Serviços Personalizados
Journal
Artigo
Indicadores
-
Citado por SciELO
Links relacionados
-
Similares em SciELO
Compartilhar
Industrial Data
versão impressa ISSN 1560-9146versão On-line ISSN 1810-9993
Ind. data vol.26 no.2 Lima jul./dic. 2023 Epub 20-Dez-2023
http://dx.doi.org/10.15381/idata.v26i2.24930
Production and Management
Economic Growth and its Influence on Fatal Occupational Accidents in Peru
1Master’s degree in Industrial Engineering. Currently working as professor at Universidad Nacional Mayor de San Marcos and Universidad Tecnológica del Perú (Lima, Peru). E-mail: pgutierrezf@unmsm.edu.pe
This study aims to determine how economic growth influenced fatal occupational accidents in Peru from 2011 to 2019. Data from the 24 departments, grouped into 4 macro-regions, were used and an econometric analysis using panel data was applied. This analysis consisted of assessing the stationarity of the data, and through first generation unit root tests, it was determined that they are integrated of order one. Likewise, it was found that there is a cointegration relationship between the variables (long-run equilibrium) by Pedroni panel cointegration means testing. Finally, the regression coefficients were estimated using the DOLS (Dynamic Ordinary Least Squares) estimator. It was determined that economic growth has a direct proportional relationship with fatal occupational accidents; that is to say, economic boom would cause fatal occupational accidents to increase.
Keywords: economic growth; occupational accidents; economic factors; occupational safety and health
INTRODUCTION
In Aristotle’s philosophy, occupational accidents were conceptualized as something that had no cause per se, that did not fit into the broader metaphysical scheme and from which no meaningful knowledge could be obtained (Loimer & Guarnieri, 1996). Over the centuries, the concept of occupational accident has developed and at present there are various definitions that can be summarized as follows: an occupational accident is an unplanned event directly related to work activities that causes injury or even death to the worker. Based on this, the premise ‘all work is dangerous’ takes on special relevance, since it becomes impossible to consider any work as safe (Gómez-Pineda, 2014). Due to this, there is a need for employers to adopt occupational safety and health measures to prevent the occurrence of occupational accidents. In this regard, it has been found that there is still a need to strengthen this matter in Peru since the subsidies for temporary disability due to occupational accidents increased from 0.20% of the Gross Domestic Product in 2011 to 0.58% in 2013 (Cossio, 2016) and the time lost due to occupational accidents during the period 2014-2016 is equivalent to 7083 workers with “zero productivity” per year (Pahuacho, 2017).
Occupational safety and health in organizations is affected by internal factors (like safety climate and the management system) and external factors (such as technological advances and economic, social and political factors) (Gümüş & Gülsün, 2020; Chang et al., 2018). Given this, the need to prioritize prevention as a tool for the reduction of occupational accidents increases (Teixidó, 2009), as deficient practices in occupational safety and health cost approximately 4% of global Gross Domestic Product each year. Theories have been proposed to predict the causes of occupational accidents, but none is unanimously accepted (Organización Internacional del Trabajo, 1998). Moreover, several models have also been proposed for investigating occupational accidents (Surry, 1971) that are not incompatible with each other. Instead, each model emphasizes different aspects. It should be noted that these theories and models of occupational accidents make it possible to explain how internal factors that are under the employer’s responsibility contributed to the occurrence of occupational accidents so that the employer implements corrective measures to prevent their recurrence.
Among the external factors that affect occupational safety and health, economic growth is a topic of great interest given its impact on the welfare of a country’s population because of a sustained increase in income (Jiménez, 2011). Economic growth is the quantitative expansion of income and value of services and final goods produced in an economic system in a given period of time (Enriquez, 2016). Therefore, economic growth is a requirement for economic development (Loayza & Soto, 2002), since it generates the resources needed.
Kossoris (1938) conducted the first studies that addressed the occurrence of occupational accidents during times of economic expansion, finding a procyclical relationship between accidents and employment in the manufacturing sector of the United States. Subsequent studies have shown that business cycle indicators exhibit procyclical behavior with occupational accidents, meaning that accidents increase during economic booms and decrease during recessions. (Kim & Park, 2020; Contreras et al., 2019; Łyszczarz & Nojszewska, 2018).
Other studies concluded that a close direct and proportional relationship exists between increased economic growth and occupational accidents (Gümüş & Gülsün, 2020; Ovejero et al., 2018); however, other studies point to the existence of a negative relationship (Kahraman et al., 2019; Mouza & Targoutzidis, 2012).
The Instituto Nacional de Seguridad e Higiene en el Trabajo [National Institute of Workplace Safety and Hygiene] (2014) states that in countries where the national occupational safety and health system is well designed and managed, the strength of the relationship between economic growth and occupational accidents is expected to be inversely proportional to the intensity of the national preventive effort. The same result would have been expected in Peru with the enactment of an occupational safety and health law (Ley N.° 29783, 2011), but results presented by Cossio (2016) and Pahuacho (2017) identified that the Peruvian national system still requires improvement.
That being said, several studies have been conducted in developed and high-income countries in Europe, Asia, and North America that agree that economic factors have an impact on the occurrence of occupational accidents (Kim & Park, 2020; Contreras et al., 2019; Łyszczarz & Nojszewska, 2018; Gümüş & Gülsün, 2020; Ovejero et al., 2018; Kahraman, et al., 2019; Mouza & Targoutzidis, 2012). Therefore, the question arises as to whether this same behavior is evident in developing countries. Considering that, in Peru, the national occupational safety and health system is still unable to protect workers from occupational hazards, this study seeks to determine how economic growth influences fatal occupational accidents in Peru In this way, the study attempts to contribute to government bodies for the development of policies that support to the intent of Law No. 29783, which is the promotion of a culture of risk prevention, a progressive reduction in the loss of the Gross Domestic Product and the improvement of productivity and competitiveness of companies and the country.
METHODOLOGY
This study adopts an applied research methodology with a quantitative approach and a non-experimental and cross-sectional design, characterized by a causal and explanatory nature. It seeks to determine the influence of economic growth on fatal occupational accidents in Peru.
To conduct the study, an econometric analysis using panel data was applied in line with other research with similar objectives (Kahraman et al., 2019; Łyszczarz & Nojszewska, 2018) because it makes it possible to estimate more realistic models compared to single-period cross-sectional data and single-entity time series.
Fatal occupational accidents were established as the dependent variable, considering that their reporting is more frequent than non-fatal accidents (Çolak & Palaz, 2017). Accident rates were not used because accident rates are affected by the increase in workers, even if there is no change in the number of accidents (Kim & Park, 2020).
Economic growth, measured through Gross Value Added, was established as the independent variable. Other economic factors were included as proxy variables that are not the main focus of the study: activity rate, private sector companies, private sector workers, and average remuneration of private sector workers.
Annual data from the 24 departments of Peru during the period 2011-2019 were used to identify the influence of economic growth on occupational accidents. These data were grouped into 4 macro-regions (Table 1) because the statistical yearbooks of the Ministry of Labor and Employment Promotion do not have recorded occupational accidents in some departments, such as Amazonas, Huánuco, Madre de Dios and San Martín. The lack of records of occupational accidents might be attributed to problems related to underreporting (Mejía et al., 2015).
The data on the number of occupational accidents and the monthly average of companies, workers and remuneration in the private sector are obtained from the statistical yearbooks prepared by the Statistics Office of the Ministry of Labor and Employment Promotion (MTPE), based on the records of the Occupational Accident System (SAT) and the Electronic Payroll (PLAME) systems. In addition, available data on Gross Added Value and Activity Rate published by the National Institute of Statistics and Informatics (INEI) were used.
Table 1 Macro-Regions of Peru.
Macro-Region | North | Central | South | East |
---|---|---|---|---|
Department | Cajamarca La Libertad Lambayeque Piura Tumbes | Ancash Ayacucho Huancavelica Huánuco Ica Junín Lima* Pasco | Apurímac Arequipa Cusco Madre de Dios Moquegua Puno Tacna | Amazonas Loreto San Martin Ucayali |
(*) Lima Department includes Metropolitan Lima, Lima’s Provinces, and the Constitutional Province of Callao.
Source: Prepared by the author.
RESULTS
Descriptive Statistics
Table 2 presents the descriptive statistics of the panel data. It is observed that the standard deviation results are high for all variables, meaning that there is a high variability of the data, which raises, the suspicion of asymmetric or atypical data. Graphical representations were made to identify heterogeneity between macro-regions and heterogeneity over time. For this purpose, the values of the variable fatal occupational accidents were transformed into natural logarithms because of the presence of asymmetric values in the sample. Figure 1 shows that the Central Macro-region shows the highest mean of fatal occupational accidents during the period 2011-2019 but presents less dispersed values. On the other hand, Figure 2 shows that the mean of fatal occupational accidents in the macro-regions increased throughout 2011-2019. Moreover, it is observed that every year one macro-region presents a higher number of fatal occupational accidents than the others. In accordance with Figure 1, it is known that this is the Central Macro-region. Therefore, it can be affirmed that, in all years, the number of occupational accidents in the Central Macro-region is significantly higher than in the other macro-regions.
Table 2 Descriptive Statistics: Macro-Regions.
Mean | Standard Deviation | Minimum | Maximum | |
---|---|---|---|---|
Dependent Variable | ||||
Fatal Occupational Accidents | 44.89 | 50.63 | 1 | 142 |
Independent Variable | ||||
GVA at Constant Prices in 2007 (in millions of soles) | 109 530.80 | 101594.80 | 17690 | 314 497 |
Proxy Variables | ||||
Activity Rate | 72.63 | 3.82 | 66.04 | 78.46 |
Monthly Average Number of Private Sector Companies | 66 909.97 | 67 324.20 | 9894.00 | 201 718.00 |
Monthly Average Number of Private Sector Workers (in thousands) | 793.28 | 936.48 | 64.47 | 2633.14 |
Monthly Average Compensation of Private Sector Workers | 1774.89 | 389.86 | 1163.38 | 2574.34 |
N = 36 (total sample), n = 4 (macro-regions), t = 9 (years).
Source: Prepared by the author. Data from MTPE and INEI processed by Stata version 15.
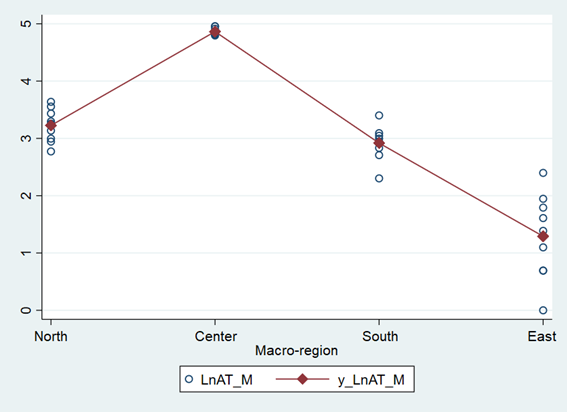
Figure 1 Heterogeneity Among Fatal Occupational Accidents in the Macro-Regions, 2011-2019 (Stata version 15).
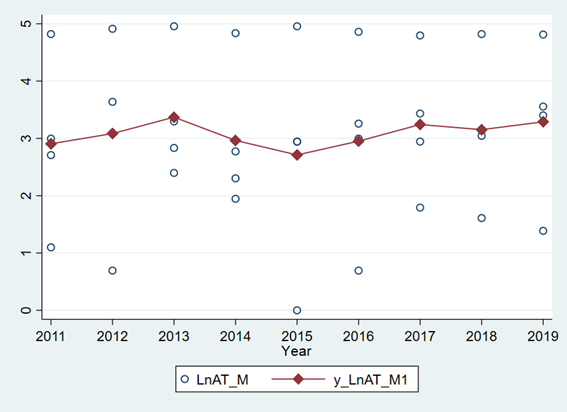
Figure 2 Heterogeneity of Fatal Occupational Accidents Over the Years., 2011-2019 (Stata version 15).
Econometric Analysis
Regression Model
The econometric analysis of panel data allows for the study of the relationships between different variables by combining a time dimension with another cross-sectional dimension; this provides greater benefits when making statistical inferences.
A panel data model where the number of time observations (t = 1, 2, 3, 4, 5, 6, 7, 8, 9 years) is larger than the number of cross-sectional observations (i = 1, 2, 3, 4 macro-regions) was proposed:
Where:
Ln(AT_M)it |
Natural logarithm of Fatal Occupational Accidents of the macro-region (i) in the year (t) |
Ln(VAB)it |
Natural logarithm of Groos Value Added of the macro-region (i) in the year (t) |
Ln(Tact)it |
Natural logarithm of Activity Rate of the macro-region (i) in the year (t) |
Ln(EMP)it |
Natural logarithm of Monthly Average Number of Private Sector Companies of the macro-region (i) in the year (t) |
Ln(TRAB)it |
Natural logarithm of Monthly Average Number of Private Sector Workers of the macro-region (i) in the year (t) |
Ln(REM)it |
Natural logarithm of Monthly Average Compensation of Private Sector Workers of the macro-region (i) in the year (t) |
ꞵ0 |
Constant that collects the deterministic components, such as the intercept |
ꞵ1, ꞵ2, ꞵ3, ꞵ4, ꞵ5 |
Regression coefficients (elasticities) |
αi |
Individual effect (unobserved) of each macro-region |
ɛit |
Identically distributed independent error term |
The variables included in the panel data models were expressed in natural logarithms (Łyszczarz & Nojszewska, 2018) and allowed the following:
Interpret regression coefficients as constant elasticities. This means that if Y is a dependent variable and X is an independent variable, the elasticity of Y with respect to X presents a percentage change in Y for a given percentage change in X.
The indicators are expressed on a similar scale, highlighting the linear relationship between them.
Unit Root Tests
The stationarity of the variables’ indicators was evaluated to avoid a possible incidence of spurious regression when using non-stationary time series. For this purpose, the first-generation unit root tests Levin-Lin-Chu, Harris-Tzavalis, Breitung, Im-Pesaran-Shin, and Hadri were applied. These tests for panel data are based on the tests for time series but have the advantage of combining time series and cross-sectional data, thus, obtaining more degrees of freedom, improving the properties of the estimators, and also correcting for unobserved heterogeneity.
The first-generation unit root tests have the following assumptions:
H0: All panels contain a unit root.
H1: Some panels are stationary.
Confidence level (α): 5%
Hadri’s Lagrange multiplier test has the following hypotheses:
H0: All panels are stationary.
H1: Some panels have unit roots.
Confidence level (α): 1%
Table 3 shows the results of the five unit root tests. At 5% significance, the null hypothesis of the Levin-Lin-Chu, Harris-Tzavalis, Breitung, and Im-Pesaran-Shin tests cannot be rejected. On the other hand, at 1% significance, the null hypothesis can be rejected by the Hadri test. Therefore, these results indicate that the time series have a unit root.
Table 4 presents the results of five unit root tests applied to the series, differentiated once, to corroborate the order of integration of each time series and to rule out the existence of more than one unit root. At 5% significance, the null hypothesis of the Levin-Lin-Chu, Harris-Tzavalis, Breitung, and Im-Pesaran-Shin tests can be rejected. In contrast, at 1% significance for the Hadri test, the null hypothesis cannot be rejected. Therefore, these results indicate that they do not have a unit root, so they are stationary.
Results from the tests applied to the variables in the panel data model (Equation 1) indicate that these series are integrated of order one. This occurs when the first difference of a non-stationary series is stationary. The series that is integrated of order one is called I(1).
Table 3 Unit Root Tests Results (variables in levels).
Variable | LLC | HT | Breitung | IPS | Hadri LM | |||||
---|---|---|---|---|---|---|---|---|---|---|
Statistic | p-value | Statistic | p-value | Statistic | p-value | Statistic | p-value | Statistic | p-value | |
Ln(AT_M) | −4.068 | 0.000 | 0.010 | 0.000 | −2.630 | 0.004 | −1.414 | 0.079 | −0.762 | 0.777 |
Ln(VAB) | −1.400 | 0.081 | 0.855 | 0.848 | 2.921 | 0.998 | −0.367 | 0.357 | 4.857 | 0.000 |
Ln(Tact) | −4.033 | 0.000 | 0.630 | 0.321 | −0.408 | 0.342 | 0.076 | 0.530 | 2.624 | 0.004 |
Ln(EMP) | −1.183 | 0.119 | 0.811 | 0.770 | 3.150 | 0.999 | −2.232 | 0.013 | 5.036 | 0.000 |
Ln(TRAB) | −9.993 | 0.000 | 0.763 | 0.662 | 2.020 | 0.978 | −1.244 | 0.107 | 4.482 | 0.000 |
Ln(REM) | −7.851 | 0.000 | 0.784 | 0.711 | 2.731 | 0.997 | −2.370 | 0.009 | 4.692 | 0.000 |
Source: Prepared by the author. Unit root tests performed with Stata version 15.
Table 4 Unit Root Tests Results (first differences).
Variable | LLC | HT | Breitung | IPS | Hadri LM | |||||
---|---|---|---|---|---|---|---|---|---|---|
Statistic | p-value | Statistic | p-value | Statistic | p-value | Statistic | p-value | Statistic | p-value | |
ΔLn(AT_M) | −8.586 | 0.000 | −0.173 | 0.000 | −2.488 | 0.006 | −2.184 | 0.015 | −1.152 | 0.875 |
ΔLn(VAB) | −4.303 | 0.000 | −0.084 | 0.000 | −1.126 | 0.130 | −1.823 | 0.034 | −0.223 | 0.588 |
ΔLn(Tact) | −3.900 | 0.000 | 0.045 | 0.000 | −2.409 | 0.008 | −1.065 | 0.143 | 0.831 | 0.203 |
ΔLn(EMP) | −2.204 | 0.014 | 0.003 | 0.000 | −0.191 | 0.424 | −2.645 | 0.004 | 1.854 | 0.032 |
ΔLn(TRAB) | −11.874 | 0.000 | −0.051 | 0.000 | −2.306 | 0.011 | −1.046 | 0.148 | 1.255 | 0.105 |
ΔLn(REM) | −25.859 | 0.000 | 0.189 | 0.000 | −0.842 | 0.200 | −0.480 | 0.316 | 2.258 | 0.012 |
Source: Prepared by the author. Unit root tests performed with Stata version 15.
Cointegration Tests
After verifying that these series are integrated of order one [I(1)], it is determined whether a cointegration relationship exists in order to prevent the inconvenience of obtaining spurious results, which can occur when the number of time observations is greater than the number of individuals in a panel. The Pedroni cointegration test was applied to determine whether there is a long-run relationship between the variables contemplated in the panel data model and the following hypotheses were established:
H0: No cointegration
H1: All panels are cointegrated
Confidence level (α): 10%
Table 5 presents the results of the Pedroni cointegration test that analyzed the dependent variable with the independent variable and the proxy variables, showing that four of the seven statistics, at 10% significance, reject the null hypothesis of no cointegration, leaning towards the alternative hypothesis of cointegration. That is, the series are cointegrated when a linear combination of several series I(1) is stationary. It is concluded that the statistical evidence points to the existence of a cointegration relationship between the variables of the model (Equation 1). Therefore, the I(1) series are in long-run equilibrium: they move together, although a group of them may wander arbitrarily.
Table 5 Results of the Pedroni Cointegration Test - Fatal Workplace Accidents.
Statistic | p-value | |
---|---|---|
Between - Dimension (Autoregressive Parameter: Specific Panel) | ||
Modified Phillips-Perron t | 3.4353 | 0.0003 |
Phillips-Perron t | −1.4116 | 0.0790 |
Augmented Dickey-Fuller t | 0.4652 | 0.3209 |
Within - Dimension (Autoregressive parameter: All Panels) | ||
Modified variance ratio | −2.6595 | 0.0039 |
Modified Phillips-Perron t | 2.4258 | 0.0076 |
Phillips-Perron t | −1.2546 | 0.1048 |
Augmented Dickey-Fuller t | −0.0378 | 0.4849 |
Source: Prepared by the author. Cointegration test performed with Stata version 15.
Estimation of Long-Run Coefficients
The United Nations Economic Commission for Latin America and the Caribbean considers the OLS (Ordinary Least Squares) method to be the dominant estimation method because, under the assumptions of the Gauss-Markov theorem (the normality assumption is not necessary), it is the best unbiased linear estimator (Comisión Económica para América Latina y el Caribe, 2011). Under this approach and upon determining the existence of a long-run relationship between the variables (the series are cointegrated), the regression coefficients (elasticities) were estimated using the DOLS (Dynamic Ordinary Least Squares) consistent estimator, which produces asymptotically unbiased and normally distributed estimators.
Table 6 presents the results of the long-run coefficients of the model Equation 1 applying the DOLS estimator. It is observed that the coefficient along the economic growth variable, measured through the Gross Value Added, reflects the existence of the influence on fatal occupational accidents (direct relationship), but it is not statistically significant (p > 0.05). This represents that with a 1% increase in the Gross Value Added in the long run, there is a 4.34% increase in the number of fatal occupational accidents.
Table 6 Estimation of Long-Run Coefficients: Fatal Workplace Accidents and Economic Growth.
Variable | Coefficient | Standard Deviation | z | p > (z) |
---|---|---|---|---|
Ln(VAB) | 4.3415 | 3.1295 | 1.39 | 0.165 |
Ln(Tact) | −4.4008 | 6.8209 | −0.65 | 0.519 |
Ln(EMP) | −7.6844 | 2.9790 | −2.58 | 0.010 |
Ln(TRAB) | 3.1105 | 1.3706 | 2.27 | 0.023 |
Ln(REM) | 4.8575 | 2.7921 | 1.74 | 0.082 |
Source: Prepared by the author. DOLS estimation performed with Stata version 15.
DISCUSSION
The coefficient of the Gross Value Added of the panel data model (Equation 1) was positive and statistically non-significant. This means that there is a direct proportional relationship between economic growth and fatal occupational accidents, which is consistent with the studies by Gümüş and Gülsün (2020) and Ovejero et al. (2018). Similarly, procyclical behavior is observed, meaning that fatal occupational accidents increase during economic booms and decrease during recessions (Kim & Park, 2020). In contrast, in the Çolak and Palaz (2017) study, it is concluded that economic development and fatal occupational accidents have a negative relationship in the long run. Moreover, in the study of Mouza and Targoutzidis (2012), it was found that the increase of business cycle indicators results in a reduction of fatal occupational accidents.
Although the proxy variables are not the subject of this study, it should be noted that:
The coefficient of the Activity Rate was negative. Similarly, saving the differences in calculation between Activity Rate and Unemployment Rate, Łyszczarz and Nojszewska (2018) also identified a negative relationship between unemployment rate and occupational accidents. Thus, if unemployment rate increases occupational accidents decrease.
The coefficient of Monthly Average Number of Private Sector Companies turned out to be negative and statistically significant. This behavior is similar to the study by Łyszczarz and Nojszewska (2018) that included the number of Polish medium-sized enterprises as a control variable.
The coefficient of the Monthly Average Number of Private Sector Workers was positive and statistically significant. In the studies by Contreras et al. (2019), Ovejero et al. (2018) and Chang et al. (2018) this same behavior was identified, especially in new workers and temporary workers.
The coefficient of the Monthly Average Compensation of Private Sector Workers was positive and statistically significant. The same behavior was identified in the studies by Contreras et al. (2019) and Ovejero et al. (2018).
It is important to keep in mind that during periods of economic boom there is a scarce supply of skilled labor, so employers incorporate new and inexperienced workers. Also, due to growing demand, they increase labor intensity and make use of old and unsafe machinery (Kossoris, 1938). In this context, it is the responsibility of employers to manage their systems, processes and resources to address those internal factors under their control that also have an impact on the occurrence of occupational accidents:
Environmental and organizational factors: These include the design of the work, the work environment, the organization of work and the characteristics of the workplace; and
Personal factors: These include monthly income, age, gender, motivation and work experience.
Fatal occupational accidents are expected to decrease during economic recessions, but it is very common for workers who are hired during these periods to be trapped in low-quality jobs combined with hazardous tasks (Leombruni et al., 2019). Therefore, it is also up to the labor authority to monitor and ensure that employers provide safe and healthy working conditions.
CONCLUSIONS
This study explored the influence of economic growth on occupational accidents during the period 2011-2019 in Peru. The results indicate that there is a direct relationship between economic growth and fatal occupational accidents, but that it is not statistically significant. In other words, the economic boom represented by the increase in the value of all goods and services produced in the country would lead to an increase in fatal occupational accidents.
Considering that the relationship between economic growth and the occurrence of fatal occupational accidents has been established, the government should complement economic policy with strategies that contribute to creating greater awareness to strengthen employers’ duty of prevention and workers’ participation in occupational safety and health. Employers should incorporate occupational safety and health into their organizational management systems to provide safe and healthy working conditions.
Finally, further studies on occupational accidents in various economic sectors should be conducted to discover their sensitivity to long-term economic growth.
REFERENCIAS
Chang, D. S., Chen, Y., y Tsai, Y. C. (2018). How injury incidence is associated with business cycles? Empirical evidence from Taiwan. Safety Science, 110(Part A), 235-248. http://dx.doi.org/10.1016/j.ssci.2018.08.014 [ Links ]
Çolak, O., y Palaz, S. (2017). The Relationship between Economic Development and Fatal Occupational Accidents: Evidence from Turkey. Scientific Annals of Economics and Business, 64(1), 19-31. http://dx.doi.org/10.1515/saeb-2017-0002 [ Links ]
Comisión Económica para América Latina y el Caribe. (2011). Modelos de series de tiempo y decisiones de política pública. https://www.cepal.org/sites/default/files/courses/files/03._modelos_de_series_de_tiempo_y_desiciones_de_politica_publ.pdf [ Links ]
Contreras, S., Manzanedo, M. A., y Herrero, A. (2019). A Hybrid Neural System to Study the Interplay Between Economic Crisis and Workplace Accidents in Spain. Journal of Universal Computer Science, 25(6), 667-682. http://dx.doi.org/10.3217/jucs-025-06-0667 [ Links ]
Cossio Peralta, A. J. (2016). Hacia una aproximación al estudio del impacto económico de los accidentes de trabajo en el Perú durante los años 2011 a 2014: La prevención de los riesgos laborales y la productividad. Revista Derecho y Sociedad, (46), 401-413. [ Links ]
Enríquez Pérez, I., (2016). Las teorías del crecimiento económico: notas críticas para incursionar en un debate inconcluso. Revista Latinoamericana de Desarrollo Económico, (25), 73-125. https://doi.org/10.35319/lajed.20162564 [ Links ]
Gómez-Pineda, F. H. (2014). Reflexión Ética: La Relación Conflictiva Entre Salud y Trabajo. Revista Colombiana de Salud Ocupacional, 4(4), 5-11. https://doi.org/10.18041/2322-634X/rcso.4.2014.4959 [ Links ]
Gümüş, R., y Gülsün, Z. (2020). Occupational health and safety indicators of Turkey and their relationships with social and economic development factors between 1998 and 2014. International Journal Of Healthcare Management, 13(2), 1-12. http://dx.doi.org/10.1080/20479700.2017.1308669 [ Links ]
Instituto Nacional de Seguridad e Higiene en el Trabajo, (2014). Relación entre siniestralidad y crecimiento económico: sí, pero hay algo más…. https://www.insst.es/documents/94886/152662/Número%20133%20-%20Erga%40nline.pdf [ Links ]
Jiménez, F. (2011). Crecimiento Económico: Enfoques y Modelos. Lima, Perú: Pontificia Universidad Católica del Perú. [ Links ]
Kahraman, E., Akay, Ö., y Kılıç, A. (2019). Investigation into the relationship between fatal work accidents, national income, and employment rate in developed and developing countries. Journal of Occupational Health, 61(3), 213-218. http://dx.doi.org/10.1002/1348-9585.12021 [ Links ]
Kim, D. K., y Park, S., 2020. Business Cycle and Occupational Accidents in Korea. Safety and Health at Work, 11(3), 314-321. http://dx.doi.org/10.1016/j.shaw.2020.05.002 [ Links ]
Kossoris, M. D. (1938). Industrial injuries and the business cycle. Monthly Labor Review, 46(3), 579-594. [ Links ]
Leombruni, R., Razzolini, T., y Serti, F. (2019). Macroeconomic Conditions at Entry and Injury Risk in the Workplace. The Scandinavian Journal of Economics, 121(2), 783-807. http://dx.doi.org/10.1111/sjoe.12285 [ Links ]
Ley N.° 29783. (2011). Ley de Seguridad y Salud en el Trabajo. Diario Oficial El Peruano. Normas Legales: 448694-448706. [ Links ]
Loayza, N., y Soto, R. (Eds.) (2002). The Sources of Economic Growth: An Overview. En Economic Growth: Sources, Trends, and Cycles. Central Bank of Chile. [ Links ]
Loimer, H., y Guarnieri, M. (1996). Accidents and acts of God: a history of the terms. American Journal of Public Health, 86(1), 101-107. https://dx.doi.org/10.2105/ajph.86.1.101 [ Links ]
Łyszczarz, B., y Nojszewska, E. (2018). Economic Situation and Occupational Accidents in Poland: 2002-2014 Panel Data Regional Study. International Journal of Occupational Medicine and Environmental Health, 31(2), 151-164. http://dx.doi.org/10.13075/ijomeh.1896.01093 [ Links ]
Mejía, C. R., Cárdenas, M. M., y Gomero Cuadra, R. (2015). Notificación de accidentes y enfermedades laborales al Ministerio de Trabajo. Perú 2010-2014. Revista Peruana de Medicina Experimental y Salud, 32(3), 526-531. [ Links ]
Mouza, A. M., y Targoutzidis, A. (2012). The impact of the economic cycle on fatal injuries. The case of UK 1971-2007. Quality And Quantity, 46, 1917-1929. http://dx.doi.org/10.1007/s11135-012-9700-9 [ Links ]
Organización Internacional del Trabajo. (1998). Enciclopedia de Salud y Seguridad en el Trabajo. https://www.insst.es/documentacion/enciclopedia-oit [ Links ]
Ovejero, S., Del Campo, M., y Cosío, A. (2018). Crisis económicas y su influencia en la siniestralidad laboral. Dirección y Organización, (65), 5-19. http://dx.doi.org/10.37610/DYO.V0I65.525 [ Links ]
Pahuacho Maguiña, P. J. (2017). Costo de oportunidad de los accidentes de trabajo en base al análisis de indicadores de seguridad y salud ocupacional en el Perú. Análisis basado en los registros del Ministerio de Trabajo y Promoción del Empleo 2014-2016. (Tesis de maestría). Universidad de San Martín de Porres, Lima. https://hdl.handle.net/20.500.12727/3163 [ Links ]
Surry, J. (1971). Industrial Accident Research - A Human Engineering Appraisal. Toronto, Canadá: University of Toronto. [ Links ]
Teixidó, P., (2009). La prevención en época de crisis. https://amat.es/wp-content/uploads/2021/03/la-prevencion-en-epoca-de-crisis.pdf [ Links ]
Received: March 20, 2023; Accepted: June 22, 2023; pub: December 20, 2023