1. Introduction
COVID-19 pandemic is increasingly metamorphosing into a threat to economic activities in rural areas (FAO, 2020b). Arable crops production activities in Nigeria and other sub-Saharan Africa countries are among the economic activities becoming threatened (FAO, 2020a). The spread of the virus is escalating in this era when arable crop outputs have become stagnant or are progressively declining in some West African countries (FAO, 2020b). Hence, it is glaring that as government strive to promote adoption of innovations and rejuvenate the performance of agricultural sector, in order to satisfy the high and rising need for food, continuous and uninterrupted crop production activities become crucial in achieving this objective (Lybbert & Sumner, 2010). During the lock-down period prompted by COVID-19 pandemic, movements of people were restricted, even in the rural areas of Delta State apart from livestock farmers. However, upon the relaxation of the lock-down and the public awareness campaign by various communication media, people decided to migrate from large rural settlements to remote rural settlements for may be, fear of getting infected by the virus (Osahon, 2020). Coupled with higher rural population in large rural settlements and curfew imposed, many rural households embarked on migration to smaller rural settlements where population of people is lower and governmental control is absent, as observed. This COVID-19 crisis started during the cropping season making most farming households to abandon their existing farms and the ones in the process of cultivation (Akpata, personal communication, May 19, 2020), hence raising concerns over the likelihood of food insecurity and incidence of high level of poverty in the source communities of the migrants. In addition, the pandemic has the potential of adversely affecting the purchasing power of the people (non - farming households and farming households).
Literature on COVID - 19 in sub-Saharan Africa is scarce as many scholars have not carried out studies on it, especially the social and economic effects or impacts of the disease. However, many people have linked rural-rural migration to various reasons. Some of these reasons include need for security in whatever form, natural disasters, conflicts and need for fertile land (Ofuoku et al., 2019); opportunities to productive resources and opportunity to agricultural extension services among others (Adatom & May, 2004; Ofuoku, 2019). The COVID -19 can be regarded as one of the security concerns as human lives are affected. Africans are very emotional about their lives and place inestimable value on human life, which represents an important factor in their reactions towards the COVID-19 outbreak.
However, across - community migrations may involve tribes’ ad-mixture in the host communities. This is important because each tribe has its specific values and in ternal land arrangements. Conflicting values and tradi tional land arrangements have the capability of inhibiting the economic activities of migrants in their host commu nities (Mwesigye & Matsumot, 2013).
Despite the increasing incidents of the disease and its indisputable effect on agricultural activities and output, empirical studies on the consequences COVID-19 pandemic are scanty. For example, the nexus between rural - rural migration and COVID-19 pandemic has not been empirically examined in the presence of on-going migration incidents. This study was thus articulated to fill this gap in existing literature on COVID-19 pandemics.
COVID-19, a disease that is currently threatening and dis turbing humanity. The world history of past pandemics that were experienced globally indicates that quarantine and panic impact tremendously on human livelihood and economic activities (Bermejo, 2004; Hanashima & Tomobe, 2012); including agricultural activities. The presence of in fectious disease outbreak prompts increased hunger and malnutrition (SAR et al., 2010; Burgui, 2020). The situation becomes worse with the spread of the disease, causing serious and stringent movement restrictions, which gives rise to farm labor shortages for farm operations and dif ficulties for transportation of harvested products to markets by farmers (Siche, 2020). Only livestock farmers were excused during the period to attend to their livestock. This has caused a new wave or rural - rural migration to com munities where restrictions are very minimal. Agriculture forms one of the most important human development sectors and there is a nexus between it and food security (Abdelhedi & Zouari, 2020; Kogo et al., 2021; Lopez-Ridaura et al., 2019). Thus, the objective of this study is to examine the impact of COVID-19 induced rural - rural mi gration and agricultural productivity and how the associ ations are affected by to COVID-19 disease related events.
The results of this study are expected to be useful guides to policy makers for future disaster or pandemic occur rences. This is more so when such results to decisions on lockdown and other forms of restrictions. It will as well be a reference point for decisions to create a situation where the effects of lockdowns will be made in a balanced way that will lead to a compromise between lockdown and agricultural production activities.
2. Materials and methods
2.1. Data collection
Data were collected from household survey and community survey in Delta State, Nigeria. From the three agricultural zones in the state, two local governments that are predominantly rural were selected, to have 6 local government areas. From each selected local government areas, 2 large rural and 2 remote rural communities were selected resulting to selection of 12 remote rural and large rural communities (6 remote and 6 large communities). With the help of contact farmers, the list of farmers in each community was accessed. Systematic sampling method was used to select the farmers used for the study. This resulted to selection of 517 farmers.
Data were collected from the farmers with the use of structured interview schedule (questionnaire administered by face-to-face interview) and the contact farmers who were mostly opinion leaders in their respective communities helped to administer the interview schedule. Information pieces on demographic characteristics of the respondents were obtained by the first item on the interview schedule. The rate of migration was accessed through information from the primary data collected. The information on the characteristics of plots, parcel and household were obtained from the respondents. The data on agricultural productivity were obtained from community survey which was conducted by Delta State Agricultural and Rural Development Authority (DARDA).
2.2. Estimation Strategy
To assess the influence of communities’ factors on migration, linear probability modeling framework was adopted. Denote population in sending communities by P, households by i and remote rural community by j. Let Mpij be a dummy variable which is equal to 1 if migration occurs from sending community p during the last farming season by household i in rural settlement j. It can be hypothesized that living in the sending community with high population density, and governmental control of movement, having the knowledge of high soil quality and land availability in host community are related with a higher probability, of exhibiting willingness to migrate, while other factors are held constant. The formal estimation of linear probability equation of the form is as follows:
Where Rj is a dummy that assumes the value of 1 if the community is classified as receiving and zero if it is sending, Un means a dummy which assumes the value of 1 if movement is controlled and zero if otherwise in a community, L is land availability which stands for land size. Xij stands for a vector of household qualities, which include age of household head, sex, years of schooling, household size, and household assets (in Nigeria naira). Zj stands for a vector of other community controls - whether road to the community is tarred, all-season dirt, or season dirt road. These variables are capturing the impact of accessibility or remoteness of village on migration. Immi gration into a village increases along with accessibility. With respect to road quality, tarred road is of better qual ity than all - season dirt road, and season dirt road and it is followed in this order by all-season dirt road and season dirt road, Ɛpij stands for the characteristics error term.
To test for the effect of migration on crop yield, a household fixed effects regression was used including two farming seasons (the last farming season of 2019 and the first farming season of 2020) semi-panel set of data. Taking Ypis as the yield harvested from plot P, owned by household i in season s, the following regression was ran:
Where Mpis is a binary variable which is = 1 if migrated and 0 if otherwise. In a distinct specification, migration is broken down into permanent and temporary, hence Mpis in this context, is a vector of permanent and temporary migration types. Plot qualities Zpis is inclusive of distance to the farm plot in minutes, whether plot is leased or purchased. Xi is a vector of household controls which include farm size (ha), sex of household head, age and number of years of schooling, household assets. Vis is capturing household and season fixed effects. Then μpis is the error term which may assume heteroskedastic form and correlated in a household and season. This was adjusted for by utilizing robust standard errors and covariance matrices that gives room for “clustering” of error terms at village, year and season classification according to Wooldridge (2010).
3. Results and discussion
3.1 Descriptive statistics
Table 1 shows that in receiving villages, many people of the present generation and some people of the second generation of household were immigrants. In the sending rural settlements, few of the people represent generation and fewer of them represent second generation of households that immigrated. Receiving settlements were highly heterogeneous in nature as a result of the many immigrants from diverse ethnic groups as shown in the number of ethnic groups (6 ethnic groups), while sending communities had 3 ethnic groups. There were more persons per hectare in sending communities, than in the receiving communities. In Table 2 the fraction of households that form the largest ethnic group was used as one more assessment of the level of heterogeneity. It shows that in receiving communities, many of the households were of the largest ethnic group compared to the higher fraction in the sending communities. This is indicative of the fact that sending communities were densely populated than receiving communities because of the remote location of receiving communities. This prompted the migrations from the larger rural communities (sending communities) to the remote (receiving) communities.
3.2. Characteristics of plot, parcel and households
Table 3 indicates that households in sending communities had smaller farmland (2.5 ha) than the households in receiving communities (2.95 ha) on the average. There is an indication that fragmentation of farm land is more in sending communities (Thompson index* of 0.56) in comparison with Thompson index of 0.38 in receiving communities. A similar trend was observed by Mwesigye & Matsamoto (2013) in their study in Uganda. The mean distance to farm in communities was about 22 minutes higher than about 19 minutes observed in receiving communities. This is indicative of scarcity of land in sending communities that makes farmers to carry out their farming activities in faraway places from their homes where they were able to procure land.
Table 3 Characteristics of Plot, parcel and households
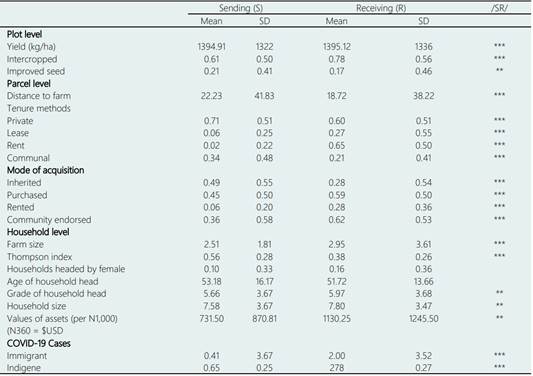
Computation from DARDA 2020 data and information from respondents.
*** Significant at 1%; ** Significant at 5% from 0 - 1. It is 0 if not fragmented.
Thompson index * is a calibration of the level of fragmentation of land which ranges from 0 to 1. It takes 0 if a household has one parcel and 1 if has infinite farm plots.
In sending communities, farmland was mainly acquired by purchase, rent or community endorsement. Indicating the scarcity of land in sending communities. While in receiving communities, farm plots were mainly acquired through community endorsement, rent, lease and private ownership (purchase or inheritance). This indicates that most plots of land belonged to the receiving communities, from which private owners purchased and others either leased on long-term or rented (short term). In sending communities, private customary arrangements for acquisition of land were prevalent than in the receiving communities, since 71% and 60% of the plots were held through private customary tenure-ship in sending communities and receiving community’s Communal ownership was predominant as the communities endorsed land for free in the first instance and as from the consecutive years they lease or rent, depending on the choice of the tenants, often determined by their financial standing. With respect to mode of acquisition, most of the plots of farmland were inherited in sending communities, while they were purchased in receiving communities. This shows that most people living in receiving communities were immigrants who purchased land from the communities and the indigenes of such communities. In sending communities, fewer plots were rented and more in receiving communities. It was observed that due to scarcity of land in sending communities, renting was phasing out and people took advantage of this to migrate to where they can access land for farming, this was further enhanced by the COVID-19 pandemic.
There were zero cases of COVID-19 infection in receiving communities as opposed to the situation in sending communities where few cases had been discovered. Among the immigrants in sending communities, some of them contacted COVID-19, while among the indigenes, many contacted the disease. Contrastingly, in receiving communities, no incidence of COVID-19 infection among the indigenes. This is consistent with the pull of the immigrants from the sending communities to the receiving communities. This is attributed to the remoteness of the receiving communities, where people seldom travel from to urban markets, unlike the situation in large rural (sending communities) where people interact frequently with urban traders. In the remote (receiving) communities, few middlemen were received from larger rural and urban communities. Social distancing naturally exists in remote rural communities because of the scanty population as well as the people’s reverence of the leadership of such communities. This prompts the religious use of safety gear such as nose and mouth mask.
In terms of yield, very minute difference was observed between the sending and receiving communities. While the mean yield per hectare in sending communities was 1394.91, mean yield of 1395.12 was recorded in receiving communities. Many farmers in sending communities practiced intercropping in their farm plots, while Most of them practiced it in receiving communities. This is consistent with the fact that intercropping is their traditional cropping system, and the receiving communities were more inclined to traditional practices. More people used improved seeds in their farm plots in sending communities than in receiving communities. While improved seeds were planted in many farm plots in sending communities, they were planted in fewer farm plots in the receiving communities. This trend is attributed to the fact that sending communities were closer to urban communities than receiving communities that are remote.
In sending communities, female headed households were fewer than in receiving communities. In receiving communities, more households were headed by females, as opposed to the situation in receiving communities. This is consistent with the fact that many women in the receiving communities were widows and those the husbands had left to work in the cities only to visit home probably once in a month, since the communities were far from cities. The household heads in receiving communities were older than those in receiving communities. This is so because the average age of household heads in sending communities was slightly than that of the household heads in receiving communities. This is because migration is age specific (Ofuoku & Chukwuji, 2012).
Receiving communities had higher household sizes than sending communities. This is consistent with the larger farm areas in receiving communities than the smaller farmland areas in sending communities. The family needs called for larger farmland cultivation by larger sized households.
3.3. Inferential Statistics
Influence of COVID-19 and receiving communities characteristics on migration
Table 4 presents results on the influence of COVID-19 and selected characteristics of receiving communities on migration. We found that the effective lockdown order in sending communities influenced migration of farming households to receiving communities which are characteristically more remote than sending communities. In the more remote communities, government officials were not noted as being there to enforce the lockdown order. The reason being difficulty in accessing such settlements and the population density which they thought was too sparse to encourage rapid spread of COVID-19. The restrictions on movement became more stringent as the disease progressed in its spread causing breakdown in farming activities and shortage of labor (Siche, 2020;Abdelhedi & Zowari, 2020).
The lockdown observance restricted the movement of people thereby depriving them of access to the base of their livelihood activities. This stopped people from carrying out their livelihood activities. The lockdown commenced during the beginning of farming season and farmers were already engaged in farm operations. For fear of losing out, they migrated, farmers decided to emigrate from their home rural communities to more remote communities where the citizens had the freedom to access their farms and carry out their farming activities.
The fear of contacting COVID-19 was another variable that influenced the migration of the farm families from the sending communities, which are more or less large rural communities to the remote rural communities that are sparsely populated). This implies that the fear of being infected with COVID-19 formed a push factor that prompted farm families to immigrate to remote rural settlements. Since the pandemic became popular during the beginning of the farming season, the decision to move from their sending communities was not difficult for them to take. The continuous spread of the disease contributed to the fear that made the farming families to move to their present more remote communities, as it created fear in them. They had to relocate to sparsely populated remote communities.
Uncontrolled movement in the remote (receiving) communities was a pull factor that influenced their migration there. Since there was little or no restrictions on movements, farm families embarked on migration to those rural communities where they can easily access their farms to carry out their farming activities. During a narrative, most of them voiced out that they left to the receiving communities in order to avoid the restrictions on their movements to farm and that they would have lost out if not for their immigration to the receiving or host communities.
Another factor they considered to immigrate to their host communities was the fertile soil there. There remote rural communities have farmlands that are left fallow for many years as they practice shifting cultivation. The fallow period led to the replenishment of soil nutrients which are the major factors in crop farming. Though lack of proximity to markets had all the years before COVID-19 pandemic had discouraged their relocation to the receiving communities. However, with a discouraging situation to them in the sending communities, they had no option than to migrate to the remote rural communities that possesses fertile soil.
Land availability in the receiving communities likewise influenced their immigration there. Availability of plenty farmland allows the practice of shifting cultivation in the receiving communities. The implication is that availability of abundance of farmland is an index of the practice of shifting cultivation. With the abundance of farmland in the receiving communities, they had no difficulty acquiring land for their farming activities.
Table 4 Influence of COVID-19 and receiving community characteristics on migration
Variables | t-statistics | Significance |
Lockdown | 4.829 | 0.0597*** |
Fear of contacting COVID-19 | 3.423 | 0.00771*** |
Uncontrolled movement | 1.214 | 0.0349** |
Soil fertility | 2.337 | 0.0232* |
Land availability | 1.654 | 0.000982** |
Number of incidence of COVID-19 | 2.652 | 0.0703*** |
Observations | 517 | |
R2 | 0.751 |
P- value. Ho: COVID-19 and characteristics of receiving communities have no influence on migration
Note: t-statistics computed using robust standard errors.
*** is significant at 1%, ** at 5% and * at 10%.
The number of COVID-19 incidents in the sending communities also significantly related with the relocation of farm families to receiving communities. This is related to the fear of contacting the disease. This confirms the influence of the fear of contacting the disease on migration to remote rural communities.
Impact of COVID-19 on migration
Figure 1 shows that the rate of migration increased with increased incidents of COVID-19. This implies that the decision to migrate from sending communities was significantly influenced by the incidents of the disease in the sending communities. This is consistent with Ekong (2006) and migration theories that state that people migrate because of pandemics and other natural occurrences. This confirms the results of the regression on influence of COVID-19 on migration from sending communities. COVID-19 is here shown as having influenced migration from the sending communities, where there were incidents of COVID-19 to receiving communities where there was no incident of COVID-19.
3.4. Relationship between COVID-19 induced rural-rural migration and agricultural productivity
Table 5 presents the relationships between migration and yield of crops in both the sending and receiving rural communities based on different criteria. In the sending communities, out-migration had an inverse relationship with yield, implying that in the presence of such migration, farm yields were reduced. As emigration increased, farm yields decrease. This trend is because of the vacuum vcreated by the out-migrated farmers. Contrarily, the relationship between in-migration and farm yields was positive, implying that such inflow of farm families increased the farm yields. As farm families migrated into the receiving communities, collective farm yields increased. This trend is attributable to the gap filled by in-migrated farm families. This is in accordance to a priori expectations.
Distance to farm (in minutes) had negative effects on the yield in both sending and receiving communities. However, the relationship is an inverse one which implies that the shorter the time taken to access farm plots, the higher the farm yields. This trend is because of the time and energy used for farming activities. Energy expended in movement to the farm plots robbed the farmers of energy needed for farming operations.
Farm size (in hectares) had positive relationships with yield. This means that larger farm sizes contributed to higher yields in both communities. The larger the farm size, the higher the yields of crops harvested from the farms. This is in consonance with the findings of Zhang (2020), FAO (2020b). This indicates that larger farm cultivation results to higher yields. Ages of the farming household heads had negative relationship with yields. This is an indication that as the household heads get older, farm yields decrease. On the other hand, the younger the household heads the higher the yields from their farms. This trend was observed in both the sending and receiving communities. This means that older farmers did not have enough energy to carry out farm operations, while the younger ones had that required energy as they were energetic.
The assets owned by farmers in sending and receiving communities had positive relationship with the yields from the two sets of communities. Greater assets led to higher yields in both sets of communities. Assets owned by the farmers contributed to the yields in both communities positively as these assets are used most times for farm operations. These assets are in form of cash and materials. This is congruent with Mwesigye & Matsumoto (2013) who found that assets contributed to the farm yields among migrant farmers in both sending and receiving communities in Uganda.
Impact of migration on agricultural productivity
Migration impacted negatively on agricultural productivity in sending communities and positively in receiving communities (Figure 2). As agricultural productivity in receiving communities increased, that in sending communities decreased up to an optimal point before it started declining, though slightly. Emigration from sending communities reduced the population of farmers and farm laborers. This led to reduction in agricultural productivity in such communities, while in-migration to receiving communities witnessed the entrance of farmers and farm laborer’s occasioning increased level of agricultural productivity, hence enhanced agricultural productivity. Migration from rural communities leads to shortage of agricultural labor in sending communities and abundance of farm labor in receiving communities (Ekong, 2006).
4. Conclusions
COVID-19 cases induced immigration into receiving communities. Migration, in turn increased productivity in receiving communities and reduced productivity in sending communities. The results of this study are suggestive of significant cases of migration from sending communities, because of COVID-19 related factors, which weakened agricultural productivity in sending communities, while it strengthened productivity in receiving communities. The effect will be higher if the immigrants in the receiving are of diverse ethnic background. It was indeed, found that with the presence of migrant farmers in the receiving communities, farm sizes increased. However, migrants, apart from COVID-19 related factors were influenced by fertility of soil and availability of land in receiving communities. Conclusively, rural-rural migration induced by COVID-19 impacted on agricultural productivity in sending and receiving communities, apart from this, other factors such as household and community factors also contributed to agricultural productivity.
These findings have some important implications. For instance, the use of state and local security formations in large rural communities to enforce the COVID-19 lockdown did not put into consideration the fact that farm families naturally observe social distance while carrying out farm operations. The government ought to have considered this and relax it for farm families going to attend to their farms.
Peeved with the level of extortion from state and local community security personnel in sending communities, people were discouraged from attending to their farms. In the case of future, mechanisms should be established to check this obnoxious attitude. For future reason, the one - way communication of the government, sensitizing the populace of COVID-19 should be changed to an interactive one. This is suggested so that alternative ways to prevent the current scenario instead of total lockdown inclusive of farming activities for all classes of farmers should be sought. Only livestock farmers were excused during the lockdown enforcement in Nigeria. It is suggested that a similar study should be conducted after recovery from this COVID-19 pandemic era.