INTRODUCTION
Due to the social isolation imposed by the COVID-19 pandemic, many higher education institutions had to abruptly switch from face-to-face to online university instruction. Although distance education was a reality before the pandemic, the timely transition from traditional teaching to online teaching requires a complex approach to effectively integrate this system.
In March 2020, the COVID-19 pandemic outbreak was officially declared by the World Health Organization (WHO), according to the British Broadcasting Corporation (2020). Due to the high transmissibility of the virus, one of the recommendations to contain it was to undergo strict social isolation. In this scenario, the Brazilian Ministry of Education allowed public or private educational institutions to implement a distance learning system (Ministry of Education [MEC], 2020). This measure was applied to avoid high prejudice and delays in the academic calendar. However, it was at the discretion of the institutions whether to postpone classes or implement distance education (MEC, 2021).
According to the report of the United Nations Educational, Scientific and Cultural Organization, 1.57 billion students in 191 countries were affected by the closure of schools and universities in the same year, and those who were in a vulnerable situation suffered even more from the negative effects of this change (United Nations Educational, Scientific and Cultural Organization [UNESCO], 2020). This study by UNESCO, also shows that the main concerns of Latin American students who wished to continue their studies within this scenario were: the lack of Internet connections, the lack of financial resources, and the difficulty of maintaining an organized study schedule. Therefore, the implementation of an effective online education system is essential to reduce the harmful effects that this transition could have around the world (UNESCO, 2020).
Analysing the context of the pandemic in Brazil, it can be seen that it has had a profound impact on higher education, considering that by the end of April 2020, 30% percent of students in public and private higher education institutions (IES) had to cancel their classes (UNESCO, 2020). According to this report, 97% of private IES had an online instructional system, while only 39% percent of public IES used this modality. This information reveals that most public universities were not even slightly prepared to offer distance education, which also explains the data published by Paixão (2020), which indicates that only 6 out of 69 of Brazilian public universities managed to immediately adapt to the distance education system.
Furthermore, putting the pandemic aside, the number of truants in Brazil is worrisome. According to 2018 data from the National Institute for Educational Studies and Research Anísio Teixeira (INEP), of all the students who started studying in 2010, only 37.9% completed their studies, while 56.8% dropped out (MEC, 2019). In this note, given the extremely negative scenario that the pandemic has imposed, especially on the low-income population, it is important to understand, analyze and develop a teaching system that at least reduces the existing tendency of evading students, who have already presented relevant data just before the social isolation caused by the pandemic. In the long term, this would have a significant impact on society, with fewer qualified professionals entering the market and an increase in the number of people in social and economic vulnerability, which would jeopardize the development and growth of society as a whole.
Thus, one can see the importance of understanding the needs and pressures of the target audience when designing an educational programme or technological tool, as the success of a system certainly depends on the users’ acceptance (Almaiah & Jalil, 2014; Baloran et al., 2021). In the literature, one of the most commonly models applied to analyze and study technology adoption and implementation is the Technology Acceptance Model (Wiradinata & Israndi, 2016). According to this model, final technology adoption behavior is a function of two behavioral variables: perceived usefulness (PU) and perceived ease of use (PEOU) (Davis, 1989).
As stated by Davis (1989), PU can be understood as “the degree to which a person believes that using a particular system will improve his or her job performance” (p. 320) whilst PEOU refers to “the degree to which a person believes that using a particular system would be free of effort” (p. 320). These two variables directly affect the attitude toward use (AT), which is understood as the affection individuals have for the desired behavior, and which in turn affects behavioral intention (BI), described as the subjective likelihood that an individual will perform the desired behavior (Fishbein & Ajzen, 1975). In addition, PEOU is expected to have a direct influence on PU (Davis, 1989). The described relationships can be seen in Figure 1.
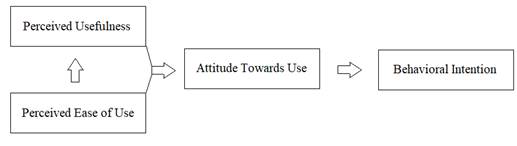
Note. Elaborated with data taken from “Perceived usefulness, perceived ease of use, and user acceptance of information technology”, by F. D. Davis, 1989, MIS quarterly, 13(3), pp. 319-340 (https://doi.org/10.2307/249008).
Figure 1 Graphic representation of the relationships proposed by TAM
In the context of online learning, two elements are emphasized in the literature: Interactivity and Cost-Effectiveness (Singh et al., 2020). Interactivity can be understood as the characteristics of digital technologies that allow the learner not only to passively observe the platform, but also to actively participate in the learning process (Radchenko & Pervukhina, 2020). This ability allows the user to keenly interact with the information carrier and change the rhythm and form of the presented content (Nakevska et al., 2017). The degree of interactivity is expected to be directly related to students' attitudes toward learning and learning efficiency (Singh et al., 2020).
Additionally, cost effectiveness is an important aspect when evaluating students' relationship with digital learning platforms. The main benefits derived from this practice are: flexible access to content (Clarke & Hermens, 2001), availability of research electronically (Yang & Cornelius, 2004), and savings in the cost of traveling to the institution (Alkinani, 2021). These benefits have led to positive relationships between students and online learning (Yang & Cornelius, 2004; Singh et al., 2020).
In another note, four studies have aimed to determine what is the greatest influence on faculty decision-making concerning the acceptability of technology for instruction in an environment as controversial as the experienced in COVID-19. Şahin et al. (2021) found that perceived ease of use and perceived usefulness were not determinants of the faculty’s intent to use. Another finding that could be explained by the mandatory adoption of the system is the resistance to opening up to change (intrinsic factors), which has no place in technology adoption. Also, for the lecturers interviewed, self-efficacy in using the technology along with facilitating conditions are great allies in the use of information and communication technologies (ICT).
Nevertheless, in this study, social influence has an impact on the PU but not on the intention to use, which contradicts the results of Sangeeta and Tandon’s study, which states that social influence matters for intention to use and not for atitude (Sangeeta & Tandon, 2020). Still, their results are in line with those of the previous studies that address the relevance of facilitating conditions to the actual use of the remote control system. The study also confirms that anxiety affects the PEOU, but its influence on intention to use is not supported, while Panisoara et al. (2020) found that mental exhaustion along with technology stress was not related to self-efficacy and extrinsic motivation was not related to intention of continuous usage. On the other hand, the study of Hussein et al. (2020) provided important information on qualitative and quantitative aspects, in which they found that financial conditions, technical conditions, i.e., quality of Internet services, and lack of interest and interactivity between instructors and students are relevant factors that inhibit the adoption of public distance education system.
From the point of view of the main constructs of the TAM model, PEOU and PU, not all authors reached the same conclusion. The study by Singh et al. (2020) found that PEOU did not interfere with PU and PEOU did not affect the user’s attitudes. Vladova et al. (2021) also concluded that the hypothesis that presented a positive relationship between the PEOU and the PU was not confirmed. On the other hand, Pal and Patra (2021), who studied the acceptance of video learning, pointed out that the PEOU and PU are directly related to acceptance; just as PEOU and PU are related to the attitude of the user, the PU is related to the actual use of the technology, and the attitude will have an impact on the actual use of the technology. As for PEOU and PU connection to attitude, the study of Vladova et al. (2021) came to the same conclusion.
Another aspect that has been frequently addressed by authors relates to subjective norms, which aim to understand how an individual's opinion might affect the acceptability of a technological agent. In the article by Ho et al. (2020), it was demonstrated that the attitude of users is directly influenced by social factors. In an attempt to understand the factors that influence the acceptance of Youtube as a teaching platform, Yaacob and Saad (2020) found that in addition to the PU and PEOU of a system, the influence of society also has a strong impact on the acceptance of technological means. Nevertheless, these findings are consistent with those of the article by Cicha et al. (2021), who concluded that PU are not influenced by subjective norms. Moreover, the authors believe that this work is due to the mandatory use of remote systems to continue teaching.
Thus, the core question of the project is: what factors contribute to the acceptance of the distance education system for higher education students? With this in mind, and in light of the great impact that isolation can have on the students' academic lives, the objective of this article is to identify the factors that influence the acceptance of technological tools from the perspective of students in the Department of Economics at a Brazilian public university during the pandemic.
MATERIAL AND METHODS
This study has an explanatory character with a quantitative approach, in which a bibliographic survey has been conducted for the last five years in the database: Web of Science, using the strings: "technology acceptance" OR "TAM" AND "covid" OR "pandemic" AND "online teaching" OR "learning" OR "education". The result of the search was eighteen articles to serve as theoretical basis.
The second phase of the research used a quiz originally from Singh et al. (2020), with some adjustments made to adapt it to the purpose of the current study. The quiz contained two blocks to determine the demographic profile of the respondents and the existing physical structure. For the development of the quiz, the perspectives of the Technology Acceptance Model (TAM) were used, a model that is widely used to assess the motivations that lead a person to adopt a particular technology, either in the school or work environment. The quiz consisted of six constructs and twenty-six variables, using the five-point Likert scale.
The quiz was divided into three main parts, the first part consisting of the profile of respondents, the second part asking what structure the students had while using distance education, and the third part was based on the TAM model, by means of 6 constructs with 26 variables to find out how these factors could influence on the use of these tools, testing 6 hypotheses, presented below and graphically displayed in Figure 2.
H1: The PU of the technological tool has a positive effect in the students’ attitude relatively to its use.
H2: The PEOU of the technological tool has a positive effect over the Perceived Utility.
H3: The PEOU of the technological tool has a positive effect in the student’s attitude related to its use.
H4: The interactivity provided by the remote teaching platform has a positive relation on the students’ attitude in its use.
H5: The cost-benefit perceived in the use of digital platforms is positively related to the students’ attitude in its use.
H6: Students’ attitude related to the platform of remote teaching is positively related to the intention to continue its use.
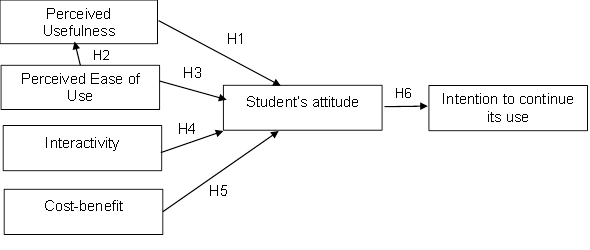
Note. Elaborated with data taken from “Adoption intention and effectiveness of digital collaboration platforms for online learning: the Indian students’ perspective”, by A. Singh, S. Parma, and M. Paliwal, 2020, Interactive Technology and Smart Education, 18(4), pp. 493-514 (https://doi.org/10.1108/ITSE-05-2020-0070).
Figure 2 Hypothetical Theoric Model
The quiz was administered to students of the Business School of the Federal University of Mato Grosso do Sul (UFMS). The population of the survey consisted of twelve hundred and sixty-two (1262) students enrolled in the following courses: Administration with 214 students (full-time and evening students), Tourism with 138, Accounting with 258, Technology in Management Processes with 134, and Finance with 218. The quiz was created remotely using the Microsoft Forms tool and sent by email to the coordinators of the business courses so that they could forward it to the students enrolled in the respective courses. This process resulted in 200 responses, of which 160 were considered valid for the survey.
In addition, descriptive statistics were applied to analyze the collected profile using the software IBM SPSS Statistics, while the structural equations model was implemented to analyze and confirm the hypotheses using the software G Power 3.1.9.2. In order to ensure the validity and reliability of the results, the following tests were performed:
a) Confirmation of sample size feasibility and reliability (verified using G Power 3.1.9.2 software);
b) Analysis of the validity of data convergence (checked by the value of the Average Explained Variance - AVE);
c) Reliability analysis of the constructs measured by the questionnaire (checked by the values of Alfa of Cronbach and Composite Reliability);
d) Calculation of Pearson's Coefficient (R²); and
e) Test t (calculated to make a comparison between the original values and the values simulated by bootstrapping).
RESULTS
Descriptive statistics were applied to analyse the collected profile using the software IBM SPSS Statistics. The public consisted of 94 individuals of female gender (58.8%), 61 individuals of male gender (38.1%), 4 individuals classified as LGBTQIA (2.5%), and one individual who preferred not to respond (0.6%). In terms of age, the greatest engagement is in the 17 to 20-year-old age group with 59 respondents (36.9%), followed by the 21 to 25-year-old age group with 55 respondents (34.4%). The average is 28.4 years and the statistical median is 22 years.
The survey was conducted among students of the School of Managing and Business of the Federal University of Mato Grosso do Sul, composed of courses in Administration, Accounting, Finance, Technology in Management Processes and Tourism. The statistical analysis showed that 74 of the respondents were enrolled in the Accounting programme (46.3%), 41 in the Administration programme (25.6%), 21 in the Tourism programme (13.1%), 16 in the Financial Sciences programme (10%), and finally 8 in the Technology in Management Processes programme (5%).
Regarding the physical structure available for the students to carry out their distance activities, the analysis shows that most of the respondents mainly used computers to access classes. This represents 38.1% of the respondents, followed by partial use of computers and cell phones with 36.9%, as shown in Table 1.
Table 1 Which structures do you use to access the internet to attend the remote classes?
Frequency | Percentage | Accumulated Percentage | |
---|---|---|---|
Only by cell phone | 6 | 3.8 | 3.8 |
Mainly by cell phone | 13 | 8.1 | 11.9 |
Half by cell phone and half by computer | 59 | 36.9 | 48.8 |
Mainly by computer | 61 | 38.1 | 86.9 |
Only by computer | 21 | 13.1 | 100 |
Total | 160 | 100 |
Note. Elaborated with data taken from survey data
As for access to the Internet, most of the respondents had a connection via WLAN (68.1%) and broadband (25.6%). Meanwhile, it was found that the place students use for their academic activities is predominantly their home (60%), followed by mostly their home (21.9%). This could indicate that students had adequate structures available at their homes to continue their distance learning activities (see Table 2).
Table 2 From which site do you access the remote classes?
Frequency | Percentage | Accumulated Percentage | |
---|---|---|---|
Only at home | 96 | 60 | 60 |
Mainly at work | 1 | 0,60 | 60.6 |
Mainly at home | 35 | 21.9 | 82.5 |
Half at work half at home | 28 | 17.5 | 100 |
Total | 160 | 100 |
Note. Elaborated with data taken from survey data
The purpose of this survey’s item was to determine the students' self-perception of their performance in distance education. The result of the survey shows that most of the respondents thought that their performance during this period was “good” (41.3%), followed by the option “normal” (38.8%) while 10% rated their performance as “great” (Table 3). The statistical median for this item was 4, indicating a good rating. This means that at least half (50%) of the respondents rated their performance as good or great, while the other half were distributed among the terrible, poor, or normal options.
Table 3 How was my performance in the couses taken, in general form?
Frequency | Percentage | Accumulated Percentage | |
---|---|---|---|
1-Terrible | 5 | 3.1 | 3.1 |
2-Poor | 11 | 6.9 | 10 |
3-Normal | 62 | 38.8 | 48.8 |
4-Good | 66 | 41.3 | 90 |
5-Great | 16 | 10 | 100 |
Total | 160 | 100 |
Note. Elaborated with data taken from survey data
In order to analyze and confirm the hypotheses, the structural equations model was implemented, using the G Power 3.1.9.2 software to first check the lowest value of the sample to confirm its viability and reliability. It was found that the value of 129 is the least acceptable, and the original sample obtained with the research was 200, yet it was necessary to eliminate 40 answers for reason of incompleteness, thus reaching the number of 160 valid responses. Since the valid number of the obtained sample is too close to the lowest acceptable number, the boostraping technique, also known as resampling, developed by Efron and Tibshirani (1993) was applied to make the sample feasible and reliable. This technique consists in simulating new databases starting from the original sample using a computational algorithm to perform the comparison with the original one. This procedure has been performed 500 times to allow a higher statistical relevance when used in hypothesis tests according to Pillar (1999).
After making adjustments to the test, the viability of the data obtained from the survey, the structural equations model was used to verify whether or not the initially proposed hypotheses were rejected. First, the convergent validity was checked by the value of the average explained variance (AVE), with values above 0.50 considered appropriate (Henseler et al., 2009). This metric assesses the presence of positive correlations between constructs or variables. A value greater than 0.50 means that the model provides satisfactory results (Fornell & Larcker, 1981). All 6 constructs assessed had values higher than the reference value (see Table 4).
Table 4 Values achieved with the Structural Equations Model
AVE | Composite Reliability | R2 | Alfa of Cronbach | |
---|---|---|---|---|
Attitude of the students | 0.579795 | 0.841621 | 0.508328 | 0.744374 |
Intention to Continue the Use | 0.729145 | 0.93074 | 0.472923 | 0.906636 |
Cost-benefit | 0.670046 | 0.890113 | 0.837909 | |
Interactivity of the Students | 0.533851 | 0.820364 | 0.708089 | |
Perception of Use Facility | 0.674973 | 0.892366 | 0.838918 | |
Perception of Utility | 0.594131 | 0.879365 | 0.455114 | 0.828567 |
Note. Elaborated with data taken from survey data
Subsequently, the factors of reliability composition (Composite Reliability - CR) and the values of confidence (Alfa of Cronbach- AC) were analysed. These indicators are used to check whether the sample has biases that could affect the reliability of the survey (Ringle et al., 2014). Values above 0.70 for AC and for CR are considered appropriate (Hair et al., 2014). Again, each construct examined was found to be appropriate (see Table 4).
In the third part of the analysis, the Pearson coefficient (R2) was calculated to check the quality of the fitted model. For the social and behavioural domain, R2 = 2% is considered a low effect, R2 =13% a medium effect, and R2 = 26% a high effect (Cohen, 1988). As well as the other indicators, the 6 constructs also have a significant effect, which proves the model’s suitability (see Table 4).
After analyzing the correlations of the constructs, the tests of the proposed hypotheses were effectively preformed. Due to the application of the resampling technique, the test was designed to make a comparison between the original values and those simulated by bootstrapping. The hypotheses with values above 1.96 indicate that the hypothesis does not need to be rejected, while the values below this value indicate that the hypothesis needs to be rejected (see Table 5).
Table 5 Test of the proposed hypotheses
Hypotheses | B | Value t | Situation |
---|---|---|---|
H1 | 0.146 | 1.537 | Rejected |
H2 | 0.675 | 15.875 | Unrejected |
H3 | -0.022 | 0.246 | Rejected |
H4 | 0.276 | 3.787 | Unrejected |
H5 | 0.405 | 3.407 | Unrejected |
H6 | 0.688 | 16.318 | Unrejected |
Note. Elaborated with data taken from survey data
Values above 1.96 indicate a p value less than or equal to 0.05, i.e., a confidence index of 95% and a margin of error of 5%. All 6 hypotheses were tested simultaneously using the G Power program. Figure 3 shows the correlation between the constructs and the values indicated in the arrows.
The results in Table 5 show that hypotheses H1 and H3 were rejected, i.e., PU and PEOU have no significant effect on students' attitude in using a digital teaching platform. It is not consistent with the result of the article by Singh et al. (2020) and H3 leads to the same result. Moreover, a positive relationship was found between the PEOU of a platform and its PU, a result that differs from the article of Singh et al., (2020) but confirms the model proposed by Davis et al. (1989).
Hypotheses H4 and H5, which stated that interactivity in the use of digital platforms and cost advantage were positively related to students' attitudes toward the use of these technological tools, were considered unrejected. Student attitudes were found to be positively related to student intention to continue using digital platforms, confirming H6. These 3 hypotheses are consistent with the findings of Singh et al. (2020).
DISCUSSION
The context of the problem leads to the need to distinguish the factors that influence student acceptance of digital instruction platforms in order to enable the development of more assertive online systems, and thus be able to deliver distance learning with greater effectiveness. Therefore, the proposal of this article was to review the factors that influence the acceptance of technological resources by students in a business school. The results of the quiz revealed that the factors that have a greater influence on the acceptance of a technological resource by students are the interactivity offered by the system and the cost advantage that benefit users.
The hypotheses tested in this work were based on the model TAM (Davis et al., 1989), and of these, only 2 were rejected by the applied model, which tested whether the PEOU and the PU of a system could have a positive effect on students' attitude. Other hypotheses that stated that the interactivity of a system and its cost advantage positively affect students' attitudes were not rejected, nor was the hypothesis that tested whether students' attitudes affect their intention to continue using technological tools for their studies.
Therefore, from the obtained results, it can be deduced that the factors that most influence a student's decision to use a technological tool are the interactivity and the cost advantage that a digital platform offers to students. This information gathered from the research, could be significant for instructors when planning classes, because if they know the relevant factors from the students' perspective, they can create a more attractive teaching program and consequently have a greater tendency to retain students atention. Understanding that students consider interaction in class as an important factor, it is possible to plan a lesson that makes the student a subject that participates more actively in the activities, rather than being a simple spectator that allows instructors to design activities that are focused on this goal. Nevertheless, it is necessary to choose tools that are easily accessible to students, and it is important to consider the cost benefit to ensure the effective implementation of the system. In such manner, developing a technological system and an academic timetable with these features suggests that students are more likely to continue using them, even in a panorama where there is no mandatory remote system.
Since the PEOU and the PU are not directly related to adoption, the rejection of these two hypotheses is a different result from what the original model proposed by Davis et al. (1989) yielded. From this, one might infer that respondent do not expect a regress to be free of effort in deciding whether or not to accept it. Respondents do not need to expect the adoption of a technology to contribute anything positive to their studies. This result could be due to the fact that the quiz was conducted in a scenario where distance education was the only possible option to continue teaching, i.e., it was an unavoidable system at that time.
The research also showed that respondents at home had the least prerequisites for using digital teaching platforms, as more than 80% of respondents accessed the system from home or mainly from home.
The methodology used was quantitative in nature and consisted of a quiz administered to students of a public university in midwest region in Brazil that offers undergraduate courses in business. A limiting factor was the application of the survey to only one of the groups that made up the teaching system, as it is relevant to understand what influences the acceptance of technological resources by other participants such as lecturers and postgraduates.
Therefore, it is recommended that the survey be applied to senior faculty in order to determine the difficulties and expectations this group has when it comes to using technological resources in their teaching. By collecting data from different areas, it will be possible to influence more effectively the design of a distance education system. As a relevant research agenda, we can answer some questions, such as what kind of active methods can be used in remote teaching in order to increase students' interactivity and, consequently, increase their acceptance and use? What kind of lessons have professors learned from COVID-19 by adapting technological use to face-to-face teaching, or if there is a gap between what they have learned and what they practice, going back at a time without COVID-19? Considering that COVID-19 provided a teaching method without physical barriers, how is it possible to make teaching meaningful for students from different countries, subsidized in a better cost-benefit, and expanding internationalization and multiculturalism in the university environment? These are questions that, when answered scientifically, greatly impact the way society can benefit from the experiences acquired through facing crises, such as COVID-19.