1. Introduction
The role of FDI inflows in the fight against poverty and income inequality has been noted in the development agendas of policymakers in several countries. But its influence on income inequality is still an emerging debated topic among economists. Obiero and Topuz (2022) highlight that public spending financed by government debt can cause income inequality. Meanwhile, progress in digital technology is an irreversible process in countries worldwide. Digitalization is considered one of the appropriate solutions to help developing economies catch up with developed economies in economic development. Starting from the related theories from Rostow (1960), Feenstra and Hanson (1997) and Helpman et al. (2004), a strand of literature has investigated the FDI - income inequality relationship, attempting to test the negative/positive impact of FDI on income inequality. However, no existing studies consider the contribution of digitalization to the FDI - income inequality relationship.
In the context of rising globalization, income inequality has become one of the severe problems in both developed and developing countries. Governments in advanced economies have many resources to tackle this problem. Meanwhile, governments in developing economies always try to improve the quality of life and eliminate poverty and income inequality to achieve the United Nations Millennium Development Goals. Developing economies lack the resources and appropriate solutions to reduce income inequality compared to advanced economies. Improving digital technology is one of the effective ways to help poor people access knowledge and improve skills to enhance income. Digital technology is also a development-oriented goal in developing economies for e-government. However, the development process of digital technology notes a digital divide in society in which the rich have better access to digital technology than the poor. The reason can stem from the cost and knowledge required to access digital technology facing low-income individuals. This problem seems more severe in developing economies because the poor in these economies have relatively low-income levels and spend most of their income on food and accommodation.
UNCTAD (2020) notes that developed economies attracted $800bn in 2019, a 5% increase from $761bn in 2018. Despite uncertain policies and weak macroeconomic performance, including tensions in trade and Brexit, growth still occurred. FDI dynamics in Europe, where inflows increased by 18% to $429bn, motivated the trend. Some European countries experienced strong fluctuations. For instance, Ireland attracted $78bn in 2019, up from -$28bn in 2018. By contrast, FDI inflows into several large economies decreased, with FDI inflows reducing by 50% in Germany but declining slightly in the United Kingdom and France. FDI flows remained in North America, with $297bn, and the United States remained the most prominent host of FDI inflows despite a slight decline. Notably, registered FDI flows in Australia declined due to a decrease in the value of cross-border M&As. Developing economies received $685bn, which declined marginally by 2%. FDI inflows into these economies have been relatively steady since 2010, oscillating around the narrow range of an average of $674bn. FDI flows to developing Asian countries decreased by 5% to $474bn in 2019 (UNCTAD (2020) ). Despite the decline, it was still the most significant host region of FDI inflows, receiving around 30% of global FDI flows. The 34% decrease in Hong Kong triggered the decline. Singapore, India, China, Indonesia and Hong Kong were the five largest hosts of FDI flows. The statistical analysis reported that China remained the second-largest host of FDI flows after the United States. Due to increased demand for commodities and moderate economic growth, FDI inflows into Africa in 2019 reduced by 10% to $45bn. FDI inflows into some commodity-exporting countries (Nigeria, Sudan, etc.) or countries with relatively more diversified FDI inflows (Morocco, South Africa, Ethiopia, etc.) declined. Exceptionally, FDI flows to Egypt, Africa's largest host of FDI inflows, increased by 1% to $9bn. Countries in Latin America and the Caribbean attracted $164bn of FDI flows, which increased by 10%. FDI flows in commodities, utilities and services to Peru, Brazil, Chile and Colombia rose.
Similarly, UNCTAD (2021) reports that the development of global digital technology could be more balanced. These days, the world is characterized by a clear distance between hyper-digitalized and under-connected economies. Compared with four out of five in developed economies, only one in five people uses the internet in the least developed economies. It mirrors just one aspect of the digital divide. The divide is significant in some sectors, such as frontier technologies and digital data. For instance, Latin America and Africa account for less than 5% of the world's colocation data centres. Geographically, UNCTAD (2021) says the digital economy does not reflect a traditional North-South divide. It is currently being led by one developing and one developed economy: China and the United States. More than 75% of the world market for public cloud computing, 50% of global expenditure on the Internet of things, and 75% of all patents connected to blockchain technologies belong to these two countries. Notably, they capture 90% of the market capitalization value of the world's 70 largest digital platforms, while Africa and Latin America's share is 1% and Europe's is 4%. Thus, in progress in digital technology, the rest of the world (especially Latin America and Africa) is left behind by China and the United States.
Meanwhile, Alvaredo et al. (2018) report that income inequality is lowest in Europe and highest in the Middle East. The share of total national income in 2016 captured by the top 10% earners was 37% in Europe, 41% in China, 46% in Russia, 47% in Canada-US and 55% in sub-Saharan Africa, Brazil and India. The Middle East is the world's most unequal region, in which the top 10% captures 61% of national income. Notably, only 3 of the 32 states in Mexico generated and distributed income and wealth efficiently (Ayvar-Campos et al., 2020). Global income inequality has risen sharply since 1980. High economic growth in Asia (especially in China and India) has led to income growth in the bottom 50% of individuals. Unfortunately, the world's top 1% of wealthiest individuals have captured twice as much growth as the global bottom 50% since 1980 due to increasing income inequality within countries. However, the increase in global income inequality has not been stable. The income share of the global top 1% declined slightly to 20% from 16% in 1980 after reaching 22% in 2000. The global bottom 50% income has fluctuated around 8% since 1980. According to Alvaredo et al. (2018, the different levels of income inequality across countries, even across countries with the same development levels, show the significant role of regulations and policies (institutional quality) in solving income inequality. Income inequality has increased in China, India, North America and Russia since 1980, while it has been moderately in Europe. It has remained relatively steady in Brazil, sub-Saharan Africa and the Middle East. The statistical data across countries since 1980 show that political and institutional contexts have affected income inequality dynamics. The positive trends in income inequality in China, Russia and India reflect various policies pursued over the past decades in these countries. However, the difference in income inequality between the United States and Western Europe has been maintained highly since 1980.
Concerning the academic aspect, notably, the literature review in Section 3 highlights three elements that make this paper different from related studies. First, it introduces digitalization into the FDI - income inequality relationship. Second, it provides empirical evidence to show the different roles of digitalization in the FDI - income inequality relationship between developed and developing countries. Third, it applies the system general method of moments (GMM) estimators (S-GMM).
In short, stemming from the fact that narrowing income inequality and attracting more FDI inflows play a crucial role in the development agenda of both developed and developing countries, and digitalization differently contributes to the FDI - income inequality relationship between them. The study examines the effects of FDI, digitalization, their interaction on income inequality for 30 developed and 35 developing countries from 2002 to 2019 using S-GMM.
We note that Salcedo (2021a, b) mention how to structure a paper to attract more readers. Notably, he systematically reviewed the previous ten years of JEFAS (Journal of Economics, Finance and Administrative Science) publications as a roadmap to make a better structure for a paper. Following Salcedo (2021a, b), we present it in the following way. Section 1 is the Introduction. Section 2 describes the theoretical framework and looks at the literature review. The methodology in Section 3 emphasizes the characteristics and appropriateness of S-GMM. Section 4 notes the results, while Section 5 is a discussion. The final section in Section 6 concludes and suggests some important policy implications.
2. Literature review
2.1 Theoretical framework
Given the topic, some theories show the effect of FDI inflows on income inequality. First, according to modernization theory, Rostow (1960) argues that a host country's different stages of development processes will have distinct effects on income inequality. This theory suggests that FDI inflows will initially enhance income inequality at the early stages of development processes, but this impact will decrease once an optimal development stage is achieved. Secondly, the North-South FDI model by Feenstra and Hanson (1997) argues that FDI inflows can lead to higher income inequality in developing economies due to driven comparative advantage. Specifically, when FDI inflows from developed economies in the North are skill-biased, the unskilled-skilled income gap of host developing economies in the South would rise. Thirdly, the heterogeneous enterprises model by Helpman et al. (2004) notes that FDI inflows would benefit the relatively abundant factor of production. In developing countries, FDI inflows can increase the income levels of the relatively plentiful factors (less skilled labour), which reduces income inequality.
Progress in digital technology can affect both FDI inflows and income inequality in the context of increasing globalization and digitalization. According to Qureshi (2020), income inequality across countries has increased since digital technology reshaped goods, businesses and work markets. As a result, wealth and income inequalities have risen between workers and enterprises. Notably, improvement in digital technology is a crucial factor in attracting FDI investors to look for skilled labour with cheap salaries in host countries. Therefore, digitalization can attract more FDI inflows, leading to changes in income inequality in host countries. This implies that the interaction term between digitalization and FDI can significantly affect income inequality. Furthermore, the difference in development level and governance between developed and developing countries can lead to different roles of digitalization in the FDI-income inequality relationship between them.
2.2 The effect of FDI on income inequality
Huang et al. (2020) surveyed 543 primary studies and noted that 222 studies indicate a positive effect of FDI on income inequality, while the remaining 321 report a negative or insignificant impact.
Regarding the adverse effect, most studies recommend that governments should attract more FDI inflows to eradicate hunger and reduce poverty. For example, Herzer and Nunnenkamp (2013) show that FDI inflow reduces income inequality in 8 European countries from 1980 through 2000 using the two-step GMM estimator and panel cointegration techniques. Recently, Matallah (2019) indicated that FDI inflow narrowed income inequality in six South Asian countries between 1996 and 2012 via estimators of pooled OLS (Ordinary Least Squares), fixed-effects model (FEM), random-effects model (REM) and the one-step difference GMM. Teixeira and Loureiro (2019) support the negative effect of FDI inflow on income inequality in Portugal by using the vector error-correction models (VECM) for time series data from 1973 to 2016.
Regarding the positive effect, most of the investigations use panel data regressions. Pan-Long (1995) employed the pooled OLS estimator for 53 countries between 1968 and 1981. Meanwhile, Basu and Guariglia (2007) used FEM, REM and one-step system and difference GMM estimators for a selection of 119 developing countries from 1970 to 1999. Similarly, Figini and Gorg (2011) used the estimators of FEM, pooled OLS, and one-step system GMM for a group of 103 developing and developed countries between 1980 and 2002. Also, both Chintrakarn et al. (2012) and Herzer et al. (2014) applied panel cointegration techniques for 48 US States from 1977 to 2001 and for a group of Latin American countries over the period 1980-2011. Recently, Alili and Adnett (2018) applied estimators of REM and FEM for a sample of 19 transition countries from 1993 to 2008, while Khan and Nawaz (2019) used the one-step system GMM estimator for a sample of 12 countries of the Commonwealth of the Independent States between 1990 and 2016. These studies suggest that governments should re-design regulations and policies to limit the adverse impacts of FDI inflows on income inequality.
Notably, Cho and Ramírez (2016) and Kaulihowa and Adjasi (2018) report the non-linear effect of FDI inflow on income inequality. Cho and Ramírez (2016 use the group-mean fully modified ordinary least squares (FMOLS) for a sample of 7 selected Southeast Asian countries from 1990 to 2013, while Kaulihowa and Adjasi (2018 employ the panel mean group (PMG) and MG (Mean Group) estimators for a group of 16 African countries from 1980 to 2013. Cho and Ramírez (2016 indicate that income inequality starts reducing after the ratio of FDI/GDP (Gross Domestic Product) reaches 5.6%. Meanwhile, Wu and Hsu (2012) and Lin et al. (2013) state that the FDI - income inequality relationship depends on absorptive capacity/human capital. Wu and Hsu (2012 use the OLS estimator and the endogenous threshold regression model for a group of 54 countries (33 developing countries and 21 developed countries) from 1980 to 2005, while Lin et al. (2013) use the instrumental variable (IV) threshold regressions approach for a sample of 73 countries between 1960 and 2005.
Unlike the above studies, Reuveny and Li (2003) and Ángeles-Castro (2011) introduce governance into the empirical equations. Through the pooled OLS estimator for a group of 69 countries over the period 1960-1996, Reuveny and Li (2003 note that FDI widens income inequality while democracy narrows it. Ángeles-Castro (2011 finds that FDI enhances income inequality in countries with low governance (significantly) and countries with high governance (insignificantly) using FEM, REM and one-step system GMM estimator for a panel data of 93 countries over the period 1980-1998.
2.3 The effect of digitalization on income inequality
Qureshi (2020) argues that income inequality within economies has risen as progress in digital technology has reshaped markets of goods, business and work. As a result, wealth and income inequalities have enhanced not only among workers also among firms. Similarly, Zilian and Zilian (2020) find socio-economic digital inequality in Austria using survey data (PIAAC (Programme for the International Assessment of Adult Competencies)) from 2011 to 2012.
There are few studies on the relationship between digitalization and income inequality. However, some studies report that progress in digital technology decreases income inequality (Richmond and Triplett, 2018; Canh et al., 2020), while some note that digitalization increases it (Mönnig et al., 2019; Law et al., 2020; Mohd Daud et al., 2020).
Regarding the negative impact of digitalization on income inequality, Richmond and Triplett (2018) use the fixed effects estimator for 109 countries during 2001-2014. They find that the effect of digitalization on income inequality depends on the specific type of digital technology and the measure of income inequality. Similarly, Canh et al. (2020) apply the two-step system GMM estimator for 87 countries from 2002 to 2014. They note that progress in digitalization and communication is an appropriate way to decrease income inequality in the short and long run. Therefore, Internet and mobile use should be encouraged as a part of an active economic policy to reduce income inequality.
Regarding the positive impact of digitalization on income inequality, Mönnig et al. (2019) use the analytical approach to examine the effect of digital technology on wage inequality. They conclude that digitalization enhances income inequality. Meanwhile, Law et al. (2020) use the PMG estimator for 23 developed countries from 1990 to 2015, while Mohd Daud et al. (2020) apply the one-step system GMM estimator for 54 countries over the period 2010-2015. Both studies provide empirical evidence to confirm that digital technology widens income inequality. Hoi and Tran (2020) find that commercial credit widens income inequality, but policy credit narrows it in Vietnam between 2002 and 2016.
From the literature perspective, we suggest three hypotheses as follows:
H1. FDI has a positive effect on income inequality.
H2. Digitalization has a negative effect on income inequality.
H3. The interaction term between FDI and digitalization has a negative effect on income inequality.
3. Method
3.1 Methodology
Following Teixeira and Loureiro (2019), Herzer and Nunnenkamp (2013), and Figini and Gorg (2011), the empirical models are presented as follows:
where subscript i and t are the country and time index, respectively. GINit is the GINI index - a proxy for income inequality. Its value ranges from 0 to 1, where 0 reports complete income equality (all individuals have equal incomes) and 1 shows the highest level of income inequality; GINit-1 is the initial level of income inequality, FDIit is the net FDI inflows, DIGit is individuals using the internet/fixed broadband subscriptions - a proxy for digitalization, (FDI X DIG) it is the interaction between FDI and digitalization, and GOVit is governance (voice and accountability, control of corruption, political stability and absence of violence, government effectiveness, the rule of law and regulatory quality). FDI inflows can have certain impacts on income inequality in recipient countries. As arguments suggested in Sub-section 3.1, digitalization can reduce income inequality. Digital progress in host countries attracts more FDI inflows,which can affect income inequality. Therefore, the paper introduces the interaction term between digitalization and FDI (FDI XDIG) it in the empirical models. Zit contains economic growth and education; μi is an unobserved time-invariant, country-specific effect and ζit is an observation-specific error term; γ0, γ1, γ2, γ3, γ4, γ5 and γ0 are estimated coefficients.
There are four severe problems of econometrics from regressing Equation (1). Firstly, digitalization, education and economic growth may be endogenous. They may correlate with the term μi, leading to the endogenous phenomenon. Secondly, some country-specific and unobserved time-invariant characteristics like anthropology and geography may correlate with regressors. They exist in the term μi. Thirdly, the presence of GINit-1in the empirical equations may lead to a high autocorrelation. Fourthly, the panel dataset has a relatively large unit of countries (N = 35) and a relatively short observation length (T = 19). They can make the OLS regression inconsistent and biased. FEM and REM cannot tackle autocorrelation and endogenous phenomena. At the same time, the IV - two-stage least square (2SLS) estimator requires some appropriate IVs that are out of regressors in the model. Therefore, following Judson and Owen (1999), the paper uses the two-step and one-step S-GMM (1S-GMM and 2S-GMM) for estimation and robustness checks.
Holtz-Eakin et al. (1988) are the first to propose the GMM Arellano and Bond (1991). There are two kinds of GMM estimators: the difference and the system. The past values of persistent variables provide little information about their future changes, making their lags weak instruments in the difference GMM estimator. Therefore, S-GMM is better than the difference GMM estimator (Arellano and Bover, 1995).
For estimation, 2S-GMM is more asymptotically efficient than 1S-GMM. However, employing 2S-GMM in small research samples like our sample has a problem (Roodman, 2009). It is the proliferation of instruments that quadratically rises as the time dimension increases, which causes the number of IVs to be very large relative to the number of panel units. The solution is to apply the rule of thumb to keep them less than or equal to the number of panel units (Roodman, 2009). The study uses the Arellano-Bond, Sargan and Hansen statistics to test the validity of instruments in 2S-GMM. The Arellano-Bond test AR(2) searches the autocorrelation of errors in the first difference, while the Sargan and Hansen tests detect endogenous phenomena.
3.2 Research data
The variables are the GINI index, net FDI inflows, individuals using the Internet, governance indicators, GDP per capita and school enrolment. The paper extracts them from the World Bank world development indicators (WDI) and worldwide governance indicators (WGI) databases. Due to unavailable data on the GINI index, the research sample contains 30 developed countries (1) and 35 developing countries (2) from 2002 to 2019.
Table 1 presents the definition and descriptive statistics of the data. The results in Tables 2 and Tables 3 note that governance in the sample of developing economies is relatively low compared to that in the sample of developed economies, suggesting that most developing countries have poor governance and developed countries have good one. It is consistent with the approach of Li and Filer (2007) that most of the developed countries are those with rule-based governance (good governance) and most developing countries are those with relation-based governance (poor governance).
4. Results
4.1 2S-GMM estimates
The study reports the estimates in Tables 4 and Table 5 (developed countries) and in Tables 6 and Table 7 (developing countries). Tables 4 and 6 use individuals using the Internet, while Tables 5 and 7 employ fixed broadband subscriptions as a proxy for digitalization. We detect that digitalization is endogenous in all estimation procedures. Therefore, we use digitalization as instrumented in the GMM style and the remaining variables as instruments in the IV style.
Table 4 FDI, digitalization and income inequality (30 developed countries)
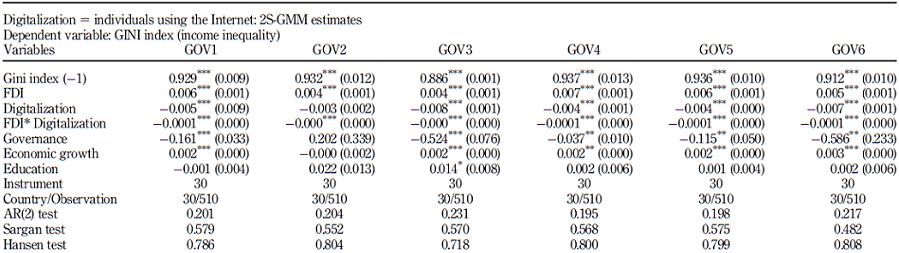
Source(s): Own elaboration. Note(s): ***, **, *report significance level at 1%, 5%, and 10%
Table 5 FDI, digitalization and income inequality (30 developed countries)
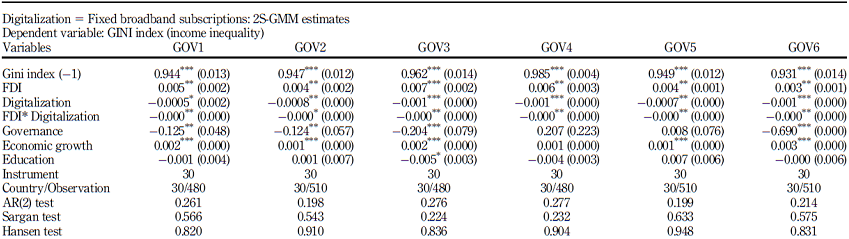
Source(s): Own elaboration. Note(s): ***, **, *report significance level at 1%, 5%, and 10%
Table 6 FDI, digitalization and income inequality (35 developing countries)
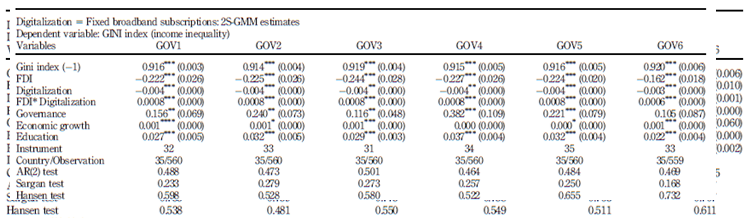
Source(s): Own elaboration. Note(s): ***, **, *report significance level at 1%, 5%, and 10%
Table 7 FDI, digitalization and income inequality (35 developing countries)
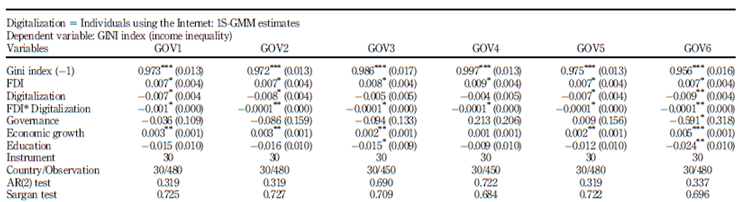
Source(s): Own elaboration. Note(s): ***, **, *report significance level at 1%, 5%, and 10%
The results across all models show that FDI widens income inequality in developed countries (validating the H1 hypothesis) but narrows it in developing countries (rejecting the H1 hypothesis). Meanwhile, digitalization reduces income inequality in both groups (supporting the H2 hypothesis). However, their interaction narrows income inequality in developed countries (validating the H3 hypothesis) but increases it in developing countries (rejecting the H3 hypothesis). These results are highly consistent for all governance indicators. In addition, economic growth enhances income inequality in both groups. Notably, governance reduces income inequality in developed countries but boosts it in developing countries.
4.2 Robustness check
To check the robustness of 2S-GMM estimates, we re-estimate Equation (1) using 1S-GMM. The paper shows the corresponding results across all models in Tables 8, Table 9, Table 10 and Table 11. In line with 2S-GMM estimates, 1S-GMM indicates that (1) FDI widens income inequality in developed countries but narrows it in developing countries; (2) Digitalization reduces income inequality in both groups; (3) interaction narrows income inequality in developed countries but increases it in developing countries.
Table 8 FDI, digitalization and income inequality (30 developed countries)
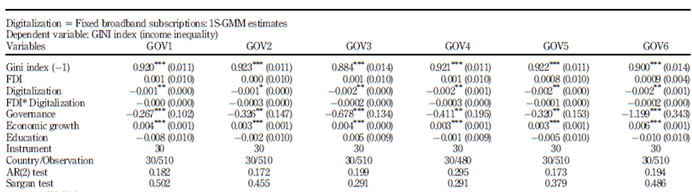
Source(s): Own elaboration. Note(s): ***, **, *report significance level at 1%, 5%, and 10%
Table 9 FDI, digitalization and income inequality (30 developed countries)
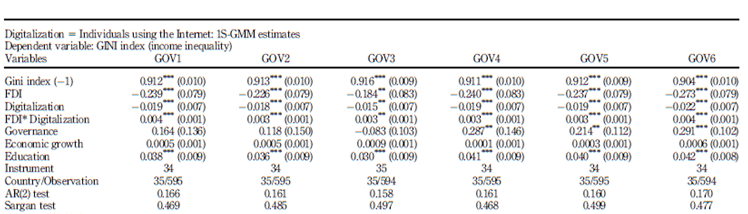
Source(s): Own elaboration. Note(s): ***, **, *report significance level at 1%, 5%, and 10%
Table 10 FDI, digitalization and income inequality (35 developing countries)
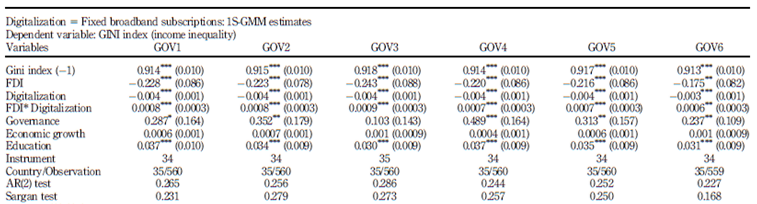
Source(s): Own elaboration. Note(s): ***, **, *report significance level at 1%, 5%, and 10%
5. Discussion
5.1 Theoretical implications
The opposite effects of FDI on income inequality between developed and developing countries seem counterintuitive. We suggest some arguments to explain as follows. First, institutional quality (policies and regulations) positively impacts attracting FDI inflows (Mahmood et al., 2019). Most developing economies have large populations with unskilled and low education, and unemployment falls mainly among unskilled and low-income workers. Policies and regulations related to FDI inflows mainly focus on employing most unskilled and low-income workers, so the low-quality FDI inflows (FDI with outdated technology and low management level) into host countries will enhance income for these low-income workers. These FDI inflows will reduce the income gap between the rich and the poor, thus narrowing income inequality. Developed economies have a small population with a high rate of skilled and educated workers. The policies and regulations related to FDI inflows are implemented strictly to attract more high-quality FDI inflows (FDI with advanced technology and high management level). Therefore, the high-quality FDI inflows into host countries mainly focus on highly educated and skilled high-income workers. These FDI inflows increase the income gap between the rich and the poor, which thus widening income inequality. Likewise, some previous studies, like Richmond and Triplett (2018) and Canh et al. (2020), show the negative impact of digitalization on income inequality. Progress in digital technology is an appropriate opportunity to help the poor enhance their knowledge and skills, increasing their ability to find high-paying jobs and decreasing the income gap between the rich and the poor, thereby narrowing income inequality. However, the different effects of interaction on income inequality between developed and developing countries may stem from progress in digital technology. Progress in digital technology will lead to several high standards for attracting FDI inflows. Regulations and policies to attract FDI inflows in developed and developing countries have become more rigorous to eliminate the adverse effects of FDI inflows on economic development process such as environmental pollution, outdated technology, etc., which encourages FDI inflows with modern technology. FDI inflows into these groups of countries will decrease in the context of increasing digitalization. Thus, the decline in FDI due to progress in digital technology in developed countries will narrow income inequality. By contrast, the decrease in FDI due to progress in digital technology in developing countries will widen income inequality.
In developed countries with a high level of economic development, governments implement regulations and policies (governance) to reduce the income gap between the rich and the poor, thus narrowing income inequality. It is consistent with the findings in Matallah (2019). In contrast, governments in developing countries formulate and implement regulations and policies (governance) to promote economic growth. As a result, economic growth outcomes benefit the rich, increasing the income gap between the rich and the poor, thus widening income inequality. Canh et al. (2020) provide this empirical finding.
Contrary to Obiero and Topuz (2022), this paper notes that economic growth enhances income inequality. The process of economic development in both developed and developing countries may only benefit a part of the rich, who have many resources to receive economic growth outcomes. The Kuznets curve by Kuznets (1955) hypothesizes that industrializing countries experience a rise and subsequent decline in income inequality. The paper notes this finding in Wu and Hsu (2012), Herzer and Nunnenkamp (2013), Kaulihowa and Adjasi (2018), and Teixeira and Loureiro (2019). This finding shows a challenge for governments in countries to allocate economic development and growth outcomes to all people across countries.
Notably, education in developed countries increases income inequality. In developed countries, education is generally universal, meaning that basic education provided by the state is free and students attend public schools without charges. However, wealthy families send their children to paid private schools with better and superior quality of education. As a result, students from wealthy families learn better knowledge and skills and thus find higher-paying jobs than those from average families. Therefore, education in developed countries widens income inequality. We note this finding in Figini and Gorg (2011), Herzer et al. (2014) and Kaulihowa and Adjasi (2018). Furthermore, we emphasize that increasing government spending on education and healthcare financed by debt can widen income inequality (Obiero and Topuz, 2022). In particular, Ayvar-Campos et al. (2020) discovered that only three of the thirty-two observed units generate and distribute income efficiently.
5.2 Policy implications
Unlike the arguments in theoretical models by Rostow (1960), Feenstra and Hanson (1997) and Helpman et al. (2004), this paper discovers that at early stages of development (developing countries), FDI inflows narrow income inequality and higher stages of development (developed countries) FDI inflows widen income inequality. Furthermore, digitalization and the interaction term between FDI and digitalization significantly affect income inequality. These findings are this paper's new contribution to the literature. Notably, they provide some crucial implications in designing, formulating and implementing policies and regulations relating to digital technology. Digitalisation is an irreversible process in countries worldwide. Progress in digital technology affects not only FDI inflows but also income inequality. Severe income inequality can result in social instability. Governments in countries should adjust the strategies and solutions of digital technology development to ensure attracting more FDI inflows and reduce income inequality. They should mitigate the adverse effects of digitalization by appropriate regulations and policies to narrow society's digital divide and income inequality.
5.3 Limitations and future research agenda
Future research should focus on the role of digitalization in the FDI - income inequality relationship by sector/industry between groups of countries. Besides, some econometric approaches like the panel quantile regression and the PMG estimator can be a suggestion for future research.
6. Conclusion
FDI is a crucial investment capital for economic development and growth in developed and developing countries. Meanwhile, progress in digital technology is an irreversible global phenomenon and a significant factor attracting more FDI inflows. Therefore, the study applies 1S-GMM and 2S-GMM to examine the effects of FDI, digitalization and their interaction on income inequality for 30 developed and 35 developing countries from 2002 to 2019. It uses Internet users and fixed broadband subscriptions as a proxy for digitalization. The results indicate that (1) FDI increases income inequality in developed countries (supporting the H1 hypothesis) but decreases it in developing countries (rejecting the H1 hypothesis), (2) Digitalization reduces income inequality in both groups (validating the H2 hypothesis), (3) interaction term narrows income inequality in developed countries (supporting the H3 hypothesis) but widens it in developing countries (rejecting the H3 hypothesis). Furthermore, these countries' governance, economic growth and education are determinants of income inequality.
Notes
1. Austria, Belgium, Canada, Cyrus, Czech Republic, Denmark, Estonia, Finland, France, Germany, Greece, Iceland, Ireland, Israel, Italy, Korea Republic, Latvia, Lithuania, Luxembourg, Malta, Netherlands, Norway, Portugal, Slovak Republic, Slovenia, Spain, Sweden, Switzerland, United Kingdom, United States.
2. Argentina, Armenia, Belarus, Bolivia, Brazil, Bulgaria, Chile, China, Colombia, Costa Rica, Croatia, Dominican Republic, Ecuador, El Salvador, Georgia, Honduras, Hungary, Indonesia, Kazakhstan, Kyrgyz Republic, Malaysia, Mexico, Moldova, Pakistan, Panama, Paraguay, Peru, Poland, Romania, Russian Federation, Thailand, Turkey, Ukraine, Vietnam, and West Bank and Gaza.