numbers; (f) lack of detection of invalid responses1 that refers to not administering and measuring validity scales; (g) item limitations that include ambiguous OR not reversed items; (h) brevity of the scale that refers to include a few quantity of items if Cronbach alpha is used to test reliability; (i) difficulty to control all variables that refers to those important variables that were not considered within the construct; and finally (j) lack of manualized instructions that includes not to consider instructions on application conditions OR administration mode OR response mode OR rating mode OR giving an example.
Taking into account the above, it has been reviewed that the three main limitations for the development of the ICD-11 PD nosology measures are, first of all, sample characteristic limitations (94.1%); secondly, methodological limitations (94.1%); and, thirdly, lack of manualized instructions (88.2%). Even though cross-cultural validation and adaptation studies of these instruments carried out to date have mostly supported the applicability of the new ICD-11 PD diagnostic nosology (Ayinde & Gureje, 2021), as mentioned by Sleep et al. (2021), the current severity instruments of personality functioning show fragile factorial structures with low discriminant validity. In addition, it has been shown that all these instruments contain ordinal response formats with four or more response options, which increases the resistance and fatigue of examinees, and reduces the possibility of controlling and/or measuring their biased answers, thereby increasing systematic error (Moran, 2021). In addition, it has been noted that these instruments have been developed and/or validated mainly in European and North American populations, which have characteristics that are very different from those of underdeveloped countries. These limitations could affect the validity and replicability of the psychometric results found in different settings and in culturally diverse groups, in which their usefulness may be questioned (Sleep et al. (2021).
The need for a comprehensive measure of ICD-11 PD in correctional settings: a science- practice gap
Personality assessment for mental health purposes in prison settings has four main functions: (1) detection and diagnosis, for example, to find out if an inmate is depressed and at risk of self-harm; (2) prognosis, e.g., to predict whether inmates will present a significant risk to others once they are released; (3) case conceptualization and treatment selection, e.g., to understand the severity of the PD and its management; and (4) treatment monitoring and follow-up, e.g., to assess whether the rehabilitation program -whether group or individual- is being, or has been, effective in reducing violence risk (Day & Cook, 2019).
The evaluation of PD in prisons also relies on five legal purposes: a) competency to stand a trial, (b) criminal responsibility, (c) dangerousness, (d) pre- sentence, and (e) risk and recidivism assessments. The competency to stand a trial assessment addresses inmates’ current state of mind and whether they can understand their charges to assist their attorney in defense (Ben-Porath et al., 2022; Butcher et al., 2015). Criminal responsibility assessments address the prisoner’s mental state at the time of committing an offense with intent, recklessness or negligence (Sellbom et al., 2022 ). Dangerousness assessments are conducted on processed inmates after dismissing criminal liability but accepting a civil one; they seek to determine whether to transfer the inmate to a forensic unit (i.e., a secure environment), a civilian psychiatric unit in the community, or if outpatient treatment is necessary (Butcher et al., 2015; 3). Pre-sentence assessments are performed in inmates between the period of adjudication of guilt but before sentencing so that the judge may consider the mitigating factors when deciding on a sentence and/or incorporating mental health needs in the prison treatment plans (Ben-Porath et al., 2022). Risk and recidivism assessments are conducted in inmates to predict future violent behavior -e.g., self and hetero- aggression- and address their security needs, often aimed at prison classification and reclassification (Cirlugea et al., 2013; Mia et al., 2020; Toop et al.,2019).
Given the above, it is understandable that mental health in prison involves psycho-legal issues within criminal law, since PD, added to the current psychopathology of addiction or other issues, is a predictor of the commission of crimes, current behavior in prison, and possible recidivism of the prisoner once free. These components (moreover, the last one) are the main prognostic foci in the evaluation of the prisoner (Day & Cook, 2019). This is because the average incarcerated person has been widely shown to have a severe personality disorder, with actual and lifetime prevalence rates of PD diagnoses of 40-88% (Hutsebaut et al., 2021).
With the ICD-11 PD model, to date, only two personality measurement instruments have been validated in a few participants from prison populations (85 and 30 inmates, respectively; see Bach & Anderson, 2020; Hutsebaut et al., 2021). However, because the characteristics of this population are not considered in the design of these instruments (Meaux et al., 2021), several factors can affect the reliable evaluation of personality functioning in prison settings. Lack of morality and capacity for reflection are internal factors related to the severity and type of predominant personality pathologies, and social desirability and deception are external factors related to the specific context (Hutsebaut et al., 2021).
As some authors state (see Abdalla-Filho, 2022; Bartlett, 2011), the ICD-11 PD nosology and therefore its measures, developed from the semiology of clinical patients, do not apply to the characteristics of patients with PD who are involved in legal issues since the very present deficits and cognitive deficiencies of inmates with this diagnosis are not taken into account. Likewise, importance is not given to the comorbidity (differential diagnosis), etiology, and natural life course of the disorder if it is not treated, including the commitment to security within the penitentiary (Abdalla-Filho, 2022). Another disadvantage is that, in contrast to what Bach et al. (2022) stated and as Halliwell (2021) already warned, the ICD-11 PD nosology does not exempt the risk of stigmatization. This has just been demonstrated in the jurisprudence, in a recent study for criminal responsibility assessment, in which the borderline pattern of the ICD-11, qualified with serious severity, is seen as «the mad» by the juries (Baker et al., 2021). Due to the aforementioned, thre is a need for measures for the diagnosis of PD in legal settings to be convincing, adequately specify the severity of the PD, establish if there is a connection with a crime, and provide guidelines for the adequate management of the diagnosis, all of which considering the perspectives of rehabilitation and community protection (Carroll et al., 2022).
The measures that have been used to assess the personality of prison populations show that, internationally, approximately 65% of male convicts have a PD and conviction rates increase the severity of PDs, especially psychopathy. This specific construct is configured within PDs and appears to be an important predictor of subsequent violence, crime, and recidivism (Hengartner et al., 2018). In the national context, although research is limited, it was found that 17.1% of convicted sex offenders at the Lurigancho Penitentiary have a mental disorder, including PD (Sindeev & Guzmán-Negrón, 2019 ), while more than 50% of the inmates of the Arequipa Penitentiary show psychopathic deviation and 38.4%, antisocial disorder (Arias et al., 2016; Arosquipa & Gutiérrez, 2016). Moreover, at the local level, antisocial disorder (55.8%) and narcissism (51.2%) are the most prevalent PD diagnoses among the inmates of the DeVida program at the HP (Gomez, 2018). With the new ICD-11 PD nosology and its developed measures, these numbers will likely increase, given its greater specificity (Halliwell, 2021).
In Peru, it is difficult to use imported clinical instruments that measure PD -e.g., the Minnesota Multiphasic Personality Inventory (MMPI-3; Ben-Porath et al., 2022b), the Personality Assessment Inventory (PAI; Morey, 2007 ), or the Multiaxial Clinical Inventory of Millon (MCMI-IV; Millon et al., 2015)- because they do not fit to the special features of the level of education, culture, and/ or statistical disposition of the prison population (Carlson, 1981). Despite the fact that the authors of these measures have elaborated interpretation guides for the evaluation of personality in prison environments, and although the psychometric evidence -questionably- supports said measures (Neal et al., 2022), the items have been formulated based on the specific characteristics of clinical populations so that they can hardly be adjusted to those of the inmates of the Peruvian correctional system.
In summary, from a practical perspective, although the current clinical personality measurement instruments are relatively complete, they do not fit the educational, cultural, and statistical characteristics of Peruvian inmates. In addition, since the implementation of the WHO nosological classification is near in all state systems in which health professionals work, there is no adequate instrument for the ICD-11 PD model, which was designed from and for the evaluation of convicts. Moreover, from a theoretical perspective, the current personality assessment measures do not have a broad theoretical and substantive basis that understands the origin, current state, or the very sense of being of the personality from a continuous spectrum between health and disease. In this sense, it is necessary to develop a comprehensive measure of personality that meets the standards proposed in the ICD-11, and that, in order to extend its practical potential, incorporates solid theoretical support on said construct as a complex and singular entity.
Purpose of this research
To address this gap, this research aimed to develop and evaluate a comprehensive measure of personality from a mental health perspective in a correctional setting, which had to be adjusted to the most up-to-date standard proposed by the WHO (ICD-11; WHO, 2022a) for the diagnosis of PD.
This measure is based on an integrating paradigm of seven personality approaches with the greatest scientific impact throughout history: (a) evolutionary, focused on biopsychosocial adaptation (neurodevelopment); (b) multivariate, focused on heritable and stable dispositional traits; (c) psychodynamic, focused on the dynamism of the unconscious; (d) interpersonal, focused on meaningful social interaction; (e) personological, focused on narrative identity; (f) empirical, focused on correlates with personality disorders; and (g) salutogenic, focused on the healthy spectrum of personality. From these paradigms, the theory, five explanatory and evaluation models of the IDPI-11 were generated. One of these models, the integrative dimensional assessment of personality model, is shown in Figure 1.
Integrating the theoretical contributions of McAdams and Pals (2006) and De la Iglesia and Castro (2018), personality is defined as «an individual’s unique variation on the general evolutionary design for human nature, expressed as a developing pattern of dispositional traits, characteristic adaptations, and self-defining life narratives, complexly and differentially situated in culture and social context». Based on this definition, the integrative dimensional theory of personality is elaborated.
To identify individual differences, one must first understand the similarities among all living things. Thus, from the evolutionary paradigm, three evolutionary strategies are established for living beings: survival, adaptation, and replication. These strategies are homologous to those of human beings which include existence, agency, and transcendence. Other paradigms complement the understanding of personality by focusing on one or more evolutionary strategies.
The multivariate paradigm focuses on the existence to try to explain the personality of individuals from the question «What am I?». Thus, the actor self responds, «I am what I do», referring to the biological and environmental influence traits that qualify the concrete and stable trend of behavior. The interpersonal paradigm focuses on the agency to explain the personality from the question «How am I?». And the response from the agent self, «I’m how I do», describes that the motivations (composed of goals, strategies, and interpretations), determine the behavior of the individual in specific significant situations of the historical-cultural context. Thus, several people with the same trait can act differently because of their personal motivations, or the same person can act in a similar situation differently because of new motivations.
The narrative paradigm focuses on the transcendence to explain the personality from the question «What am I for?» and the response from the author self, «I am to give value to my existence», refers to the fact that people value and narrate their history and project it into the future to give consistency to their identity and meaning of life. The psychodynamic paradigm focuses on the agency and transcendence to explain, from defense mechanisms and object relations, the dynamism underlying the motivations and meaning of life. Likewise, the salutogenic and empirical paradigms are integrated into each of these three strategies to explain personality in a continuum of health and disease.
With the models and methods extracted from these paradigms that make up the core of the IDPI-
11, personality can also be assessed from its positive range (see De la Iglesia & Castro, 2018), and within the context of mental health, including factors associated with or in conjunction with the most prevalent psychopathology in the Peruvian context (see National Institute of Mental Health, 2019).
Methodological design
This research is a psychometric study because it includes the development of new instruments and appropriate methods for scoring, establishment of reliability and validity analyses for the measures, examination of the properties of the items and scales and/or their dimensions, and evaluation of the differential functioning of the items between subgroups (VandenBos, 2015). In psychometrics it is known that one of the approaches for developing measures uses a deductive process followed by an inductive one (Boateng et al., 2018). The deductive process is based on an iterative literature review of paradigms, theories, and models to formulate and delimit the construct (or constructs), dimensions, indicators and items, which, after being evaluated semantically and theoretically, follow an inductive process based on statistical refinement and the subsequent estimation of their properties so that their norms can be generalized (Kuhn, 2012).
In this sense, to address the objective of the development and evaluation of the measure, this research was divided into a pre-empirical phase and two phases of empirical studies, which together consisted of 13 steps. Preliminary phase included (1) identification of constructs and generation of items, and (2) item revision by a panel of five experts. Study 1: item refinement included (3) item calibration (selection) with classical test theory (CTT) and item response theory (IRT). Study 2: development, evaluation and normalization of the IDPI-11 scales included (4) item designation for the floating scales, (5) formulation of differentiated item scores for scales according to their prototypicality, (6) content validity of scales, (7) item-level analysis from CTT and IRT2, (8) structural validity of scales of the Traits subgroup (9) convergent and discriminant validity of the scales within their own groups, (10) validity of differentiation by groups of the scales, (11) criterion validity of Personality group scales, (12) reliability of scales, and (13) development of norms for the scales raw scores3. All the phases of this research address the development purpose and, in the last phase, the evaluation aim of the measure. Likewise, the sequential steps represent the specific objectives of each study (see Table D.1 in Appendix D for full details).
To develop the IDPI-11 scales and their corresponding items, a combination of theoretical and statistical test development methods was used: (a) the rational theoretical method for all the IDPI-11 scales, (b) the item response theory (IRT) for item calibration, (c) a sequential system for the development of construct-oriented scales for the elaboration of the scales of the IDPI-11 Traits subgroup, (d) content grouping with statistical refinement, and (e) a priori designation (Ben-Porath & Tellegen, 2020a; Williams et al., 2019). The rational theoretical method is based on the judgment of the creators of the instrument, and their understanding of psychopathology, for both the elaboration and selection of items. This method assumes a theory-based relationship between item content and the assessed personality attributes, giving each item face validity.
Likewise, the sequential system for the development of construct-oriented scales uses a modification of the exploratory factor analysis (EFA) to find, based on the exclusion of some items in the analysis, well-differentiated latent constructs which are adjusted to what is required by the theory. The IRT, on the other hand, allows refinement of the scales through calibration (selection of items) according to the level of the trait to be measured and the probability of supporting each item. Moreover, content grouping with statistical refinement, involves grouping items according to similar theoretical content, verifying the belonging of the item to the scale or the interassociation between individual items using correlational statistical methods (e.g., Spearman’s rho, polychoric correlations ( ρ), tetrachoric correlations (rtc), Cronbach’s α or CFA). Finally, a priori designation is a method for generating a scale without defined scoring content (Ben-Porath & Tellegen, 2020a Bender et al. 2011; Bender et al., 2018). Table D.1 of Appendix D shows the methods used to develop each of the IDPI-11 scales by steps and phases.
Preliminary development of the IDPI-11 scales
Identification of constructs and generation of items
For the identification of the construct, a vast literature was reviewed concerning the generation of new diagnostic models of PD based on the DSM-5
AMPD (see Cain & Mulay, 2022) and the ICD-11 (see Bach & Roger, 2022), focusing more on the last one because it is a global, intersectoral and multidisciplinary standard. Issues concerning the reasons for the new versions, theories that supported them, discussions and scientific debates around these classification systems were also reviewed. As a result, it was intended to incorporate mental health constructs to enable a better understanding of personality since, for example, Morey (2019) explained the importance of having instruments that are not only parsimonious but also complete and practical, as commercial instruments do well for clinical evaluation of personality. Also important was the inspiration from the work of Widiger (2016) and De la Iglesia and Castro (2018) who focused on the positive variants of maladaptive personality traits. In this sense, some authors were asked to share their instruments to thoroughly review the method of writing the items and their organization to measure the personality constructs. General psychopathology instruments were also reviewed, focusing on the constructs of psychopathology and mental health factors more frequently in the Peruvian population. Similarly, upon identifying the lack of test development (not validation) studies in the correctional population, we decided to implement our study in that setting.
By applying the rational theoretical method with some considerations -as explained in Table D.2 of Appendix D)- the constructs and items were generated and constantly revised, eliminated, added, and reformulated as new relevant information was obtained. In this way, the items were ready and organized with their respective indicators and dimensions: IDPI-11 «solid scales» which included R3, R4, F1, F2, F3, F4, F5, F6, F7, F8, S1, S2, S3, S4, S5, S6, S7, S8, S9, S10, a.1, a.2, a.3, a.4, a.5, a.6, a.7, b.1, b.2, b.3, c.1, c.2, c.3, d.1, d.2, d.3, d.4, e.1, e.2, and e.3. These were organized into groups and subgroups to facilitate the interpretation of the instrument in its final version. Likewise, taking a dimensional perspective of the personality constructs and integrating them into the salutogenic model, items with a positive or resilient connotation -strengths and virtues of personality (Peterson & Seligman, 2004)- were generated and accompanied the items with a maladaptive meaning to compose each of the scales of the Facets of Traits group. Then, those items were chosen and, due to their meaning, could belong to new scales without their own items. IDPI-11 «Floating scales» which included R1, R2, PF, A, B, C, D, E, and PL. These scales could not only be part of the personality construct but also part of others that may help to evaluate the biased responses of those evaluated as instruments already used in the prison system (see Table 4 to view the names of each of these scale codes). It should be noted that the floating scales PF A, B, C, D, and E were also composed of positive meaning items and maladaptive meaning items. The PL scale only included maladaptive items from the trait facet scales, given its categorical nature, similar to other scales of the Psychopathology group Appendix C provides a brief description of each of the IDPI-11 scales.
Finally, the items were read and reviewed by a 68-year-old male who had only completed the third grade of primary school. Thanks to his comments, these items were restructured so that they were clear and understandable for the target prison population. Also, the pertinent administrative, ethical and logistical procedures were already being carried out to be able to evaluate said population.
Item revision by a panel of experts
The whole instrument was validated by a panel of five experts: the number of experts recommended according to Zamanzadeh et al. (2015). One of these experts is currently working in the penitentiary system, with approximately 10 years of practice, and four of them have similar average experience in the field of clinical psychology. The instrument developed is especially focused on clinical and mental health constructs, which is why most of the panel of experts belong to this area. However, as the instrument was standardized in a prison sample, it was pertinent to complement it, at least, with the validation of experts in that area to obtain a valid and reliable instrument. The experts were asked to review the items regarding the criteria of «clarity». After this review, and after an analysis of the content validity index of each item, satisfactory results were obtained, since 222 items reached item content validity indices (CVI- I) in the range of .80 to 1; therefore, 59 items were reformulated taking into account the observations provided. Likewise, it is important to mention that no item was eliminated in this step, since all 222 items had a CVI-I equal to or greater than .80 and item content validity ratios (CVR-I) greater than .60. Although the cut-off criteria may vary depending on the size of the panel of reviewers, in general, Almanasreh et al. (2019) indicated the following: CVI-I = .78 to 1, to be retained; CVI-I < .78, to be eliminated; and CVR-I = .60 to 1, to be retained, and CVR-I < .60, to be eliminated. These 222 items, which were already reviewed, were randomly reordered to be administered to participants of study 1.
Study 1: item refinement
Methods
The main objective of the first study was to select parsimonious items from the CCT and IRT to be kept in the composition of the IDPI-11 scales. Although brief in time and austere in sample size, the first application of the instrument is important to predict and meet psychometric needs in a subsequent larger study. It also provides an opportunity to learn about the potential limitations in the examinee’s performance in the test and the logistical complications.
Participants
Through a random sampling stratified by type of crime, 70 inmates were selected from the HP, a male penitentiary located east of the city of Huancayo, assuming a loss rate of 10% after evaluating the eligibility criteria, which excluded the cases with 10 or more blank or null answers and/or some disability that limited the willingness to give consent at the time of the evaluation. The final sample of this study consisted of 60 inmates with an average age of 38.3 years and an age range between 26 and 49 years (81.7%). In addition, it was shown that most inmates had a cohabiting partner (20%), did not finish secondary school (35%), came from the Peruvian highlands (65%), were located in pavilion P2 (33.3%), were sentenced to a minimum security facility (38.3%), were detained for crimes against public administration (38.7%), have spent from 4 to 12 years in the HP (23.3%), and were serving a prison sentence also from 4 to 12 years (58.3%). See Table 1 for more details on this sample.
Measures
Integrative Dimensional Personality Inventory-11 (IDPI-11). This measure was developed for the present study and consisted of 222 false/true (F/T) initial items, distributed in 49 scales4: 4 Response Styles scales, 8 Mental Health Factors scales, 7 Personality scales, 10 Psychopathology scales, and 20 Trait Facets scales. The instrument had a booklet of questions with relevant instructions. It also had an answer sheet that included a section for sociodemographic data (date of birth, marital status, level of education, and place of origin) and another one to fill in the answers to the items.
Procedure
The data collection was performed during the first week of April 2021, meeting the respective ethical and logistical procedures. To carry out the data collection, permission from the Ethics and Research Committee of the Faculty of Humanities (CEI-DD-HH) of the Continental University, as well as the Directorate of Penitentiary Treatment of the National Penitentiary Institute of Peru - Junín Region, was obtained. Then, the head of the psychology department was trained in the procedures for administering the instrument and he, in turn, trained his personnel, since contact was restricted in the prison area due to the COVID-19 pandemic. Inmates sat at a (personal) desk with a pencil, blue or black pen, and eraser.
The informed consent and instrument sheets were available on the desk. Next, the psychologist monitored the entire administration procedure of the instrument. In addition, he was in charge of excluding the inmates who did not have the decision-making capacity to sign the informed consent and complete the questionnaire. Likewise, the psychologist signed the informed consent form as a witness for each of the examinees who participated in the study. The completed study 1 questionnaires and informed consent forms were collected from the prison on the day after the last day of the week. Data collection for study 1 was carried out in two steps in an average total time of 36 minutes to avoid examinees to get tired.
Furthermore, the techniques used for data collection were: (a) a survey that consisted of the application of the instrument and (b) a review of secondary data from a database with information on the type of crime, prison security level imposed, and other data (age, National Identity Card number, pavilion, time served in prison, and length of sentence). Although not all were analyzed inferentially, they are presented in Table 1 for a better understanding of the characteristics of the sample in this study. In addition, Microsoft Excel v. 2019, IBM SPSS Statistics 28.0.1.0, and RStudio v. 2021.09.1+372 were used for data processing and analysis. Furthermore, missing data were imputed with the abovementioned second software using the multiple imputation method.
Data analysis
For the descriptive statistics of the sociodemographic characteristics of the sample, the mean age and mode of the rest of these characteristics were calculated using IBM SPSS Statistics. To select the items from the classical test theory (CTT) approach, Spearman’s rho statistics were analyzed to relate each item to its original scale using IBM SPSS Statistics. The cut-off point of rho ≥ .70 was used to retain the item, since it implies a strong correlation (Akoglu, 2018). In addition, using Microsoft Excel, the items difficulty index (p) and discrimination index (D) were analyzed, following the algorithm of Reynolds and Livingston (2019). The cut- off points used were: (i) for items difficult to endorse by the participants -including all «solid scales,» except for scales F1, F2, F3, and F4- the cut-off points were p < .50 and D ≥ .30 to retain the ítem and (ii) for items theoretically easy for participants to endorse -including items of scales F1, F2, F3, and F4- p = .30 to .70 and D ≥ .30 were used to retain the item (Reynolds and Livingston (2019).
Furthermore, parameters b and a of the item characteristic curve from the IRT approach were also used in this step with the RStudio «psych» package. For items that were theoretically difficult to ratify -including items of all solid scales, except for scales F1, F2, F3, and F4- the cut-off point of b ≥ 0 was used to retain these items because they measure psychopathological constructs (Nguyen et al., 2014; Yang & Kao, 2014). In addition, another threshold of b = -2 to 2 was used to retain the item because these items were theoretically not difficult or easy for participants to endorse -including items of scales F1, F2, F3, and F4-. For the item discrimination parameter across all items of «solid scales,» the cut-off point of a ≥ 0 was used to retain them (Nguyen et al., 2014; Yang & Kao, 2014).
Table 1 Sociodemographic characteristics of study 1 and study 2 samples
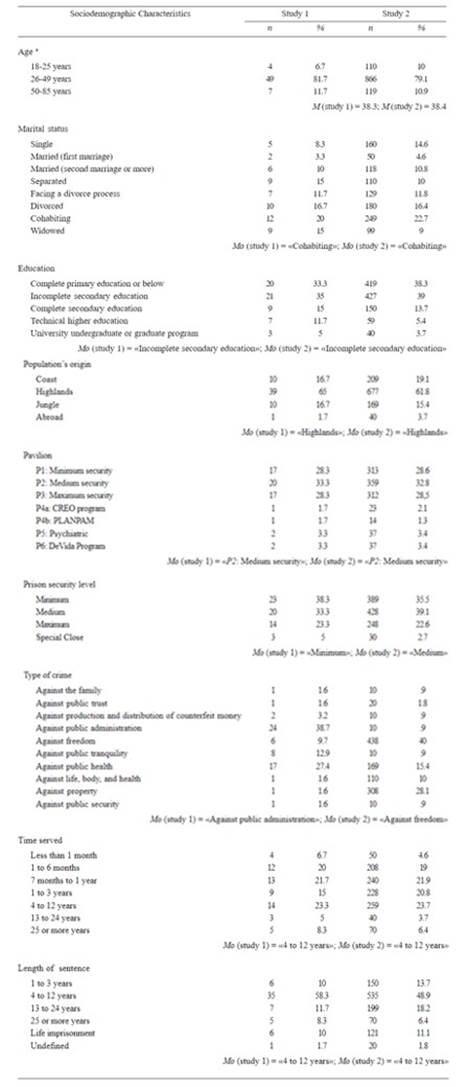
Note: N = 2009 (n study 1 = 60; n study 2 = 1095); M = mean; Mo = mode. Central tendency statistics were observed: mean in the case of quantitative variables and mode in the case of qualitative variables. a This variable was transformed into a qualitative ordinal variable to facilitate its understanding.
Results
After conducting the analyses for the selection of items in study 1, 32 items were eliminated for not reaching adequate levels in all calibration indices (see Table 2). Note, for example, that item 7 «Solo por las mañanas siento que me falta el aire» obtained adequate levels of item-scale correlation (rho = .76) and discrimination from the TCT (D = .31) (Akoglu, 2018; Reynolds & Livingston, 2019), but the other calibration indices had inadequate levels (p = .33; b = -8.99; a = -.15) (Nguyen et al., 2014; Reynolds and Livingston (2019; Yang & Kao, 2014). Another example is in item 8 «Actualmente, me siento terriblemente deprimido y triste la mayor parte del tiempo», which measures a psychopathological construct. It obtained an adequate level of difficulty indices from the TCT and IRT (p = .52; b = .14) (Nguyen et al., 2014; Reynolds and Livingston (2019; Yang & Kao, 2014), but its other indices were inappropriate (rho = .67; D = .09; α = -.01) (Akoglu, 2018; Nguyen et al., 2014; Reynolds and Livingston (2019; Yang & Kao, 2014).
Table 2 Statistics of items eliminated from the IDPI-11 after the refinement
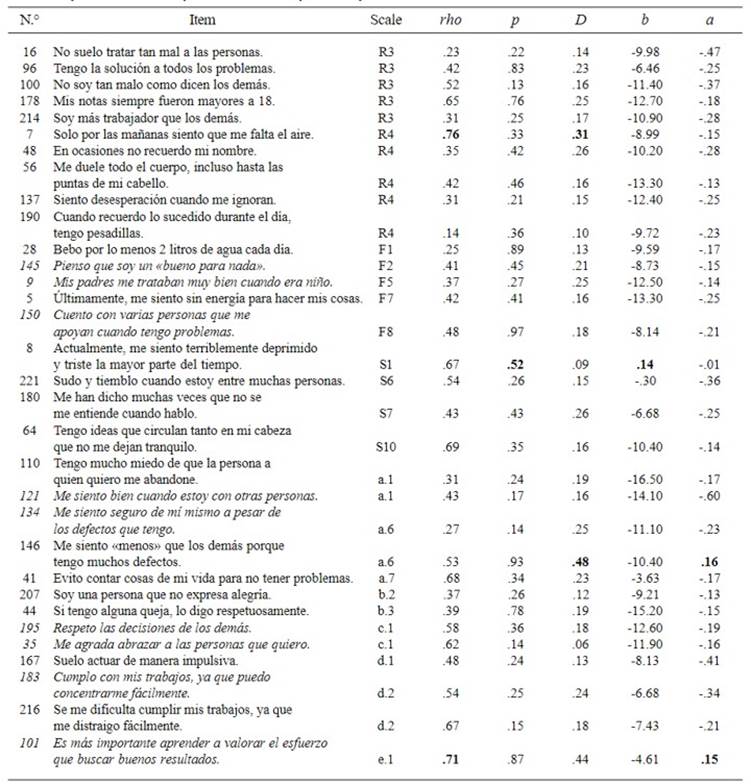
Note: rho = Spearman’s rho; p = difficulty index (TCT); D = discrimination index (TCT); b = difficulty parameter (TRI); a = discrimination parameter (TRI); R3 = Dissimulation; R4 = Simulation; F1 = Healthy Habits; F2 = Self-Esteem; F5 = Childhood Abuse; F7 = Health Concern; F8 = Lack of Social Support; S1 = Major Depression; S6 = Agoraphobia; S7 = Schizophrenia Spectrum; S10 = Obsessive-Compulsive Disorder; a.1 = Calm vs. Anxiety; a.5 = Humor vs. Depressiveness; a.6 = Initiative vs. Shame; a.7 = Faith in Others vs. Distrust; b.2 = Love vs. Emotional Detachment; b.3 = Assertiveness vs. Lack of Assertiveness; c.1 = Altruism vs. Egocentrism; d.1 = Prudence vs. Temerity; d.2 = Commitment vs. Irresponsibility; d.4 = Emotional Fullness vs. Thrill Seeking; e.1 = Frustration Tolerance vs. Perfectionism. The table shows all the items eliminated for not meeting adequate levels in all calibration indices. Inverted items with respect to their scale are shown in italics. Indices with adequate level of calibration are shown in bold.
Among the eliminated items, indicators in which no longer any items were eliminated. For example, the Minimization indicator, and the Defensiveness and Positive Impression dimensions of the R3 scale. Other eliminated indicators include Infrequent Symptoms of Post-Traumatic Stress from the R4 scale, Social Support Not Specified from the F8 scale, Intense Sadness from the S1 scale, Conceptual Disorganization from the S7 scale, Social Deterioration Due to Obsessions from the S10 scale, Comfort During Social Relationships vs. Social Anxiety from the a.1 scale, and Ease of Concentration vs. Distractibility from the d.2 scale. After deleting the items, the selected items were randomly sorted and renumbered according to their quantity.
Study 2: development, evaluation and normalization of IDPI-11 scales
Methods
This study has three sections: (a) Development of the IDPI-11 scales with the parsimonious items selected in the previous study. (b) Evaluation of the psychometric properties of the developed measure. (c) Norms derivation for IDPI-11 scales. Eleven specific objectives of this study are included in each of these sections. The first section includes the following objectives: (1) to designate items for the floating scales and (2) to formulate differentiated item scores for scales according to their prototypicality. The second section includes these objectives: (3) to analyze the content validity of scales, (4) to perform an item-level analysis of the measure with CTT and IRT, (5) to analyze the structural validity of the scales of the Trait subgroup, (6) to analyze the convergent and discriminant validity of the scales within their own groups, (7) to analyze the validity of differentiation by groups of the scales, (8) to analyze the criterion validity of Personality group scales, and (9) to analyze the internal reliability of the IDPI-11 scales. The third section includes one objective: (10) to develop norms for the raw scores of the IDPI-11 scales .
Participants
Through a random sampling stratified by type of crime, 1130 inmates from the HP were initially included, assuming a loss rate of approximately 10% as a result of the eligibility criteria. The inclusion criterion was the approval of the consent form for the evaluation by the convict, and the exclusion criteria were cases with 10 or more blank or null answers and/or some disability that limited the willingness to give consent at the time of the evaluation. As a result, a representative sample of
1095 inmates remained eligible for the study: an adequate sample size since it has a CI = 95% and e
= 2% (see Bayne, 2018; Dillman et al., 2014). The average age of the inmates was 38.4 years, with an age range between 26 and 49 years (79.1%). In addition, it was shown that most inmates had a cohabiting partner (22.7%), did not finish secondary school (39%), came from the Peruvian highlands (61.8%), were located in pavilion P2 (32.8%), were sentenced to a medium security facility (39.1%), were detained for crimes against freedom (40%), have spent from 4 to 12 years in the HP (23.7%), and were serving a prison sentence from 4 to 12 years (48.9%). See Table 1 for more details on this sample.
Measures
Integrative Dimensional Personality Inventory-11 (IDPI-11). This measure was developed for the present study with the calibrated items. The IDPI-11 evaluates the personality of the examinees in a dimensional (health-disease continuum) and integrative manner (integrating related constructs for a differential diagnosis and a complete evaluation) in the context of mental health. It uses a dichotomous format for its answers, which speeds up its application. The response options are «F» or «T» for its 190 items distributed in 49 scales within nine subcategories and five main groups. The instrument includes 4 Response Style scales (Invalidity, Inconsistency, Dissimulation, and Simulation), 8 Mental Health Factors scales, 10 Psychopathology scales, 7 Personality scales, and 20 Trait Facet scales .
In addition, it can be applied individually or in groups in approximately 36 minutes.
Procedure
The data collection was performed from April to July 2021. Like study 1, the test was performed in two steps. It followed the same procedures as the previous study because it was granted permission from the pertinent authorities of the prison and the Department of Ethics of the Continental University. Furthermore, the techniques used for data collection were: (a) a survey that consisted of the application of the instrument and (b) a review of secondary data from a database with information on the type of crime, prison security level imposed, and other data (age, National Identity Card number, pavilion, time served in prison, and length of sentence). On this occasion, some sociodemographic characteristics such as age, type of crime, and prison security level were used to perform inferential statistics and assess the criterion validity of the Personality group scales. In addition, Microsoft Excel v. 2019, IBM SPSS Statistics 28.0.1.0, IBM SPSS Amos 28.0.0, and RStudio v. 2021.09.1+372 were used for data processing and analysis. Likewise, missing data were imputed with the abovementioned second software using the multiple imputation method.
Data analysis
For the descriptive statistics of the sociodemographic characteristics of this sample (step 5), the mean age and mode of the rest of these characteristics were calculated using IBM SPSS Statistics.
Development of scales. To designate items for the floating scales (objective 1) -taking into account the considerations described in Table D.2 of Appendix D- the a priori designation method was used. It consists in selecting all the items of the IDPI-11 to compose Invalidity (R1 scale), thus the raw score of this scale is increased by «1» point each time the examinee answers blank or null. This method is similar to those used by Ben-Porath and Tellegen (2020a) to compose the Can Not Say (CNS) scale of the MMPI- 3 -although this procedure did not require statistical analysis and therefore had no results-, it is important to report the answers. Likewise, to compose Inconsistency (R2 scale), the content grouping with statistical refinement method was used. It consisted in evaluating the semantics of pairs of IDPI-11 items with similar content and items with opposite content. The pairs of items with similar content were mainly drawn from the same scale (e.g., both Agoraphobia items or Post-Traumatic Stress items). On the other hand, the pairs of items with opposite content were extracted from those items formulated for trait facets that have items of positive meaning and items of maladaptive connotation. Then, the pairs of selected items were evaluated using tetrachoric correlations (r ) from RStudio «psych» package and cut-off points of> .70 to confirm its relevance in the R2 scale (Glen,2016).
Likewise, the items of the scales of the Facets of Traits group were also designated to compose the scales of the Traits subgroup -Serenity vs. Negative Affectivity (scale A), Humanity vs. Detachment (scale B), Integrity vs. Dissociality (scale C), Moderation vs. Disinhibition (scale D), and Psychological Flexibility vs. Anankastia (scale E)- using the sequential system of construct-oriented scale development. This procedure implied that the adjustment of the exploratory factorial analysis (EFA) was verified using IBM SPSS Statistics through the varimax rotation of this group of items. Kaiser- Meyer-Olkin (KMO) and Bartlett’s Test of Sphericity (BTS) were used with a cut-off point >.75 of KMO and a chi-square p-value (p-value (χ²))< .05 of BTS as pre-requisites to evaluate the EFA (Ferrando et al., 2022; Indrayan & Holt, 2017). After verifying the adjustment of the EFA, the lambdas (λ) of the items’ factor loads were analyzed, and only those with λ > .30 were considered adequate (Padilla, 2019). Moreover, taking into account the guidelines of Padilla (2019), the subsequent EFA required constant elimination from the analysis, items with low communalities (h2 < .30)5 and those items mainly loaded on the last factors. With this, it was possible to obtain items loaded on the five factors required for the scales of the Traits subgroup.
Next, the items were designated for the composition of the Functioning (PF) and Borderline Pattern (PL) through the content grouping with statistical refinement method to identify possible items of the Facets of Traits group scales to compose such scales. To have a good theoretical basis, the ICD-11 guidelines on the severity of PD were revised, which were mainly based on intra and interpersonal dysfunction, similar to criterion A of the DSM-5 AMPD (Section III). Existing measures of this construct were also reviewed to identify items corresponding to these main characteristics and to include them in the PF scale. The procedure for initially composing items on the PL scale was similar. Once the items were identified, one- factor CFA with the maximum likelihood (ML) estimator from IBM SPSS Amos was used to compose each of these scales. The cut-off points used to assess the goodness of fit of the model were: relative chi- square (χ²/df) < 5, root mean squared error of approximation (RMSEA) ≥ .05, Tucker Lewis index (TLI) ≥ .90, standardized root mean square residual (SRMR) ≤ .08, and comparative fit index (CFI) ≥ .95 (Boateng et al., 2018; Collier, 2020). Once the fit of the model was confirmed, the factor loads were assessed, and a cut-off point of λ ≥ .60 was used to retain the items in each scale (Awang, 2014).
To formulate differentiated item scores for scales according to their prototypicality (objective 2), the content grouping with statistical refinement method6 was used. It includes only items from solid scales; therefore, Spearman’s rho was calculated using IBM SPSS Statistics to analyze the relations between the items of the solid scales and the other solid scales and thus assign non-prototype items to other scales. It was verified that these items provide a theoretical and diagnostic contribution to the scale in order to finally include it (Grossman, 2019; Millon et al., 2015). The cut-off point used to consider the inclusion of an item in the target scale was a moderate to high degree of correlation, I rho I ≥ .40 (Akoglu, 2018).
It should be noted that, although the prototype items of the solid scales Dissimulation (R3) and Simulation (R4) were designated as non-prototype items in other scales of the IDPI-11, prototype items of other scales were not designated as non-prototypes in the R3 or R4 scale. This is due to the possibility that, in an acute episode of psychotic symptomatology (e.g., with a high score on the Schizophrenia Spectrum (S7 scale)), the prisoner may endorse items in the R4 scale, which does not imply a «faking bad» performance (Sellbom et al., 2022). This situation can also occur in the way that an examinee with egocentric characteristics (e.g., with a high score on Altruism vs. Egocentrism (scale c.1)) supports items on the R3 scale, which does not imply a «faking good» performance (Ben-Porath et al., 2022). However, the fact of dissimulating (elevation in the R3 scale) and feigning (elevation in the R4 scale) is often accompanied by similar responses -supported by items in the IDPI-11 scales that measure egocentrism (scale c.1) and psychotic symptomatology (S7 scale)- due to the tendency of the inmates’ responses (Ben-Porath et al., 2022; Sellbom et al., 2022).
Thus, the designation of the scores for the scales was relatively simple. A score of «2» was established
if the item supported by the examinee was adequate to its scale (prototype item), and a score of «1» if the item was not appropriate (non-prototype item). With this, direct non-prototype items correspond to objective scales with the same direction as their original scale-either towards health or disease7- and inverse non- prototype items correspond to target scales with a direction opposite to that of their original scale.
Evaluation. To analyze the content validity of scales (objective 3), CVI-S was used -which is the average of the CVI-I of the items that compose it, also called Ave-CVI-I- from the CVI-I of the prototype and non-prototype items of each scale, based on the panel of experts’ assessment of the IDPI-11 items conducted in step 2 with a cut-off point of CVI-S > .80 to consider an adequate level of content validity (Almanasreh et al., 2019).
Then, to analyze an item-level analysis of the measure with CTT and IRT (objective 4), successive one-factor CFAs were performed to assess the degree of relationship between the items (prototypes and non- prototypes) and the latent factor of each scale using IBM SPSS Amos and the standardized RMR plugin. To do this, first, the fit of the one-factor models was evaluated taking as cut-off point χ²/df < 5, RMSEA ≥ .05, TLI ≥ .90, SRMR ≤ .08, and CFI ≥ .95 (Boateng et al., 2018; Collier, 2020). Then, the factor loads were analyzed taking the cut-off point as appropriate: λ ≥.60 (Awang, 2014).
Likewise, to perform the item-level analysis from IRT -having fulfilled the assumption of unidimensionality of the IRT, evidenced in the adjustment parameters of the CFA- we proceeded to evaluate the assumptions of monotonicity, local independence and invariance of the items with the monotonicity violation criterion (crit), ítem discrimination parameter (a), and differential item functioning (DIF) statistics from RStudio’s «mokken»,«psych», and «mirt» packages, respectively. This considered the following as cut-off points: crit < .80, to confirm the monotonicity; a ≤ 4, to confirm local independence; chi square (χ²) > 3.3; and p-value (χ²) ≥ .05 of DIF to confirm the invariance of the items according to the age groups and type of crime (Nguyen et al., 2014). Also, using RStudio’s «mirt» package, and as suggested by Nguyen et al. (2014), the adjustment of the appropriate IRT model to the items of each of our scales was evaluated, comparing the models of two (2PL) and three parameters (3PL), as is usually done in the literature for dichotomous items. To do this, the -2 log-likelihood ratio (-2LL) statistic, also called the Likelihood ratio test, was used with the RStudio «mirt» package. In addition, as suggested by these authors, the fit of the selected IRT model was evaluated at the item level (Nguyen et al., 2014), with the Orlando and Thissen’s chi square (S- χ²) statistic, using the same package. For the last two previous analyses, the cut-off point p-value (χ²) ≥ .05 of -2LL was used to confirm that the most parsimonious model (2PL) fits better than the most complex and flexible model (3PL), and a p-value (S- χ²) ≥ .05 was used to confirm the good fit of each of the items for each of our scales (Nguyen et al., 2014).
Finally, and after evaluating these IRT prerequisites, we proceeded to analyze the statistics: item difficulty parameter (b) and item discrimination parameter (a) with the RStudio «psych» package, using the cut-off points of b = -2 to 2 for items not difficult to support (including the items of scales F1, F2, F3, and F4), b ≥ 0 for construct items theoretically difficult to support (including the items of the rest of the scales), and a ≥ 0 for all the IDPI- 11 items to valuate a good level of discrimination (Nguyen et al., 2014; Yang & Kao, 2014 ).
To analyze the structural validity of scales of the Trait subgroup (objective 5), a five-factor CFA (with the ML estimator) was used using IBM SPSS Amos and its standardized RMR plugin, with the cut-off points recommended by Boateng et al. (2018) and Collier (2020): χ²/df < 5, RMSEA ≥ .05, TLI ≥ .90, SRMR ≤ .08, and CFI ≥ .95 to consider an adequate fit of each of the models. Next, the factor loads were analyzed, considering the cut-off point λ ≥ .60 as appropriate (Awang, 2014).
For objective 6, to analyze the convergent and discriminant validity of the scales within their own groups, consecutive CFAs of the IDPI-11 scales were analyzed by group using IBM SPSS Amos and its «master validity» and «standardized RMR» plugins. For this, the cut-off points mentioned by Boateng et al. (2018) and Collier (2020) were used for the indices of good fit. Then, the average variance extracted (AVE) and Pearson’s r correlations were analyzed, and the appropriate values of convergent validity for a scale resulted in an AVE ≥ .50 (Bello, 2016). The optimal values of discriminant validity implied that the square root of a scale AVE is greater than the correlations between said scale and the other scales of the subgroup (Ab Hamid et al., 2017; Bello, 2016).
For objective 7, to analyze the validity of differentiation by groups of the scales, we first evaluated whether the raw scores of the IDPI-11 scales, according to age groups (group 1 = 18 to 34 years old, group 2 = 35 years old and older) and type of crime (see Table 1), had a normal distribution using IBM SPSS Statistics. To assess the indices of Kolmogorov-Smirnov (KS) for groups of 30 or more cases and the indices of Shapiro-Wilk (SW) for groups with fewer than 30 observations, we used a p-value > .05 for both indices as confirmation of the non- normality of these distributions (Frey, 2022). Later, with the RStudio «onewaytests» package, the homoscedasticity of the variances was e valuated using the Fligner-Killeen index (χ 2) with the cut-off point of p-value (χο ) > .05 to confirm said homogeneity
(Frey, 2022). After confirming non-normal distributions and similar variances in the scales, the Mann-Whitney (U) and Kruskal-Wallis (H) indices were used with IBM SPSS Statistics to assess whether there were significant differences between these groups, with the p-value ≤ .05 of both indexes showing a clear difference (Frey, 2022). In addition, two algorithms were used to calculate the effect sizes of said differences through Microsoft Excel, with the Glass rank biserial correlation coefficient (r g ) for U -with ( King et al. (2018 ) algorithm- and with epsilon squared (ε2) for H -with Tomczak and Tomczak (2014) algorithm-. The cut-off points of |r g | ≥ .40 and ε2 ≥.04 were considered moderate effects or more (King et al., 2018; Stikker, 2018).
Then, to analyze the criterion validity of Personality group scales (objective 8) using IBM SPSS Statistics, Nagelkerke’s pseudo-R2 coefficient of determination (R2 N) and odds ratio (OR) statistics were first analyzed as fit indicators and predictors in logarithmic regression models to explain the presence of crimes against public health, heritage, liberty, and life, body, and health. For this, the parameters of R2 N ≥ .50 (Frey, 2022) and p-value (OR) < .05 (Sperandei, 2014) were evaluated and thus considered significant. Then, the R squared coefficient of determination (R2) and beta (β) statistics were analyzed for predictive analyses of the linear regressions of the Personality group scales to explain the increase in the prison security level. The parameters of R2 ≥ .50 (Frey, 2022) and p-value (β) < .05 (Ali & Younas, 2021) were taken into account to consider a good fit of the model and a significant predictive capacity of the scale, respectively. And, finally, to analyze the reliability of IDPI-11 scales (objective 9), McDonald’s omega (ω) was computed for items of each scale using the new features of IBM SPSS Statistics, and the parameter to assess an adequate degree of internal consistency was ω ≥ .70 (Olivas-Ugarte & Cipriani-Delgado, 2022).
Normalization. For objective 10, to develop norms for the raw scores of the IDPI-11 scales (traditional and uniform), the mean (M), standard deviation (SD ) skewness (Sk), and position (percentile score (Pc)) were calculated using IBM SPSS Statistics. The methodology of Ben-Porath and Tellegen (2020a) was followed to transform the raw scores of the IDPI-11 scales into uniform T scores (UT scores), similar to the scores established in the MMPI-3 scales with right-skewed PD distributions (Sk ≥ .1). Thus, the final scores were generated for the raw scores of each IDPI-11 scale. Traditional T scores (TT scores) were designated for the Healthy Habits (F1), Self-Esteem (F2), Significant Activities (F3), and Openness to Treatment (F4) scales, because their distributions do not have a bias to the right. Additionally, UT scores were elaborated for the scales in case they had this bias. The steps for the construction of UT scores of the aforementioned scales involve a «matching» between the raw scores and integer composite traditional T-scores (ICTT scores) as described below:
(a) First, the means and standard deviations of the raw scores on each scale were calculated.
(b) TT scores of each raw score obtained for each scale were calculated.
(c) Next, percentiles .5, 1, 2, …, 99, 99.5 (101 percentiles) of the TT score of each scale were found.
(d) Subsequently, the average of the TT scores of all scales for each percentile was calculated, the results of which were incorporated into a new column called the composite traditional T score (CTT score).
(e) After obtaining the CT scores for each percentile, all TT scores for each scale were replaced with their respective raw scores.
(f) Next, the raw score of each ICTT score -ICTT scores were used as a template- were calculated through linear interpolation.
(g) Then, to determine the UT scores of the raw scores obtained, UT scores were calculated from polynomial interpolations of degree 3: raw scores of the ICTT scores less than 60 and UT scores of the raw scores of the normative simple corresponding to ICTT scores equal to or greater than 60, were determined by linear interpolation, which served to verify that the UT scores were the same as the ICT scores as the test intended, and indeed they were.
(h) Finally, to establish raw scores that were outside the range of the normative sample, corresponding to the UT scores, linear extrapolations were performed by establishing a lower limit (UT score = 20) and an upper limit (UT score = 100). Thus, any extreme raw score outside the linear extrapolations would take the UT score corresponding to the upper or lower limit, as the case may be. For more details, see Appendix F.
Results
Development of scales. In item designation for the floating scales (objective 1), using content grouping with statistical refinement method, 55 pairs of items with high tetrachoric correlations (r > 77) were obtained for Inconsistency (R2 scale). For example, for pairs of items with opposite content (49 pairs in total) corresponding to the scales of the Trait Facets group, most of the correlations were greater than .80; whereas for pairs of items with similar content (six pairs in total), the tetrachoric correlation was in the range of .78 to .86. Consequently, these 55 pairs in total were included with strong correlation coefficients to compose the R2 scale (Akoglu, 2018).
For the composition of scales of the Facets of Traits group, the sequential system of construct- oriented scale development was applied through a series of EFAs up to a five-factor solution with previous verification of its adequacy: KMO = .81, χ² = 2314.9, p-value = .00 (Ferrando et al., 2022; Indrayan & Holt, 2017). Thus, following Padilla’s criteria (2019), only three items from the a.1 scale, two items from the a.3 scale, two items from the a.4 scale, and two items from the a.6 scale obtained adequate factor loadings (λ = .35 to .73) to compose scale A. In addition, six items belonging to scale b.1 and two items belonging to scale b.2 obtained relevant levels of factor loadings (λ = .40 to .71) to compose scale B. Likewise, two items from the c.1 scale and five items from the c.2 scale obtained appropriate factor loadings (λ = .38 to .63) to compose scale C. Similarly, three items from the d.1 scale, two items from the d.2 scale, and five items from the d.4 scale obtained adequate indices of factor loads (λ = .32 to .71) to compose scale D. Finally, three items from the d.1 scale, two items from the e.2 scale, and two items from the e.3 scale obtained relevant indices of factor loads (λ = .37 to .69) to compose scale E.
Then, for the composition of Functioning (PF) and Borderline Pattern (PL), the content grouping with statistical refinement method was used through one- factor confirmatory analysis (CFA) for each scale - with previous verification of its adequate goodness- of-fit indices: χ²/df = 4.3, RMSEA = .06, TLI = .95, SRMR = .07, and CFI = .97 for scale PF and χ²/df =3.8, RMSEA = .08, TLI = .92, SRMR = .06, and CFI= .99 for scale PL (Boateng et al., 2018; Collier, 2020). Once the fit of the model was confirmed, appropriate levels of factor loads were evidenced (λ = .71 to .86) to designate items from the scales of the Facets of Trait group -from a.2 (one item), a.3 (4), a.5 (2), b.1 (4), b.2 (2), c.2 (2), e.1 (2), and e.3 (4)- to compose the PF scale (Awang, 2014). Similarly, considering the cut-off proposed by Awang (2014), appropriate levels of factor loadings were evidenced (λ = .76 to .92) to designate items from other scales -from a.2 (one item), a.2 (1), a.3 (3), a.4 (3), a.5 (1), a.7 (2), c.2 (1), d.1 (1), d.4 (3), and S8 (1)- to compose the PL scale.
In the formulation of differentiated item scores for solid scales according to their prototypicality (objective 2), the content grouping with statistical refinement method was applied. This implied that the items of the other solid scales were related to a moderate-to-high level with the total score of the objective scale. After the analyses, correlations were obtained in the range of |rho| = .40 to .66. These items were designated as direct non-prototype items for having positive correlations with the total score of the target scale (scored as F = ‘0’ and T = ‘1’) and asinverse non-prototype items for having negative correlations (scored as F = ‘1’ and T = ‘0’). See Table E.1 of Appendix E for details on these correlations. Consequently, solid scales with an average of 3.2 (from 1 to 7) direct non-prototype items and an average of 1.7 (from 1 to 5) inverse non-prototype items could be obtained, excluding from the account the solid scales that did not have any direct or inverse non-prototype items. The final composition by items of all IDPI-11 scales is shown in Appendix H.
Evaluation. In the analysis of the content validity of scales (objective 3), CVI-S was used as the CVI-I average of all items that integrate each scale, based on the assessment of the IDPI-11 items in step
2 conducted by the panel of experts (item revision by a panel of five experts). The results showed adequate levels of content validity for all scales, since CVI-S was found in the range of .88 to 1 (Almanasreh et al., 2019).
In the item-level analysis from CTT (objective 4) through one-factor CFAs for each scale, in general, with good fit indices, χ²/df in the range of 2 to 4, CFI > .96, TLI > .95, RMSEA < .07, and SRMR > .06 (Boateng et al., 2018; Collier, 2020), IDPI-11 items obtained good levels of factor loads with their original scales (λ1 > .59) (Awang, 2014). However, their mean levels of factor loadings with their target scales varied (λ2 = .30 to .78).
In the item-level analysis from IRT, the unidimensionality of the IDPI-11 scales was confirmed by considering the adequate goodness-of- fit indices of the one-factor confirmatory analyses shown in the previous analysis (Nguyen et al., 2014). In addition, considering the criteria of Nguyen et al. (2014), the monotonicity, local independence and invariance of the items were adequate for each of the IDPI-11 scales with crit = .23 to .65, a = .1 to 3.6, and χ² = 3.6 to 5.8 (p-value = .06 to .08), respectively. Similarly, in the IRT model fit test, the most parsimonious model (2PL) was adequate with χ² = 4.6 to 8.2 (p-value = .05 to .07) in the -2LL test; in addition, the adjustment at the item level showed S-χ² = 6.3 to 8.7 (p-value = .06 to .09).
Once the assumptions of the IRT were verified and the best fit of the 2PL model was evidenced, the results of the IRT parameters showed that the IDPI-11 items differed well in the high and low levels of the construct according to the probability of supporting the item in the direction of its original scale (a > .1). Likewise, the difficulty parameters showed, in general, adequate levels according to the construct they measured, although there were some exceptions: for example, item 19 (b = .3) and item 26 (b = -.2), which focus on depressiveness and distrust, and are frequently supported in the study population. These parameters showed that the items of the clinical construct were difficult to support for the examinees (b > .5) and that the non-clinical constructs obtained a varied range of difficulty (b > -.6). These results are generally consistent with the levels of probability of support and the degree of change in said support in relation to the measured trait, according to some authors (Nguyen et al., 2014; Yang & Kao, 2014). See Table E.2 in Appendix E for more details.
Then, in the structural analysis of the Trait subgroup scales (objective 5), through the a five- factor CFA, adequate goodness-of-fit indices were obtained for the Five Factors model: χ²/df = 4, CFI = .99, TLI = .91, RMSEA = .65, and SRMR = .52 (Boateng et al., 2018; Collier, 2020), as well as appropriate levels of factor loads (λ ≥ .70) (Awang, 2014). Figure 2 presents the CFA standardized factor loads for the items of these scales.
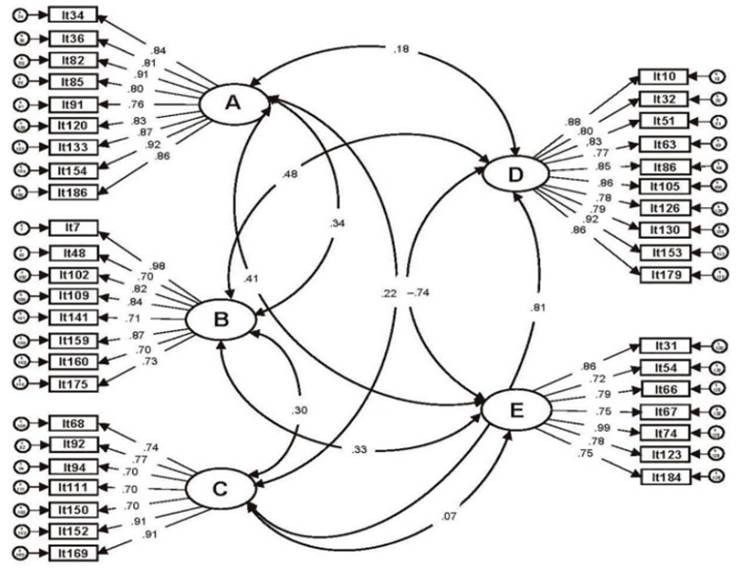
Figure 2 . Standardized coefficients of the confirmatory factor analysis of the traits subgroup scales
In the analysis of convergent and discriminant validity of the scales within their own groups (objective 6), carried out through five CFAs, the adjustment indices were previously verified, finding adequate values: χ²/df = 2 to 3, CFI > .96, TLI >.93, RMSEA > .07, and SRMR < .04 (Boateng et al.,2018; Collier, 2020). It was also found that the AVE of a CFA series of the scales of its corresponding group was greater than .50, evidencing convergent validity (Bello, 2016). It was also noted that √AVE was higher than Pearson’s r correlations, with the other scales of its group confirming discriminant validity of these scales (Ab Hamid et al., 2017; Bello, 2016). Once non-normality (KS/SW ≥ .09) and homoscedasticity (χ 2 ≥ .26) in most of the scales by groups of crime type and age range were confirmed, a series of comparison tests were analyzed in each IDPI-11 scale for these groups (objective 7). Most of the scales showed significant differences between crime groups (H ≥ 32.97, p-value ≤ .001), although with low effect sizes (ε2 < .39). Similarly, most of the scales showed significant differences between age groups (U < 131 331.50, p-value < .05), as well as low effect sizes (rg < .40) (Frey, 2022; King et al., 2018; Stikker, 2018 ).
In the analysis of criterion validity of Personality group scales (objective 8), it was found that the IDPI-11 personality group scales were capable of significantly explaining -according to the thresholds of some authors (Frey, 2022; Sperandei, 2014)- the probability of belonging to the group of inmates with crimes against public health and property, in addition to crimes against liberty, and against life, body, and health (R2 between .52 and .68 with predictors OR between 1.02 and 1.98, p-value ≥ .21). Likewise, these scales can predict that the prison security level imposed will increase: R2 = .53 to .61 with β predictors between 1.16 and 1.97, p-value ≥ .008 (Ali & Younas, 2021; Frey, 2022), as shown in Table 3.
Table 3 Predictive logistic and linear models of the Personality group scales
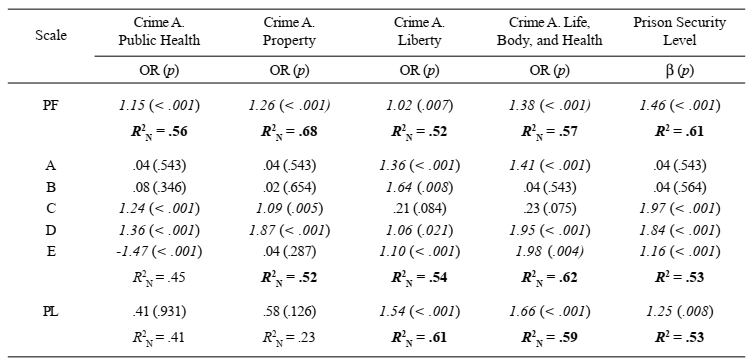
Note: n = 1095; OR = odds ratio; β = beta (standardized regression coefficient); p = significance value; R2 = coefficient of determination of the linear regression; R2 N = Nagelkerke’s pseudo-R2 coefficient of determination of the logistic regression; PF = Functioning; A = Serenity vs. Negative Affectivity; B = Humanity vs. Detachment; C = Integrity vs. Dissociality; D = Moderation vs. Disinhibition; E = Psychological Flexibility vs. Anankastia; PL = Borderline Pattern. Only four types of crimes were used for the regression models because there were morethan 50 cases. The coefficients of determination of the models that explain a variance of the dependent variable greater than .05 are shown in bold. The significant regression coefficients (OR or β) of these models are in italics.
Finally, McDonald’s omega (ω) was used to analyze the internal consistency of the IDPI-11 scales (objective
9), and the results showed adequate internal consistency indices for the scales of the Response Styles group: Dissimulation (ω = .88) and Simulation (ω = .90). However, the only scale that obtained a low level in this group was Inconsistency (ω = .12) (Olivas-Ugarte & Cipriani-Delgado, 2022). Similarly, considering the criteria of Olivas-Ugarte & Cipriani-Delgado, 2022, the internal consistency of the other scales of the Mental Health Factors (ω =.76 to .94), Psychopathology (ω =.73 to .95), Personality (ω = .77 to .95), and Trait Facets (ω = .74 to .96) obtained appropriate levels of internal consistency. See Table 4.
Table 4 Mean, standard deviation, range, and McDonald’s omega of the IDPI-11 scales
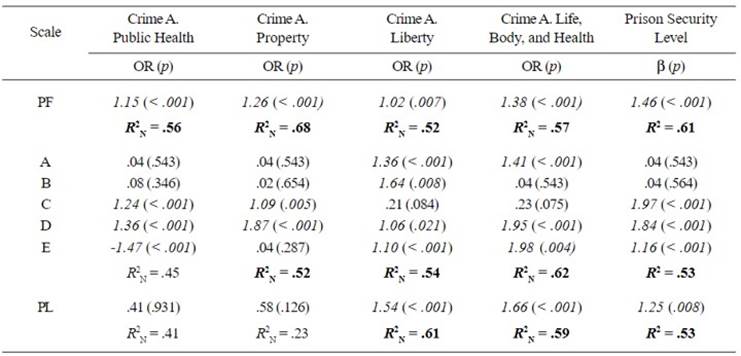
Note: M = mean; SD = standard deviation. Group names are shown in bold and subgroups names, in italics. a Invalidity (scale R1) was excluded from the analyses.
Normalization. After the process detailed in Appendix F, the development of norms for the raw scores of the IDPI-11 scales (objective 10), the UT scores generated for the scales of the Risk Factors subgroup, as well as for the scales of the Psychopathology, Personality and Trait Facets groups, managed to obtain common percentile scores that facilitate the differential diagnosis (Ben-Porath & Tellegen, 2020a). So, uniform PTs of 30, 35, 40, 45, 50, 55, 60, 65, 70, and 75 were equivalent to percentile score (Pc) of < 1, 12, 14, 41, 61, 73, 80, 87, 98, and > 99, respectively.
Discussion
The eleventh revision of the ICD has recently entered into force and is ready to be implemented by member states, continuing the tradition of having a global scope and being applicable in diverse cultures and settings, with a focus on primary care in underdeveloped countries unlike the DSM (Halliwell, 2021). One of the sections of this diagnostic system addresses personality disorders and related traits, a model that promises to correct the errors of its predecessor, which was criticized by the scientific community for being less restrictive and allowing greater specificity. Some measures have been developed based on this model to obtain adequate psychometric levels with a few exceptions. The limitations of these results mainly emerge from the methodology, the samples used, and the manner in which their manuals are specified. In fact, its application in prison settings and underdeveloped countries has not yet been rigorously evaluated. Therefore, in this research a comprehensive measure of personality based on the ICD-11 PD model was developed, considering an extensive theoretical, practical, and methodological foundation that favors the best evaluation of the construct in the Peruvian correctional system.
The item-level results supported the psychometric properties of each IDPI-11 scale. It was expected that the factor loadings of the items with their original scales would be higher than those of their target scales as found in the development of the MCMI-IV (Millon et al., 2015). Likewise, for the most part, both the IRT parameters of discrimination and those of difficulty reflected a good ability to differentiate and detail the levels of constructs measured through the probability of their support. In addition, it was found that in this study, as in Medina (2021), depressiveness and distrust, in addition to other characteristics and symptoms, are frequent in the criminal population. In this sense, it is key to make decisions to identify appropriate personalized and group treatments to reduce or control the exacerbation of these dysfunctional indicators in convicts.
The findings showed that the developed instrument had an adequate level of construct validity and an external criterion for the scales of the IDPI-11 Traits subgroup. These results are similar to those found in the construction of the Personality Inventory for the DSM-5 - Brief Form Plus (PID5-BF+), a multinational study (Bach et al., 2020) in which the structural validity demonstrated a CFI above .95 and an RMSEA below .06. Additionally, the instrument traits were correlated with personality constructs evaluated through interviews (r > .17). They were also similar to the optimal results of the research carried out by Oltmanns and Widiger (2018) in the USA for the construction of the Personality Inventory for the ICD-11 (PiCD): CFI = .77 to .83, TLI = .76 to .82, RMSEA = .10 to .11, and SRMR = .10 to .11. However, they are different from those found in the Spanish validation of the instrument because their data did not fit the CFA model (Gutiérrez et al., 2021).
The external criteria validity results found in this research are similar to those obtained in a study conducted in Italy (Ferretti et al., 2021 ; First et al., 2018), in which high irresponsibility (disinhibition) and high restricted affectivity (detachment) predicted child sexual abuse (β = -.60 and β = -1.17, respectively), and irresponsibility (disinhibition) predicted crimes against the person (β = .017). Similarly, it is partially similar to a study carried out in Huancayo, Peru, with child sexual abuse offenders (Medina, 2021), in which high prevalence of an obsessive-compulsive clinical pattern (anankastia) was found (41.5%). However, contrary to the results of this study, the dependent clinical pattern (negative affectivity) was more prevalent in this population, and was also shown as the second most prevalent (24.5%). This divergence may be due to the descriptive design used and certain shortcomings that prevented the instrument to be adapted to the Peruvian context. Similarly, it has been shown that psychopathy in its two variants (primary and secondary) is related to recidivism (Alonso et al.,2021), which is an important component in the designation of the prison security level in the Peruvian system.
In this study, the IDPI-11 personality scales were correlated with prison criteria, as they are of greater practical relevance (Day & Cook, 2019). Psychopathy and PD are closely linked, since several five-factor traits overlap with the psychopathic personality variants. Certainly, psychopathic traits are the main predictor of recidivism. Nevertheless, comorbidity of ASPD and BPD, in addition to substance use, predicts the commission of violent crimes (McMurran & Howard, 2019). It was also fou nd that offenders with high levels of substance use and attention-deficit/hyperactivity disorder (ADHD) are related to theft, and those who commit matricide are generally people with psychosis (Davison & Janca, 2012 ; David et al., 2018).
Therefore, it is important to understand that any link between personality disorders and violence must be viewed within the context of a link between general mental disorders and violence. The more psychopath characteristics people have, the better prognosis they will have to feel distressed (Stricker & Pietrowsky,2022 ) when committing a crime, attacking people, or attacking themselves, as shown by the significant predictive capacity of the Functioning and Borderline Pattern scales in most prison criteria. In this situation, it is advisable to assess the protective attributes and positive tendencies (traits) of personality as proposed by the IDPI-11 scales. This proposal is based on the evidence of successful studies of salutogenic perspectives that support the importance and necessity of evaluating positive personality in a penitentiary center (see, e.g., Miner, 2021; Pasowicz & Piotrowski, 2021). This will make it possible to identify the protective factors and strengths of the inmates. This, through the «High» levels of the scales of the Protective Factors subgroup (T score ≥ 65) and the «Positive» levels of the Functioning scale, the Traits subgroup scales, and the Facets of Traits group scales (T score = 20 to 34). Such evaluation can help determine the goals, plan and supervise the treatment, to help them «give back» to others and provide them with opportunities to participate in daily community life (Levak et al., 2011).
The reliability findings of each of our scales are similar to those found in the development of the MCMI-IV (Cronbach’s α ≥ .63; see Millon et al., 2015) and MMPI-3 (Cronbach’s α ≥ .69 in the Reestructured Clinical (RC) Scales; see Ben-Porath & Tellegen, 2020b). Furthermore, the low reliability of Inconsistency (R2 scale) is similar to a study carried out in Ohio, USA, on the Combined Response Inconsistency (CRIN scale) of the MMPI-3 (Cronbach’s α = .27), due to the willingness of examinees to do the evaluation (Whitman & Ben- Porath, 2021).
Finally, standardized scores were designed for the IDPI-11 scales, depending on the bias of their distribution and/or the nature of their conception. Moreover, it is necessary to mention that the UT score of Functioning (PF scale) needs to be modified using the Intensity/Comorbidity (IC) adjustment in order to increase the specificity of this scale. This is because the comorbidity of several maladaptive personality traits/types, followed by the severity itself that can exist in a single maladaptive personality trait/ type, usually causes deterioration of personality functioning (Tyrer et al., 2019). For more information on the IC adjustment see Appendix G.
How does IDPI-11 fill the gap?
The rationale of this research is derived from the potentialities previously expressed in the ICD-11 PD model for evidence-based psychological assessment (EBPA). Theoretically, focusing on the actor self allows a better understanding of the personality dynamics of the prison population, conceptualizing current behavior (clinical diagnosis), determining the reason for it (etiological diagnosis), and understanding the early interaction and the current result between nature and nurture in this type of population. Likewise, focusing on the agent self, it is possible to understand how psychosocial factors and the manifestations of mental disorders in the prison population influence each other or whether they influence the maintenance of the personality disorder, thus enriching the theoretical basis of differential diagnosis.
From a practical point of view, by emphasizing the author self, the developed instrument contributes to the detection, diagnosis, and prognosis of PD and other psychopathological conditions; by emphasizing the agent self, group treatment programs can be planned and evaluate the dynamic (current) risk and protection factors of the group; and by emphasizing the author self, individual treatment programs can be established, taking into account the dynamic and static (historical) risk and protection factors, and the way in which the inmate himself understands all of this to fit in society by finding the meaning of life and causing rehabilitation to last over time (see Appendix I for evidence-based treatment for the IDPI-11 scale profiles). These benefits are important since, to date, most evaluations for classification and treatment at the HP have been guided more by psychologists criteria than by a measuring instrument. With the profile obtained from the IDPI-11 measurement, which can be even shown by codes and not with the full names of the scales, and following an interpretation based on a measurement theory, it is possible to avoid mentioning the «label» (stigma) of the clinical conditions and instead reveal a comprehensive look towards the possible reason for the current state of the inmate (Grossman, 2019). This subtle way of translating and reporting the results promotes the development of empathy and a therapeutic alliance with the inmate, thereby obtaining better results in rehabilitation programs.
Limitations and future directions
This study is not exempt of limitations. One of the limitations was in the sample (n = 60) used for meeting the objective of study 1: item selection analysis from the IRT. At that time, there was no adequate statistical guide; therefore, the assumptions and minimum size necessary for the analysis were not evaluated. However, this was not evidenced and did not affect study 2, since adequate psychometric levels were evidenced. This may be due to the fact that the analysis with the IRT of the items in step 6 was accompanied by the analysis of the difficulty and discrimination indices of the CTT. In addition, the guidelines for establishing sample size and test length assumptions to obtain precision in IRT modeling have been determined based on simulated studies and not with real test data. In this sense, there are -although few- accuracy studies of dichotomous items in small samples of up to 50 participants with test lengths of up to 10 items (Sahin & Anil, 2017).
Another limitation was that the selected sample, although large and randomized, was only collected in a single center, and was mostly made up of inmates from the Peruvian highlands. For this reason, more validation and adaptation studies are still needed in other correctional contexts and they must also be carried out with populations of women and adolescents to further generalize its adequate psychometric properties. A possible bias in the evaluation with the IDPI-11 may be caused by professionals underqualified for this type tasks. An advanced level of expertise; extensive knowledge; and practice in personality, psychopathology, epidemiology, clinical health psychology, and correctional psychology are needed for adequate interpretation of the profile of the examinee. If this is achieved, professionals will have a valuable tool for their evaluation and treatment practices.
An additional limitation was the failure to identify cut-off points through semi-structured interviews for the IDPI-11 scales due to the restrictions generated by the COVID-19 pandemic. Consequently, the thresholds for interpretation were designated intervals based on the statistical distribution of the scores on each scale, which was supported by a dimensional rather than a categorical one. Professional users must consider an error threshold for interpretation, whether this is greater or less than that obtained by the examinee on the Psychopathology and Personality scales (Grossman, 2017): the IDPI-11, like all psychological measurement instruments in general, does not perfectly predict the specific level where a person is located; and, to make better decisions, all the available information must be evaluated. Diagnostic accuracy studies for this measure are . Professional users must consider an error threshold for interpretation, whether this is greater or less than that obtained by the examinee on the Psychopathology and Personality scales (Grossman, 2017): the IDPI-11, like all psychological measurement instruments in general, does not perfectly predict the specific level where a person is located; and, to make better decisions, all the available information must be evaluated. Diagnostic accuracy studies for this measure are needed to be applied in clinical settings; however, in the absence of similar instruments, IDPI-11 can be used with caution in populations with similar characteristics, as suggested by Millon et al. (2015).
Despite its limitations, the developed instrument, whose scales are generally valid and reliable, has many potential applications in the practice of the psychologist in the penitentiary context as follows:
(a) For mental health purposes, because IDPI-11 has been developed primarily to provide a comprehensive assessment of the inmate’s personality and associated mental health factors for mental health purposes, such as detection (as primary health care), diagnosis, prognosis, case conceptualization, treatment monitoring, and treatment follow-up (as secondary health care), as previously explained in the introductory section (Day & Cook, 2019). It can also be used for evaluating the predisposition of any mental illness in healthy inmates, for stratifying the risk of mental illness in inmates with risk factors (primary health care), for staging the severity of general mental disorders that inmates already have (as secondary health care), for monitoring inmates’ chronic mental disorders, and for surveilling the remission or recurrence of the prisoners’ chronic mental disorders (tertiary health care) (see Deeks & Bossuyt, 2021).
(b) For legal purposes as in competency to stand a trial, criminal responsibility, dangerousness, pre- sentence, risk, and recidivism assessments.
For competency to stand a trial assessments, Simulation (scale R4) can help verify the intention of over-reporting psychiatric symptoms after ruling out a significant increase in the score of Schizophrenia Spectrum (scale S7) (see Ben-Porath et al., 2022; Butcher et al., 2015). For criminal responsibility assessments, it is also important to identify if there is a significant elevation in the R4 and S7 scales to better understand said responsibility, and it is also essential to verify if the items of infrequent symptoms of memory problems that make up the R4 scale (ítems 23 and 128) have been supported (Butcher et al., 2015; Sellbom et al., 2022). For dangerousness assessments, the elevations of the Patience vs. Anger (a.4 scale), Prudence vs. Temerity (d.1 scale), and Commitment vs. Irresponsibility (d.2 scale) should be determined to carry out its pertinent transfer to civil or forensic units.
For pre-sentence assessments, the «present» and «prominent» levels of any of the scales of the Psychopathology group and the mild, moderate or severe level of Functioning (PF scale) can provide guidelines for decision-making on the main mental health needs, as well as the «positive» levels of the Prudence vs. Temerity (d.1 scale), Commitment vs. Irresponsibility (d.2 scale), and Planning vs. Disarray (d.3 scale), to inform the judge about the mitigating factors that may be considered for the sentence.
For risk assessments, it is appropriate to assess the «present» and «prominent» levels of the Patience vs. Anger (a.4 scale) and Kindness vs. Aggressiveness (c.3 scale). And, finally, for recidivism assessments, the «positive» levels of the sociability scale (b.1 scale) -common in sociopaths (see «The mask of sanity»; Patrick, 2018)- for hetero aggression, «medium» or «high» level of Suicidal Tendency (F6 scale) for self-aggression, and the «present» and «prominent» levels of the scales can be assessed (S3, a.4, b.2, c.1, c.2, c.3, d.1, d.2, d.3, and d.4). It can also be considered the type of crime committed, such as crimes against property and public order, since they are commonly associated with recidivism and therefore require a higher level of prison security level.
Another strength is that the IDPI-11 scales conform to current health standards. As a result, correctional psychologists, prison inmates, and researchers who use the IDPI-11 will be able to obtain an evaluation tool, pertinent care, and an appropriate methodological resource, respectively. In the global context, a comprehensive instrument has not yet been built and, at the same time, adjusted to the ICD-11 PD model. In the Latin American context, to date, no comprehensive instrument has been developed to measure personality. In this sense, it is expected that the authorities of the Peruvian prison system can incorporate this instrument into their guidelines for the evaluation and treatment of convicts. Finally, it will be of great theoretical and practical benefit that future studies evaluate the IDPI-11 scales from network psychometry, an emerging and promising methodology for exploring the direct and integral dynamics of observed variables (nodes) without the direct influence of latent constructs (see e.g., Christensen et al., 2020; Isvoranu et al., 2022; See et al., 2020).
We conclude that the IDPI-118 is a valid and reliable measure for determining HP inmates’ personality from the model proposed in the ICD-11 nosology, since it provides a vast theoretical foundation from the integration of the models with greater scientific support and is a EBPA tool for the purposes of the primary, secondary, and tertiary mental health care, and for the guidance of legal decisions by the authorities of the Peruvian correctional setting.
Conflicts of interest
The authors have no conflicts of interest to declare.
Ethical responsibility
This study did not involve human or animal experimentation, and informed consent was obtained from the inmates and witnesses. To ensure the confidentiality of the data, consent was administered separately with the protocols, eliminating all forms of identification. Likewise, the study does not detail any individual data on prisoners’ responses or any data that allows their identification. This study was reviewed and approved by the Research Ethics Committee of the Faculty of Humanities (CEI-DD- HH) of Continental University.
Authors’ contributions
LMHO, DNRC, and RMCA conceived the article; DNRC and PAAV collected the data; LMHO and PAAV performed the statistical analysis; LMHO reviewed the contents; and RMCA advised on the entire study and manuscript preparation process.
Acknowledgment
The authors thank Dr. Luis Centeno Ramírez for his recommendations regarding the statistical analysis of the data. We also thank Mike J. Crawford, M. D., Laura Weekers, Ph. D., and Joshua R. Oltmanns, Ph. D., who shared their instruments of personality psychopathology which served us as a guide for the construction of the IDPI-11.
Note:
1 It was originally mentioned as «social desirability bias» (Morgado et al., 2017); however, the term has been modified to encompass the entire spectrum of distorted responses.
2 The factor analysis approach from the CTT and IRT approach is used not only for the refinement of the measure but also for complementing the structural validity (internal structure) of the scales to report the individual properties of each item in the evaluation phase (Bach, Brown, et al., 2021; Nguyen et al., 2014).
3 Some authors consider norms development as part of the evaluation phase of the measure (see, e.g., Boateng et al., 2018). In study 2 it is placed as an independent section for its better understanding.
4 It is important to emphasize that the IDPI-11 has two types of scales: (a) «solid scales,» which have their own items (prototype items) and items from other scales (non-prototype items), and (b) «floating scales,» which only have items from other scales (non-prototype items). The responses to the items are recorded in a dichotomous format F = 0 and T = 1 which, at the time of scoring, are configured in two types of items (prototype item = 2 points, non-prototype item = 1 point), as they correspond to each of the IDPI-11 scales.
5 We chose different cut-off points for factor loadings at different objectives, either for EFA or CFA, due to development or evaluation purposes and the theoretical importance of the desired latent factors.
6 Although this procedure originally corresponded to Wiggins (1973, as cited in Williams et al., 2019), Millon et al. (2015) modified it to give differentiated scores to the items according to their prototypicity within a scale, thus reducing the overlap between these scales and, at the same time, increasing the internal consistency of the scales without the need to add more items to the measure.
7 Dissimulation (scale R3) and the scales of the Protective Factors subgroup (Healthy Habits (scale F1), Self-Esteem (scale F2), Meaningful Activities (scale F3), and Openness to Treatment (scale F4)) have a direction towards health. The scales of the Validity Indices subgroup (Invalidity (scale R1) and Inconsistency (scale R2)) do not have a specific direction. And the rest of the scales follow a direction towards psychopathology.
This study is based on the thesis:
Hualparuca, L. M., Ramos, D. N., & Arauco P, A. (2021). Construcción y validación del Inventario Dimensional Integrativo de la Personalidad-11 (IDIP-11) en internos del Establecimiento Penitenciario de Huancayo (Construction and Validation of the Dimensional Integrative Personality Inventory-11 (IDIP-11) in inmates of the Huancayo Penitentiary Establishment). (Bachelor’s degree thesis). Continental Institutional Repository. https://hdl.handle.net/20.500.12394/10507