1. Introduction
Fusarium wilt (Fw) is a highly destructive disease that affects banana plants (Musa spp.) globally. Their pathogen responsible is Fusarium oxysporum complex (Garcia et al., 2018). Recently, Maryani et al. (2019) identified different Fusarium isolates causing banana wilt, which were later merged into the Fusarium oxysporum complex. One of these isolates, Fusarium odoratissimum, is referred as F. oxysporum f. sp. cubense Tropical Race 4 (FocTR4) (Carvalhais et al., 2019). FocTR4 is a soilborne pathogen that can survive without a host for extended periods (Bishop & Cooper, 1983). This fungus infects the root systems of banana plants and spreads to the vascular tissue, causing water stress, severe chlorosis, and wilting (Ploetz, 2015). FocTR4 is particularly harmful to Cavendish and other subgroup banana plants grown in tropical and subtropical regions, including parts of Australia, the Canary Islands, and South Africa (Mostert et al., 2017; Ploetz et al., 2015). Fw is a challenging disease to manage due to the limited efficacy of current control methods.
Despite some studies indicating a reduction of Fw with the use of biological control agents, currently, there is no management measure that can effectively eradicate FocTR4 once it has been detected and established in a field. Furthermore, the lack of FocTR4-resistant genotypes makes this pathogen a problem for banana production (Olivares et al., 2021). The fungus develops at temperatures between 9 and 38 °C, with optimum growth occurring at 23 - 27 °C (Baharum et al., 2018). Fw is most severe during warm and rainy months, but environmental conditions and soil type also influence its spread (Brake et al., 1995; García-Bastidas et al., 2019). However, the susceptibility of the cultivated Musa plants in the area is the most significant factor in FocTR4's impact on banana production, with losses reaching 94 billion USD worldwide (Martínez et al., 2023; Rodríguez-Yzquierdo et al., 2023; de Figueiredo et al., 2023).
Until recently, FocTR4 was not present in Latin America. In 2019, wilting, leaf chlorosis, and vascular discoloration, typical symptoms caused by FocTR4, were observed in 100 Cavendish plants in northeast Colombia (García-Bastidas et al., 2020). FocTR4 presence was also reported in Cavendish plants of one farm in Querecotillo, Peru, with typical symptoms of Fw (Acuña et al., 2022). In July 2022, severe yellowing and foliar wilting, and pseudostem vascular discoloration were found in Cavendish banana plants cultivar 'Valery' in the Aragua, Carabobo, and Cojedes states, Venezuela. Subsequently, the FocTR4 presence in this country was confirmed (Herrera et al., 2023). How FocTR4 entered the Americas is not known at this time. However, the Colombian (six) and Peruvian (four) isolates are distributed in two different clades. For example, the Colombian isolates represent one clade, while the Peruvian isolates represent a different clade. This means that the introductions of the disease to the continent in Colombia and Peru are separate and independent (Reyes-Herrera et al., 2023).
This raises concerns for banana production in Ecuador as it is located between Colombia and Peru. The vulnerability of banana plants in the country is particularly high as it has only reported the presence of F. oxysporum race 1 (Magdama et al., 2020), the pathogen that affects only Gros Michel plants. Currently, the presence of FocTR4 in neighboring countries of Ecuador, increases the risk of disease spread due to the constant flow of people, including tourists, and commercial transactions, having imperative to conduct a thorough risk analysis and review the current scenario to implement effective measures for disease prevention and control (Martínez-Solórzano et al., 2020). In a recent study by Ibarra-Zapata et al. (2021) on modeling the spatial pattern of FocTR4 in Pantropical America, they found that Ecuador's phytosanitary risk is alarmingly high, measuring above 0.9 (maximum is 1). Despite the importance of FocTR4 for Ecuador, until now, there is no national risk map for this biotic problem. The study of disease dynamics and spatial distribution is essential for understanding pathogen biology, implementing effective prevention and manage-ment strategies, and identifying risk factors.
For instance, in a study by Salvacion et al. (2019), the suitability of banana crops and the potential distribution of FocTR4 in the Philippines was evaluated under current and future climate conditions using fuzzy logic and the maximum entropy approach. They found that future changes in rainfall patterns due to climate change may expand the areas that are suitable for the occurrence of Fw, highlighting the need for effective strategies to prevent the spread of the disease. Fw is a disease that exhibits an aggregative spatial pattern, as it can spread rapidly to neighboring and distant plants. While studying the dynamics and spatial distribution of FocTR4 within a region or country is crucial, it is not feasible in countries like Ecuador, where the disease is not yet present. Instead, a future risk analysis could be conducted to evaluate the potential impact of the disease and implement preventive measures.
The emergence of FocTR4 in Latin America poses a significant threat to Ecuador, the world's leading exporter of bananas and plantains, which represented an annual average of one-third of the total global banana export volume between 2014 and 2020, worth 4.2 billion USD (Herrera et al., 2021; Hutter et al., 2021). Ecuador's banana production is primarily based on the family economy (Vaca et al., 2020). Given the significance of Musaceae crops for Ecuadorians, we must conduct a comprehensive risk analysis for FocTR4 in Ecuador. This assessment not only enhances agricultural practices in banana crops but also identifies suitable areas for new farm locations, providing more opportunities to manage the coexistence of FocTR4 with banana and plantain producers in the country (Olivares et al., 2021). The present study aims to identify agroclimatic zones favorable for FocTR4 in Ecuador to develop national pest strategies. The specific objectives were a) to test linear models to evaluate the incidence of the disease related to climatic conditions and, b) to map FocTR4 favorable zones in Ecuador.
2. Methodology
2.1. Study area
The study area chosen for this research was the entire land surface of Ecuador (Figure 1a). Ecuador is a South American country located on the equator line, with its territory divided between the northern and southern hemispheres and coordinates of -2.20 latitude and -79.89 longitude. The country is bor dered by Colombia to the north, Peru to the south and east, and the Pacific Ocean to the west, spanning a total geographical area of 256,370 square kilometers. Ecuador comprises four regions, consisting of 24 provinces and 221 cantons. Most of the banana producers in Ecuador are small businesses, with medium-sized producers accounting for a total of 95.6%. The main banana and plantain producing provinces in Ecuador are Guayas, Los Rios, El Oro, Manabi, and Esmeraldas. These provinces have different altitudes ranging from 10 to 300 meters and feature tropical savanna (Aw), semi-arid (BSh), tropical monsoon (Am), and tropical rainforest (Af) climates, respectively, according to Köppen (1918).
2.2. Climatic data and climatological water balance
To conduct the agroclimatological analysis, gridded data from 20 banana points (BP) was extracted using land use and land cover maps and random points tools, covering the entire territory of Ecuador (Hugentobler, 2016; MAE-MAGAP, 2015). Daily precipitation data from the Climate Prediction Centre/ National Oceanic and Atmospheric Administration CPC/NOAA with a resolution of 0.5° lat x 0.5° lon from 1980 to 2022 was used (available at https://psl.noaa.gov/data/gridded/data.cpc.globalprecip.html) (Chen & Xie, 2008). The daily air temperature was obtained from the European Center for Medium-Range Weather Forecasts (ECMWF) ERA5 Reanalysis with a resolution of 0.25° lat x 0.25° lon from 1980 to 2022 (Hersbach et al., 2019). The analysis of long-term climatic data was focused on the month’s most favorable for F. oxysporum f. sp. cubense race 1, according to the assessment by the Ecuadorian National Plant Protection Organization (NPPO) (Agrocalidad), which are April, May, June, July, and August (Parsons, 1957).
The Thornthwaite and Mather method was used to calculate soil water availability, considering a soil water holding capacity of 100 mm for all banana points. This method accounts for the different types of soil found in the BP and is commonly used in agro-climatological studies to measure disease risk (Goss & Richards, 2008). All calculations were carried out using R software and Interactive Data Language (IDL) on a 10-day timescale.
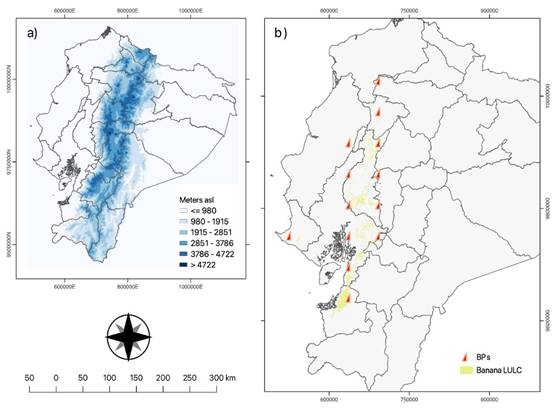
Figure 1 Study area. (a) Map of Ecuador showing the digital elevation model in meters above sea level. The colors represent the elevation, with warmer and cooler colors indicating higher and lower elevation, respectively. (b) Map of banana points (BPs) in cantons showing land use and cover. The points are shown as orange triangles. The digital elevation model data and the banana points were obtained from the Ecuadorian Militar Geographical Institute (IGM).
2.3. Fusarium oxysporum f. sp. cubense tropical race 4 occurrence assessment
The occurrence of Fw caused by FocTR4 was estimated by calculating the growing degree days (k, °C) for each month, with emphasis on the months with the greatest risk of disease, namely April, May, June, July, and August. The calculation was based on the equation proposed by Arnold (1959):
Eq. 1where: TM is maximum daily air temperature (°C), Tm is the minimum daily air temperature (°C), Tb is the minimum threshold temperature (°C) (here we used 17 ° C) (Nelson, 1991), n is the total number of days of each month and k j is the growing degree days of each month j, and; a total number of cycles per month which represents the possible number of total pest cycles according to the calculated BP climatology, was calculated according to the following equation:
Eq. 2where: NC month is the number of cycles per month, TM, Tm, Tb, and kj are as defined above. Using air temperature and calculated water balance data, the main climatic conditioning factors that influence the occurrence of Fw caused by FocTR4 were determined.
2.4. Examining the relationship between incidence and climatic conditions
The relationship between Fw incidence and climatic conditions was investigated through correlation analysis on disease occurrence scores (computed from Eqs. 1 and 2) for 20 banana plantations and their respective climatic data from 1980 to 2022. The statistical variables used to explain Fw incidence were obtained from Pegg et al. (2019) and Sentelhas et al. (2016) and include the following:
1. Number of 10-day periods with mean air temperatures between 17 and 33 °C (NPT1733)
2. Number of 10-day periods with mean air temperatures between 20 and 28 °C (NPT2028)
3. Number of 10-day periods with mean air temperatures between 24 and 34 °C (NPT2434)
4. Number of 10-day periods with water surplus between 50 and 90 mm (NPWS5090)
5. Number of 10-day periods with water surplus above 100 mm (NPWS100)
Linear models in both simple and quadratic forms were tested:
Eq. 3Eq. 4Here, Inc represents the incidence of Fw, and X1 and X2 are the climatic variables (NPT1733, NPT2028, NPT2434, NPWS5090, NPWS100). The coefficients a, b, c, d, e, and f were estimated using the linear models. To ensure the accuracy of the models, Fw incidence data was generated using a climatic data set for August to November and compared with simulated data. To evaluate the performance of the models, various measures of goodness-of-fit were employed, including the coefficient of determination (R2), mean error (ME), mean absolute error (MAE), root mean square error (RMSE), normalized mean square error (RMSEn), index of agreement (d), and modified index of agreement (d1). These measures were used to determine the best model, as recommended by Willmott (1982) and Sentelhas et al. (2016):
Eq. 5Eq. 6Eq. 7Eq. 8where P i and Q i refer to the predicted and calculated data respectively, n is the number of observations, M is the mean of the observed occurrence, and |P 𝑖 ´ | = P 1 - M and |𝑄 𝑖 ´ | = Q 1 - M.
2.5. Agroclimatic favorability index
Using Eqs. 3 and 4, yearly data (2000-2022) was calculated for all BPs. This data was normalized on a scale of 1-7 (based on Pérez-Vicente et al., 2014) to simulate the ranges of FocTR4 infection (1: 5% to 7: 100%). The agroclimatic favorability index (AFI) was then calculated using Equation 9 to identify the regions in Ecuador most vulnerable to TR4:
Eq. 9where AFI calc is the calculated favorability index for Fw, Yn is the number of years with a given score n, Y is the total of years used in the calculation, and 0, 1, 3, 6, and 10 are empirical factors for weighting the disease. Ranges go from 0 (not favorable) to 10 (most likely favorable for disease incidence) (Sentelhas et al., 2016).
Finally, AFI calc values were then correlated with geographic latitude, longitude, and altitude using the following equation:
Eq. 10where AFI est is the agroclimatic favorability index estimated, 𝜙 and 𝜆 are latitude and longitude (degrees), 𝜉 is the altitude (m) and a, b, c, d, e, f, g, h, i, and j are the coefficients of the multivariate linear model.
The results from the modeled AFIest values were compared to the actual AFIcalc values to obtain the goodness-of-fit measures (GOFs) between data sets. In addition to the GOFs mentioned in 2.4, the Kling-Gupta efficiency (KGE) was calculated to analyze the correlation, bias, and variability of the data. The KGE was used to evaluate the performance of the model, and to determine how well it represents the observed AFIcalc values.
2.6. Agroclimatic favorability index maps for Ecuador
The estimated model results (2.5) were used to generate maps of the agroclimatic favorability index (AFI) for Ecuador using Qgis software. Altitude data was obtained from a digital elevation model (DEM) (30 m x 30 m resolution) of the Ecuadorian Militar Geographical Institute (IGM) generated from IGM, Pronareg/Ingala/Orstom, Petro Ecuador Project. Five data sets (DEM_30_Set 1, 2, 4, and 5) with 6-7 orthophotographs each were combined and transformed into a single raster (Geotiff) using the merge tool from the raster toolbox. The original coordinate reference system of the data was PSAD56/UTM zone 17S, so the raster was reprojected to WGS 84/UTM Zone 17S for consistency with the political data. Longitude and altitude were obtained from the dig ital elevation model (DEM) using GrassGIS version 7. The multivariate linear model results were used to create AFI maps for Ecuador, which were then classified into 5 categories: low (AFI ≤ 2.0), medium-low (AFI 2.1 - 3), medium-high (AFI 4.1 - 6), high (AFI 6.1 - 8.0), and very high (AFI > 8.0) for better un derstanding. The images were saved in Geotiff format, polygonized, and used to generate the final maps (Bivand et al., 2016).
2.7. Model Validation
To validate the AFIest model, we compared its results with those of Maximum Entropy (MaxEnt) species distribution modeling. We used environmental parameters from WorldClim version 2 (Fick & Hijmans, 2017) and current data from the CABI database for known FocTR4 locations (Mayotte, Mozambique, Indonesia, Papua New Guinea, China, Lebanon, Israel, Laos, Pakistan, Colombia, and Peru) (Table 1) to minimize limitations in MaxEnt modeling.
To extract data for validation, we created 1000 random points using QGIS and intersected them on AFIest and MaxEnt maps. The data pairs were used to perform cross-validation using the R carat package (Kuhn, 2008,2015) with a calibration grid of parameters for various classifications (n = 100) and regression routines. The models were fitted and resampled based on performance measures.
3. Results and discussion
3.1. Fusarium wilt occurrence assessment
The results of the Fw cycles, based on climatic conditions are presented in Table 1 and Figure 2. The primary banana-producing regions, Guayas, Los Rios, and El Oro, exhibited several NPTs for different temperature ranges and NPWS levels. On average, Guayas had 9.3 NPTs, Los Rios had 8.2 NPTs, and El Oro had 7 NPTs. For NPWS, 3 were calculated for Los Rios, 3.7 for Guayas, and 4 for El Oro. It is important to note that the range of NPT1733 was consistent across all points and was not considered for the NC model estimation.
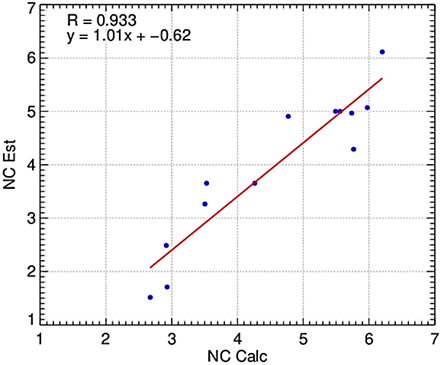
Figure 2 Relationship between the number of Fusarium wilt (Fusarium oxysporum f. sp. cubense tropical race 4) cycles estimated with the best performance agroclimatic model (Incest) for BPs.
The use of models to study disease intensity is a useful approach for understanding important aspects of observations or dimensions of interest, even in small populations such as plants or crops. To generate data for regions that are currently free of pests, we used experimental data from small plots at other latitudes, a common approach in epidemiology research to make inferences and understand fundamental relationships (Horgan et al., 2014; Portalanza et al., 2019). We employed empirical models developed by Sentelhas et al. (2016) and Yamada & Sentelhas (2014) to define the occurrence of FocTR4 using statistical principles and found that these models provided a good fit to the calculated value and were useful for determining variable correlation. The process of developing the model involved four steps: data acquisition, describing the intensity, evaluating the goodness of fit, and using the selected model to predict past and future values and compare the results (Madden et al., 2017).
Here, we relied on modeled mean air temperature reanalysis data to estimate Fw intensity, as daily weather observations are not always available in certain areas, making crop disease monitoring challenging. Our calculated Fw intensity values showed a good match with actual banana cropped areas. The limited spatial representativeness of INAMHI's land weather stations made reanalysis a more common choice for research studies (Toreti et al., 2019), as reanalysis provides a valuable source of information for crop monitoring. Studies have shown good performance for crops such as maize and wheat, with correlations higher than 0.6 for European regions (Barzman et al., 2015; Hmimina et al., 2013). However, other databases, such as NOAA NCDC, Berkeley Earth time-series data, or CEDA, may face difficulties in forecasting biotic factors, lack complete yearly data, or have a coarse resolution (> 0.5º).
We need to keep in mind that cropping year, district, cultivar, crop density, planting date, weeding, sucker and bud management, source of moisture and planting materials, presence/absence of wilt in neighboring farms, disease management practices, farmer's awareness towards wilt diseases, planting date, and cropping system are significantly associated with Fw incidence (Mengesha et al., 2018). These activities, allied to the pathogen's characteristics of growing saprophytically in surrounding tissues as diseased plants die, forming mycelium, conidia, and chlamydospores that help it spread through soil and water, using vector insects such as the Cosmopolites sordidus weevil, among others (Dita et al., 2018; Rodríguez-Yzquierdo et al., 2023; Martínez et al., 2023), could imply that in case FocTR4 enters Ecuador, the pathogen has the potential to spread rapidly throughout the national territory. We must consider that the impact of FW is broad and proportional to the containment of the disease and the financial capacity of producers to simultaneously face yield losses and increased production costs of the fruit (Martínez et al., 2023).
3.2. Fusarium wilt incidence based on climatic conditions
Based on the evaluation of Fw from 20 BP, four linear models were developed, with intensity and climatic conditions as dependent and independent variables, respectively. The models were considered in a simple form (SF) and an integrated form (IF) and are presented as follows:
The statistical coefficients of the models are presented in Table 2.
Table 1 Number of calculated Fusarium wilt (Fusarium oxysporum f. sp. cubense tropical race 4) cycles (FwC) and number of 10 days occurrence for air temperature (NPT) and water surplus (NPWS) for BPs climatic normal
Site | NPT1733 | NPT2028 | NPT2434 | NPWS5090 | NPWS100 | FwC |
---|---|---|---|---|---|---|
Bolivar | 13 | 7 | 0 | 10 | 0 | 2.6739 |
El Oro | 12 | 8 | 0 | 7 | 0 | 2.9298 |
Guayas 1 | 12 | 10 | 0 | 6 | 0 | 3.5056 |
Guayas 2 | 12 | 12 | 0 | 4 | 1 | 4.2635 |
Guayas 3 | 12 | 12 | 10 | 0 | 8 | 5.9766 |
Guayas 4 | 14 | 12 | 9 | 0 | 8 | 6.2028 |
Los Rios 1 | 12 | 9 | 0 | 9 | 0 | 2.9173 |
Los Rios 2 | 12 | 9 | 0 | 8 | 0 | 3.5304 |
Los Rios 3 | 12 | 12 | 8 | 7 | 0 | 5.7380 |
Manabí | 13 | 12 | 7 | 2 | 5 | 5.5615 |
Pichincha | 12 | 12 | 4 | 0 | 0 | 5.4933 |
Sto. Domingo | 12 | 12 | 0 | 2 | 0 | 4.7731 |
Sta. Elena | 12 | 12 | 9 | 2 | 8 | 5.7675 |
Table 2 Linear and angular coefficients of the multivariate linear models for estimating Fusarium wilt (Fusarium oxysporum f. sp. cubense tropical race 4) occurrence, considering the simple (Sf) and integrated (If) variables for Ecuador
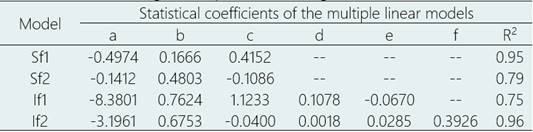
Table 3 Models’ performance evaluation measures for Fusarium wilt (Fusarium oxysporum f. sp. cubense tropical race 4) incidence
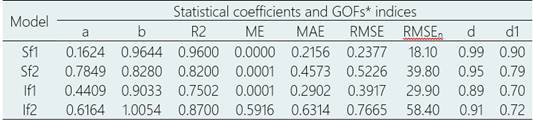
*Goodness of fit measures; ME, mean error, MAE RMSE nRMSE, normalized root means square error, d, Willmott index of agreement, d1, a normalized index of agreement.
Table 4 Estimated of Fusarium wilt (Fusarium oxysporum f. sp. cubense tropical race 4) scores for main banana regions in Ecuador for the period 2000-2022
year | g1* | g2* | g3* | g4* | lr1* | lr2* | lr3* | eo* |
---|---|---|---|---|---|---|---|---|
2000 | 4 | 3 | 3 | 4 | 3 | 4 | 1 | 4 |
2001 | 2 | 1 | 3 | 4 | 2 | 1 | 1 | 2 |
2002 | 2 | 4 | 3 | 4 | 3 | 4 | 1 | 3 |
2003 | 2 | 4 | 3 | 5 | 1 | 2 | 2 | 3 |
2004 | 2 | 7 | 7 | 7 | 1 | 4 | 7 | 2 |
2005 | 1 | 7 | 7 | 7 | 1 | 4 | 7 | 1 |
2006 | 5 | 5 | 3 | 4 | 5 | 5 | 1 | 5 |
2007 | 4 | 5 | 3 | 4 | 3 | 4 | 1 | 4 |
2008 | 6 | 5 | 3 | 4 | 5 | 6 | 1 | 4 |
2009 | 7 | 6 | 5 | 6 | 4 | 7 | 4 | 3 |
2010 | 3 | 5 | 3 | 5 | 1 | 5 | 2 | 2 |
2011 | 5 | 5 | 3 | 4 | 5 | 6 | 2 | 6 |
2012 | 7 | 7 | 7 | 7 | 6 | 7 | 7 | 5 |
2013 | 5 | 4 | 3 | 4 | 5 | 6 | 1 | 5 |
2014 | 6 | 5 | 3 | 4 | 7 | 6 | 1 | 7 |
2015 | 6 | 5 | 3 | 4 | 7 | 6 | 1 | 7 |
2016 | 6 | 5 | 1 | 1 | 6 | 6 | 1 | 6 |
2017 | 6 | 5 | 3 | 5 | 3 | 6 | 2 | 4 |
2018 | 5 | 7 | 6 | 6 | 4 | 6 | 6 | 2 |
2019 | 4 | 6 | 5 | 6 | 4 | 6 | 6 | 4 |
2020 | 5 | 6 | 5 | 6 | 5 | 6 | 6 | 5 |
2021 | 5 | 6 | 6 | 5 | 5 | 5 | 5 | 6 |
2022 | 5 | 5 | 6 | 6 | 4 | 6 | 6 | 5 |
Average | 4 | 5 | 4 | 5 | 4 | 5 | 3 | 4 |
*Guayas 1, 2, 3, and 4, Los Ríos 1, 2, and 3, El Oro. *Standard deviation.
The results showed that the linear model using integrated variables of NTP2028, NTP2434, NPW5090, and NPW100 had the best fit for estimating the Fw incidence in the 20 banana points in Ecuador. This model had a high precision (R2 = 0.87) and accuracy (d = 0.91) with a normalized root mean square error of 58%. These results suggest that the model can be used to estimate Fw incidence in Ecuador based on climatic conditions (NTP2028, NTP2434, NPW5090, and NPW100).
3.3. Estimated agroclimatic favorability index for Fusarium wilt (Fusarium oxysporum f. sp. cubense tropical race 4)
The integrated multiple linear models that best fit the estimated FwC from Table 3 were used to estimate the disease for a period of 18 years (2000-2018) and normalized on a scale of 1-7, corresponding to the FocTR4 bibliography ranges of infection (Table 4). On average, all banana regions scored between 3 and 5 (sd=1.45-2.34), indicating a medium to high risk of FocTR4 estimates. The years between 2006 and 2011 had a higher amount of rainfall and were warmer based on climatology BP calculations. In contrast, 2004 and 2005 were drier and cooler years, with an overall score of about 1 and 2. The BPs of Los Rios, Guayas, and El Oro, which have wetter climates, showed variability between them. For instance, in Guayas 1, most years (80%) scored between 2 and 6, indicating a medium-high to very high risk for FocTR4 estimates, while Los Rios 3 showed values between 1 and 4 (60%) in most years.
Although the Ecuadorian government has been implementing inclusion measures since the first report of FocTR4 in Colombia, these controls must be more rigorous and continuous on the border with Peru, where there are countless clandestine crossings. These include the use of quaternary ammonium salts (Zhang et al., 2023), particularly along the borders with Colombia and Peru, and in many of Ecuador's banana plantations. Also, the measures should not only prioritize the non-transit of banana or plantain propagation material, but also other ornamental plant species such as Aglaonema pictum, Canna edulis, C. indica and Hedychium coronarium that could harbor FocTR4 (Waman et al., 2013; Ortega & Uribe, 2023), and that are very desired (especially the first one) to use them in gardens in Ecuador.
The results of our study have significant implications for banana crop management in Ecuador. By predicting potential future occurrences of FocTR4, our study can assist in developing a proactive approach to managing the disease. The identification of hotspots in Los Ríos, Guayas, and El Oro provinces, where most Ecuadorian banana producers are concentrated, is particularly valuable for targeting disease management strategies. While the Ecuadorian government has implemented exclusion and biosecurity measures, further steps are necessary to prevent future losses, which are expected to be substantial. Improved methods for preventing the introduction and spread of FocTR4 are needed to protect Ecuador's banana industry. By providing a model for predicting disease occurrences and mapping potential epidemics, this study can contribute to the development of more effective strategies for managing and preventing FocTR4 in Ecuadorian banana crops (Scheerer et al., 2018; Staver et al., 2020). FocTR4 prevention and containment programs must be complied with, but at the same time it is necessary to advance in the knowledge of the multiple factors related to this situation (Martínez et al., 2023).
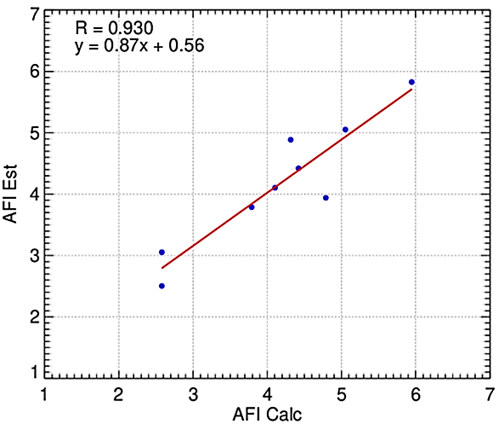
Figure 3 Relationship between agroclimatic favorability index estimated (AFIest) and the calculated (AFIcal) agroclimatic favorability with the best performance AFI model (AFIest) for BP.
The estimated disease data scores from Table 5 were used to calculate AFIcal based on the number of years with a specific score (Equation 9) and then used to generate the best-fit model to estimate AFI as a function of geographical coordinates and altitude (Figure 3). The best-performing model was the equation 11 (/).
Table 5 Coefficients of the multivariate linear model for AFI as a function of geographical coordinates and elevation. Goodness of fit indices for validation data set
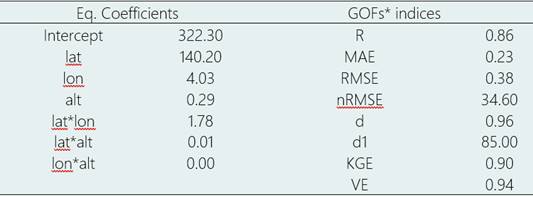
*Lat = latitude, Lon = longitude, alt = altitude, R2 = coefficient of determination, MAE = mean absolute error, RMSE = root mean square error, RMSEn = normalized mean square error, d = Willmott index of agreement, d1 = normalized index of agreement, KGE= Kling-Gupta efficiency, and VE= Volumetric efficiency.
The recent occurrence of FocTR4 in commercial farms in the Colombian Guajira region (García-Bastidas et al., 2019), in Querecotillo, Peru (Acuña et al., 2022), and in the Aragua, Carabobo, and Cojedes states, Venezuela (Herrera et al., 2023), the potential risk of the spread of this problem to Ecuador has increased. The current situation in the Americas is further complicated by the recent report of the pathogen in Venezuela (Martínez et al., 2023). The proximity of the banana-producing border areas of El Oro province, Ecuador, to the FocTR4 outbreaks in Peru - less than 242.7 km - has heightened the potential risk of the disease spreading to this region. In general, in these border areas, government policies such as exclusion should be implemented, which is one of the priority measures to prevent the movement of propagation material, establish quarantine sectors on time, and promote awareness campaigns aimed at producers about the threat posed by FocTR4 (Dita et al. 2017).
Here, AFIest represents the estimated agroclimatic favorability index, ϕ and λ denote latitude and longitude (in degrees), and ξ represents the altitude (in meters). The coefficients of the multivariate linear model presented in Table 5, denoted by a, b, c, d, e, f, g, and h, were used in Equation 11. This model showed the best precision (R2 = 0.86) and index of agreement (0.96), and the lowest MAE (23%), nRMSE (38%), and highest KGE (0.90) compared to other integrated tested models.
3.4. Agroclimatic favorability index map
The results of AFIest for Fw are shown in Figure 4. Most of the provinces where bananas are produced, such as Guayas, Los Rios, El Oro, Manabi, Santa Elena, and Esmeraldas, had a medium to very high-risk estimate. In Guayas and Los Rios, the agroclimatic favorability zones for very high-risk areas represent almost 50% of the total area. In El Oro province, nearly 70% of the zones fall within a medium-high range. There is a general decreasing trend in favorability in higher areas, such as Pichincha, Bolivar, and the Andean region, as well as in the Ecuadorian Amazon, which has lower values compared to the coastal region.
Accurate information about the spatial distribution of plant diseases plays a critical role in effective planning and policy-making (García-Bastidas, 2022). Maps are an essential tool for managing FocTR4 and comprehending its implications. The GIS technology provides a powerful framework for monitoring and detecting the causes of new and emerging plant diseases, as highlighted by Oxoli et al. (2017). With agriculture rapidly evolving, it is crucial to prioritize research for crop health management (Savary et al., 2012). In our study, a generic FocTR4 risk identification model was developed, incorporating the complexity of host-environment interactions, and integrated with GIS to map potential epidemics in Ecuadorian banana crops. This model can serve as a useful tool for linking epidemiological theory to actual disease occurrences in the field (Madden et al., 2019).
In Venezuela, the Cavendish banana farms (clone highly susceptible to FocTR4) with high potential risk are in the municipalities Colón, Francisco Javier Pulgar, and La Cañada de Urdaneta in Zulia State, because a) they are large plantations (500-1900 ha) from the Cavendish clone; b) they present precipitation greater than 1200 mm and a range of mean daytime temperatures of 24 to 34 °C, which are adequate for the occurrence and establishment of the pathogen; c) use intensive production systems, requiring continuous movement of agricultural workers between plantations; and d) they use a single water source for irrigation, which can favor the rapid spread of the fungus within and between plantations (Olivares et al., 2021). In another study, Rodríguez-Yzquierdo et al. (2023) demonstrated that agro-environmental conditions in Colombian moss land create a favorable environment for the establishment and spread of FocTR4. The environmental factors associated with the susceptibility of Colombian moss lands to the pathogen make it imperative to implement sound biosecurity measures and sustainable agricultural practices. These characteristics are quite like those found in Ecuador, particularly on farms at Guayas, Los Rios, and Santa Elena. Nonetheless, farms at Manabí and El Oro comprise small and medium-sized farmers.
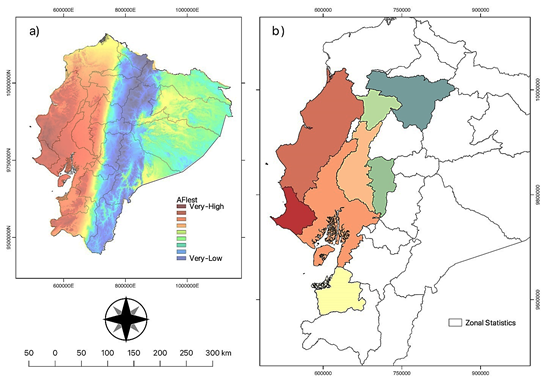
Figure 4 Agroclimatic favorability index (AFIest) for Fusarium wilt (Fusarium oxysporum f. sp. cubense tropical race 4) occurrence in Ecuador. (a) AFIest for the entire Ecuadorian domain. The AFI ranges from very high to low and is represented by a gradient color scale. Warmer colors represent higher AFI values, while cooler colors represent lower AFI values. (b) Zonal AFIest statistic for the evaluated cantons. The zonal AFIest statistic indicates the AFI value for each of the evaluated cantons.
In addition, FocTR4 has been observed in tropical countries like Thailand, Vietnam, and Cambodia (Hung et al., 2018; Zheng et al., 2018) and it is considered a serious threat to Latin America (Mintoff et al., 2021). FocTR4 can aggressively spread in both subtropical and tropical conditions, and other factors such as soil characteristics, banana cultivars present in the region, and monoculture versus mixed cultures, play a role in the development of the disease in the field (Martínez et al., 2023; Olivares et al., 2021). Climate conditions are only one of the factors in the development of Fw, but they can be a fair predictor of the disease epidemic by themselves, and they should be combined with other variables, including phytosanitary surveillance, hotspot analysis, and various linear and non-linear models.
3.5. Model Validation
The cross-validation results using the MaxEnt model showed that the RMSE was 0.1036, R2 was 0.61, and MAE was 0.0794. The first 20 resamples produced R2 values higher than 0.61, reaching up to 0.70. These results indicate that the MaxEnt model was able to predict the distribution of FocRt4 with a moderate level of accuracy. The use of environmental parameters from WorldClim version 2 and current data from the CABI database for known FocRt4 locations likely helped to minimize limitations in the MaxEnt modeling. Comparing the results of the MaxEnt model with those of the AFIest model provides further validation of the AFIest model's accuracy in estimating the occurrence of Fw based on climatic conditions.
The methodology used in this study is not novel, as it has been applied in previous studies for risk assessment (Goss & Richards, 2008). However, the process of variable selection is detailed and time-consuming, and it can provide decision-makers with valuable information to address the potential entry and spread of FocTR4 in Ecuador. It is important to note that the climatic data used in this study may not be the sole driver of disease severity and vulnerability. Further researches are necessary to fully understand the factors contributing to the severe epidemics of the pathogen in subtropical ecosystems (Li et al., 2011).
4. Conclusions
Ecuadorian provinces such as Guayas, Los Ríos, El Oro, Manabí, Santa Elena and Esmeraldas, where most of the country's bananas are produced, had an estimate of medium to very high risk. Guayas and Los Rios are the provinces with agroclimatic zones of very high risk, and about 70% of the zones in El Oro are in the medium-high range. In general, favorability decreases in the highlands (Pichincha and Bolivar) and in the Ecuadorian Amazon. However, the limitation of the study is the use of climatological data, and future studies should consider using other complete datasets with better spatial resolution to increase the accuracy of the estimated agroclimatic favorability index.